0 Introduction
With the development of demand response (DR)technology and the enhancement of environmental awareness,the formulation of reasonable policies to limit the pollutant emissions of microgrids and the dispatch of power supplies under these policies have become core issues when microgrids participate in the electricity market as power sales companies.A reasonable day-ahead scheduling strategy can decrease the cost of microgrid operations and reduce pollutant emissions.
Currently,the optimization problem of a microgrid’s day-ahead scheduling is mainly based on establishing optimization models according to different optimization objectives and solving the models using different algorithms[1-6].Reference [7] used the particle swarm optimization(PSO) algorithm to obtain scheduling results with the goal of reducing the carbon emissions and operating costs of microgrids.Reference [8] considered the factors of greenhouse gas emissions and system reliability,and proposed a stochastic operation scheduling scheme to reduce the fuel cost and greenhouse gas emissions of power generation.Reference [9] proposed a real-time energy management strategy based on a style="font-size: 1em; text-align: justify; text-indent: 2em; line-height: 1.8em; margin: 0.5em 0em;">Demand response has received increasing attention in recent years [12-14].Demand-side regulation through direct load control (DLC) [15] can reduce the load pressure on microgrids,reduce pollutant emissions,and improve the local environment.Reference [16] proposed an energy management model for microgrids that considered the DR and economic dispatch of energy storage to improve renewable energy utilization and reduce system cost.Reference [17] proposed a model predictive control strategy in conjunction with DR to manage the energy resources of residential microgrids to improve their economic efficiency and reduce power consumption.In [18],DR was used to improve the economics of microgrid operations and reduce the peak load.References [19-23] considered the role of DR in enhancing the economics of microgrids.However,these studies did not consider pollutant emissions,develop relevant strategies,or clarify the relationship between microgrid emissions and DR.
The operation of a microgrid is usually optimized to minimize operating costs or maximize the profitability of the microgrid operator [24-26].Reference [27] minimized the total operating costs by scheduling multiple microgrids to fully utilize the generating capacity of the power system.Reference [28] designed a microgrid containing multiple energy storage systems and proposed a distributed economic dispatch strategy to minimize microgrid operating costs.A real-time scheduling method based on a twostage correction strategy for the economic operation of a microgrid to ensure the economy of the microgrid day-ahead schedule was proposed in [29].Although the optimization operation of the above references considers the microgrid economics,it does not consider the environmental impact of pollutants caused by nonclean power supplies in microgrids at different time periods,nor does it analyze the relationship between the curtailment of the demand-side load and pollutant emissions.The relationship between the loads and microturbine emissions is shown in Fig.1.When the microgrid utilizes the microturbine to generate electricity for power balance,the micro turbine inhales air from the outside atmosphere through the pressurizer.The compressed air is pressed and sent to the heat boiler to mix with the injected fuel to generate high-temperature and high-pressure steam,CO2,SO2,and other exhaust gases.
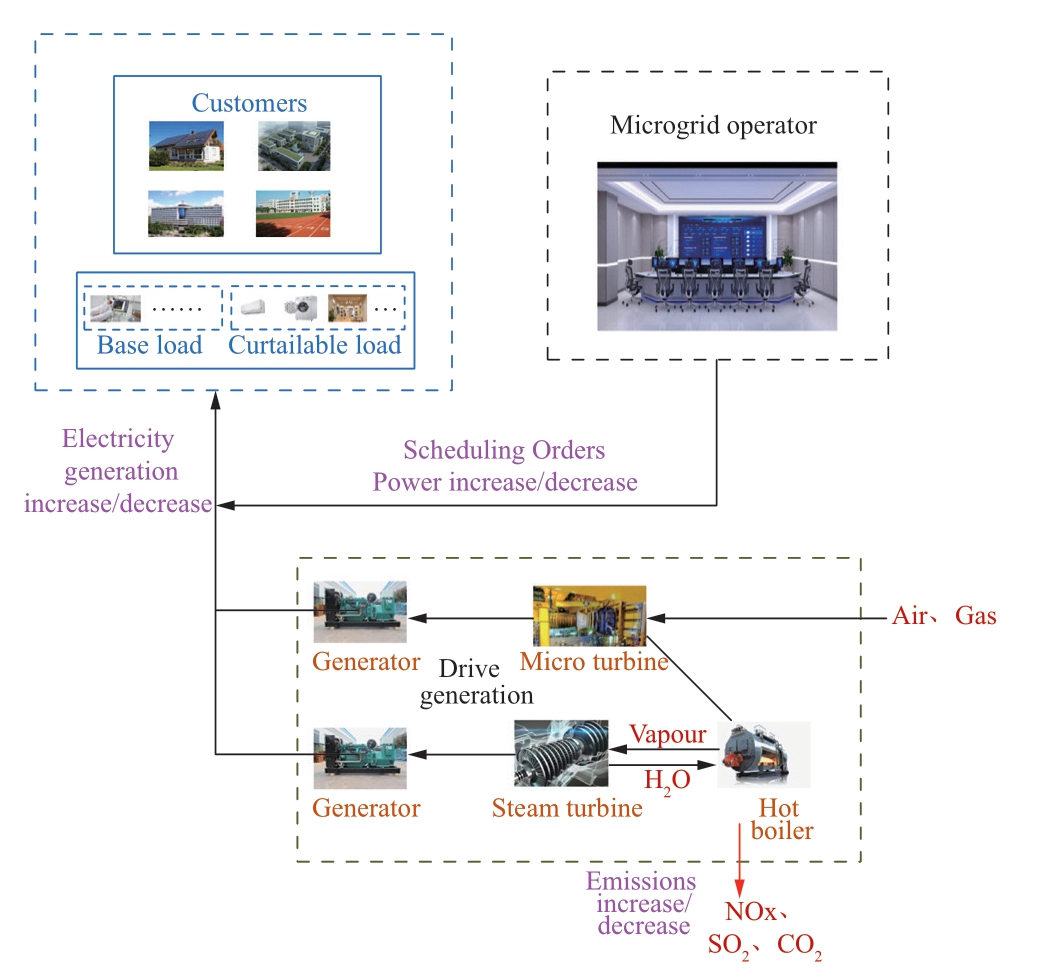
Fig.1 Relationship between loads and microturbine emissions
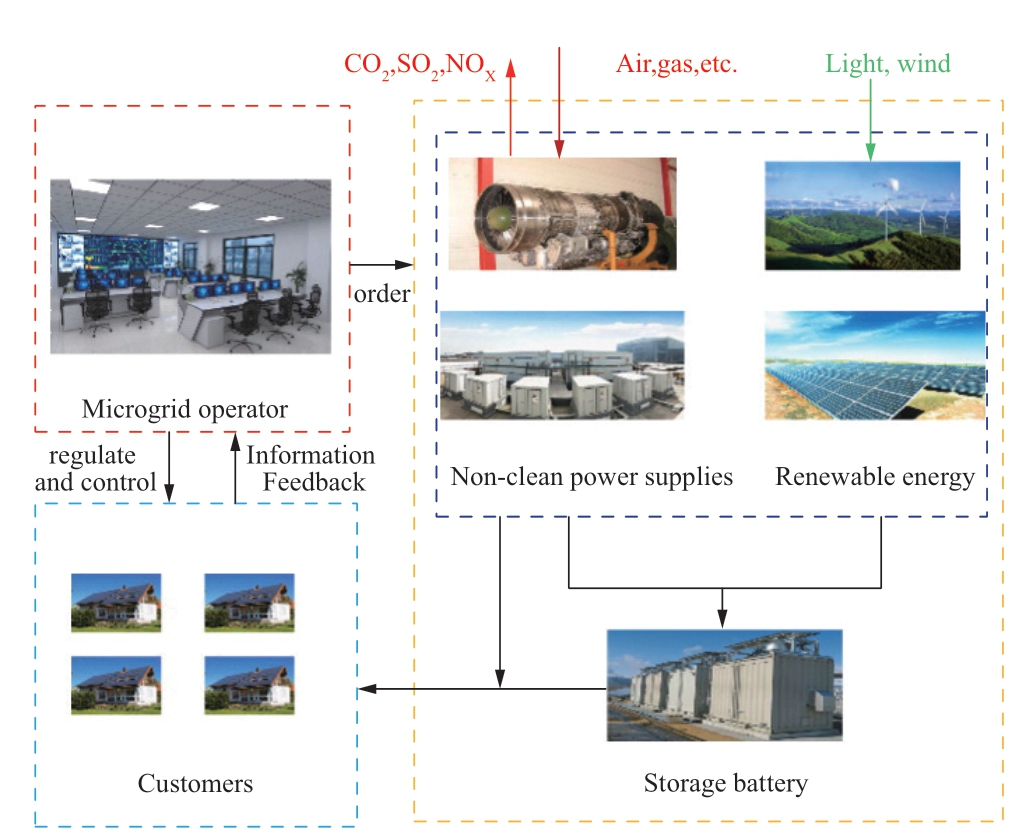
Fig.2 Structure of the microgrid
According to the above analysis,to assess the degree of pollution of microgrids in the environment and reduce pollutant emissions from microgrids,this study proposes an optimal day-ahead scheduling strategy considering pollutant emissions and the curtailment potential of curtailable loads in each time period.First,this paper defines a regional pollution index (RPI) and customer compensation mechanism based on the RPI.Second,a curtailable load control strategy based on DLC is established.Third,a microgrid optimization model that considers the RPI and the curtailment potential of curtailable loads is proposed.
The main contributions of this study are as follows:
1) This study proposes an RPI to assess the degree of regional pollution caused by nonclean power generation in each period.On this basis,an RPI-based penalty mechanism is proposed for microgrid operators to reduce the use of nonclean power supplies in microgrids by curtailing the load through a demand response.
2) This study proposes a day-ahead scheduling strategy for a microgrid that considers the RPI and curtailment potential of a curtailable load,and it is to enable the microgrid to obtain a stronger ability to transmit power to the grid,and it can further reduce pollutant emissions during microgrid operation through direct load control.
The remainder of the paper is organized as follows:Section 1 describes the composition of the microgrid,proposes an RPI-based penalization mechanism for microgrid operators,and a DLC-based compensation mechanism for customers.Section 2 proposes a microgrid day-ahead scheduling strategy that considers the RPI and curtailment potential of curtailable load.Section 3 summarizes the study by analyzing case studies.
1 Customer compensation mechanism based on RPI and curtailment potential of curtailable load
This section models the microgrid power supplies,defines the RPI,and proposes a microgrid penalty mechanism based on the RPI and customer compensation mechanisms based on the curtailment potential of the curtailable load.
A microgrid is a small power system that contains a set of localized power supplies and has two operating modes:islanded and grid-connected.In an islanded operation,the microgrid does not interact with the grid,whereas in a grid-connected operation,the microgrid interacts with the grid through a point of common coupling (PCC),and the microgrid can switch from one mode to another.The microgrid adjusts the output power of each device through its central coordination controller to generate an optimal scheduling scheme that satisfies the load demand.
1.1 Power supplies in microgrid
The power supplies included in the microgrid in this study are distributed renewable energy sources,fuel cell(FC),micro turbine (MT) and storage batteries (BA).Among these,MT includes hydrogen gas turbines,which have higher efficiencies and lower pollutant emissions than conventional MT.
1) Wind turbine generating model
The wind turbine (WT) output power is related to wind speed and is expressed as follows:
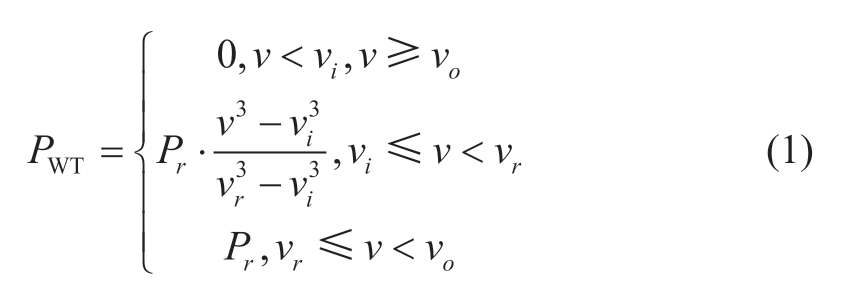
where PWT is the output power of the WT;v is the realtime wind speed;vi is the cut-in wind speed;vr is the rated wind speed;vo is the cut-out wind speed;and Pr is the rated power.
2) Photovoltaic generating model
Photovoltaic (PV) output power is related to the climate:

where,PPV represents the output power of PV;PSTC represents the output power of PV under the standard condition;GA represents the real-time light intensity;GSTC represents the light intensity under the standard condition,with a value of 1000 W/m2;k represents the temperature coefficient, Tp_c represents the working temperature of the equipment;and Tr represents the standard ambient temperature 25 °C.
3) Fuel cell cost model
The cost of generating electricity from fuel cell is as follows:

where CFC_FC denotes the fuel cost of fuel cell; CFC denotes the market price of gas;LHVnl denotes the lower calorific value of gas,which is considered to be 9.7 kWh/m3;PFC denotes the output power of fuel cell,and enotes the utilization efficiency.
As fuel cells can produce pollutants,the cost of treating pollutants can be given by

where CEC_FC represents the cost of pollutant treatment for fuel cell;K denotes the number of pollutant types;αk denotes the cost of treatment (p.u.) for pollutant type k; βk,FC denotes the emission factor for pollutant type k for the fuel cell.
4) Microturbine cost model
The cost of generating electricity from microturbine is as follows:
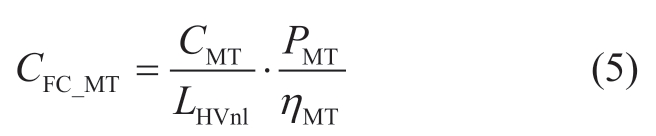
where CFC_MT denotes the fuel cost of the MT;CMT denotes the price of natural gas;PMT denotes the output power of the MT and ηMT denotes the utilization efficiency.
The cost of treating pollutant from microturbine is as follows:

where CEC_MT represents the cost of pollutant treatment (p.u.)for MT;βk,MT denotes the emission factor for pollutant type k for the MT.
5) Storage battery model

where SOCBA,t denotes the state of charge (SOC) of storage battery at time t;SOCBA,t-1 denotes the SOC of storage battery at time t-1;δ is a 0-1 variable,and it is charged when δ is 1,and it is discharged when δ is 0;PBA,t is the output power;ηcha is the charging efficiency;ηdis is the discharging efficiency;BESScap is the capacity of storage battery.
1.2 Microgrid penalty mechanism based on regional pollution index
Among the microgrid power supplies,FC and MT are non-clean power supplies that produce gaseous pollutants such as CO2,NOx,and SO2 while generating electricity.
1.2.1 Regional pollution index
When the curtailable load in the microgrid is completely curtailed and the microgrid is in the islanded mode,the microgrid operation generates pollutants;however,it is unavoidable to generate pollutants to satisfy the electricity demand of microgrid customers.Microgrids operated in the grid-connected mode also generate pollutants;however,in this mode,the microgrid is operated not only to satisfy the electricity demand of customers,but also to participate in power trading.To measure microgrid pollution in a region,this study proposes a regional pollution index using the following expression:
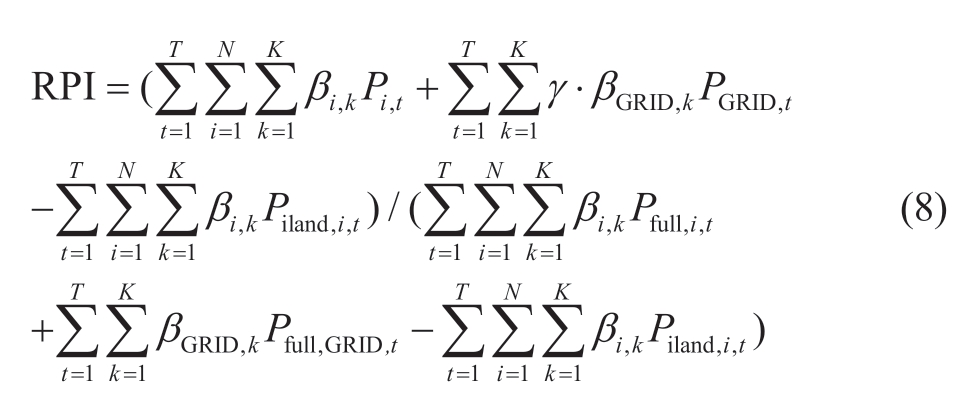
where T is the scheduling period;N is the number of power supply types;K is the number of pollutant types; Pi,t denotes the output power of power supply type i at time t;βi,k denotes the emission factor of pollutant type k for power supply type i;PGRID,t denotes the transmission power from the grid to the microgrid;βGRID,k denotes the emission factor of pollutant type k generated by grid;Piland,i,t denotes the power generation of the i-th power supply at the moment t after the curtailable load of the microgrid in islanded mode have been completely curtailed;and γ is a 0-1 variable;when PGRID,t <0,γ =0,and when PGRID,t ≥ 0,γ =1;Pfull,i,t denotes power generation of the i-th power supply at the moment t when the i-th power supply is fully loaded;and Pfull,GRID,t denotes the maximum power which can be transmitted from the grid to the microgrid at the moment t.
1.2.2 RPI-based penalty mechanism
This study proposes an RPI-based penalty mechanism to reduce regional pollution caused by microgrids and compensate customers.The details are shown in Eq.(9) and Fig.3.
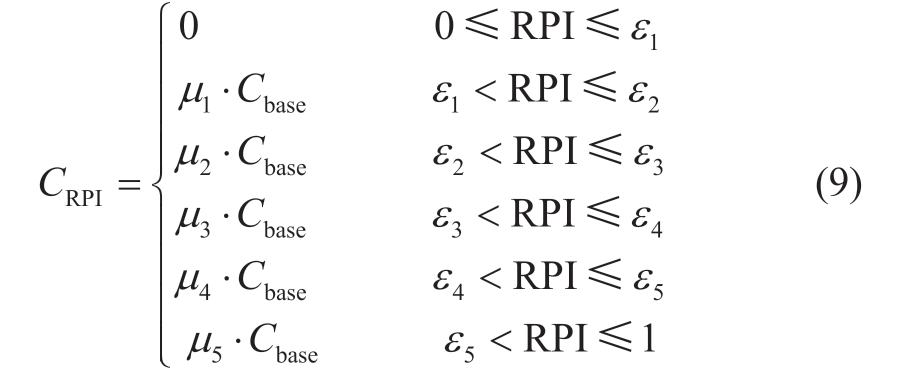
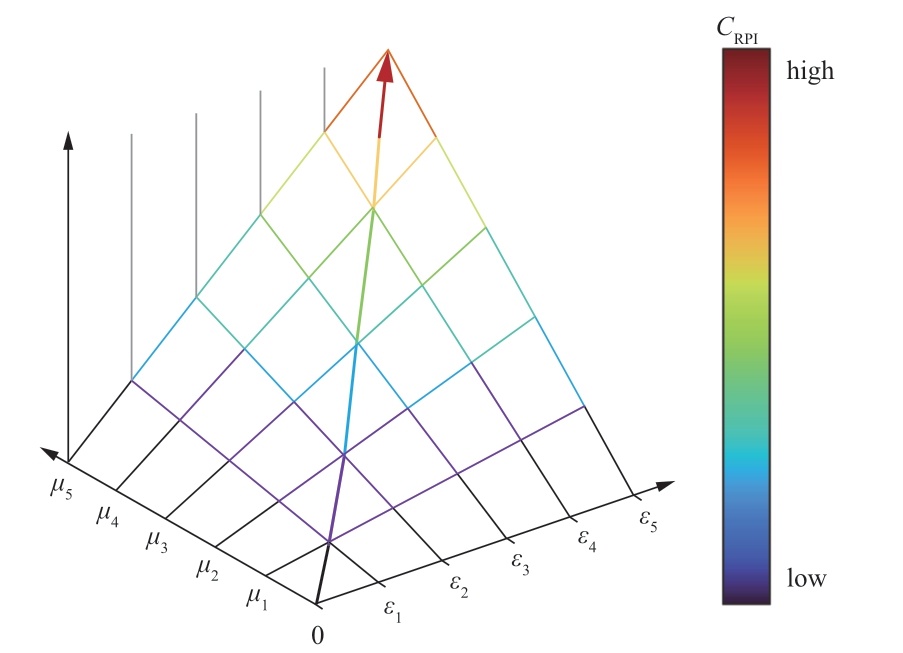
Fig.3 Relationship between CRPI,ε,and μ
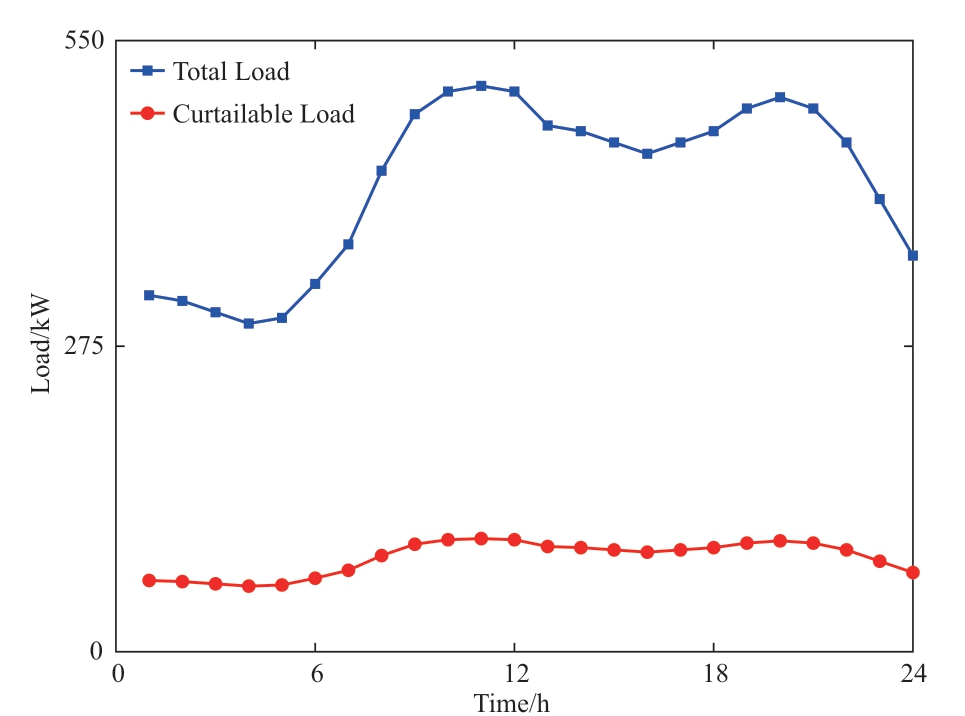
Fig.4 Load curves of a typical day
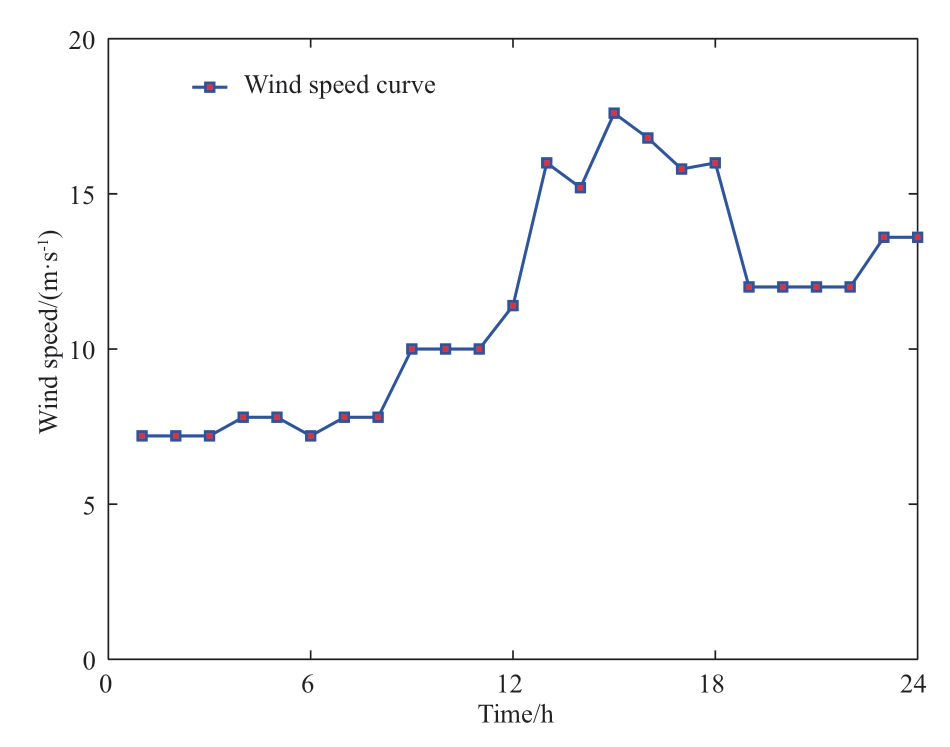
Fig.5 Wind speed curve of a typical day
where CRPI is the penalty tariff;Cbase is the base value of penalty tariff;ε1,ε2,ε3,ε4,ε5 are the division values of RPI;μ1, μ2, μ3, μ4, μ5 are the RPI-based penalty coefficients.
1.2.3 Customer compensation mechanism based on the curtailment potential of curtailable load
This study proposes a customer compensation mechanism based on the curtailment potential of curtailable loads.Customers in microgrids include critical loads,such as communication hubs,and curtailable loads,such as air conditioning and lighting in commercial buildings.The microgrid operator can dynamically adjust or turn off the customer’s electrical equipment to reduce the microgrid load by signing an agreement with the customers who can curtail their loads.Customers who curtail their loads are compensated based on the curtailment amount.The agreed tariff between the microgrid and customer is modeled as
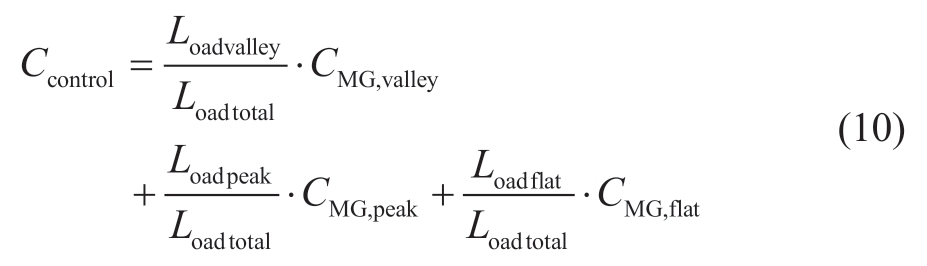
where Loadpeak, Loadflat, Loadvalley, Loadtotal are the amounts of load during the peak,flat,valley,and scheduling periods,respectively; CMG,peak,CMG,flat,CMG,valley are the tariffs of the microgrid during the peak,flat,and valley periods,respectively.
2 Microgrid day-ahead scheduling strategy
In this section,an optimal day-ahead scheduling strategy for a microgrid that considers pollutant emissions and load curtailment is proposed based on the mechanisms proposed in Section 1.The conventional day-ahead scheduling of a microgrid considers the fuel cost of nonclean power supplies in the microgrid,the cost of interactive electricity,the maintenance cost of the power supply,and the cost of pollutant treatment.This section proposes a day-ahead scheduling strategy that incorporates the curtailment potential of the RPI and curtailable load.
2.1 Objective function for day-ahead scheduling of microgrid
2.1.1 Objective function for day-ahead scheduling of microgrid in grid-connected mode
Under the penalty mechanism based on the regional pollution index,the objective function of the microgrid operation in grid-connected mode is as follows:
1) Fuel costs

where CFC,t denotes the fuel cost of the MT at time t;CMC,t denote the fuel costs of the MT and FC,respectively,at time t.
2) Interactive power cost

where Cgridsell,t is the price that the grid sells electricity to the microgrid at moment t;PGRID,t is the power transmitted from the grid to the microgrid at moment t;Cmicrogridsell,t is the price that the microgrid sells electricity to the customers at moment t;Cgridbuy,t is the price that the microgrid sells electricity to the grid at moment t; Pcustomer,t is the amount of electricity consumed by the customers at moment t;and λ is a 0-1 variable,with λ being 1 when PGRID,t <0 and λ being 0 when PGRID,t >0.
3) RPI-based penalty for environmental pollution
The proposed RPI-based penalty mechanism penalizes the microgrid and compensates customers based on the RPI value.

where CRPI,t is the penalty tariff at time t based on RPI.
4) Pollutant treatment cost
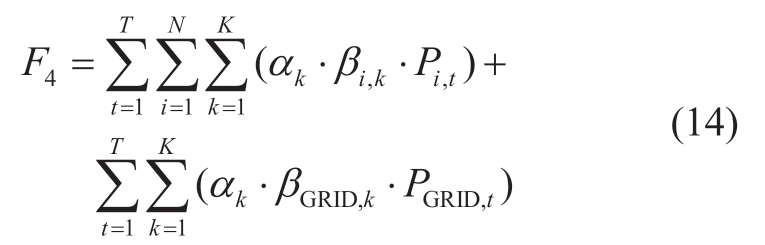
where αk represents the cost of treatment (p.u.) for type k hazardous gas.
5) Compensation based on curtailment potential of curtailable load

where Pcurt,t represents the amount of curtailable load’s curtailment at moment t.
6) Power supply maintenance cost

where KMC,i,t represent the maintenance cost factors for i-th power supply.
The objective function of the proposed day-ahead scheduling strategy is modeled as follows:

2.1.2 Objective function for day-ahead scheduling of microgrid in islanded mode
The microgrid does not interact with the grid in the islanded mode;therefore,the power interaction cost and pollutant treatment cost in the objective function in the islanded mode are different from those in the grid-connected mode.The details are as follows:
1) Interactive power cost

2) Pollutant treatment cost

The objective function for the day-ahead scheduling of the microgrid in the islanded mode is modeled as follows:

2.2 Constraints
2.2.1 Constraints for day-ahead scheduling of microgrid in grid-connected mode
In grid-connected mode,the microgrid operates under the following constraints.
1) Power balance constraint
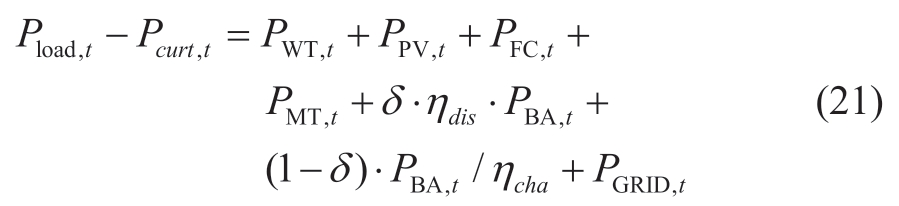
where Pload,t is the total load of the microgrid at moment t;PWT,t is the output power of the WT at moment t;PPV,t represents the output power of the PV at moment t; PFC,t denotes the output power of the FC at moment t;and PMT,t denotes the output power of the MT.
2) Power Output Constraint

where is the minimum output power of the i-th power supply at time t;P
is the maximum output power of the i-th power supply at time t.
3) Battery SOC constraint

where is the minimum SOC value;
is the maximum SOC value;SOCBA,start is the initial SOC value;and SOCBA,final is the final SOC value.
4) Transmission capacity constraint of PCC

where is the minimum power allowed to be transmitted from the microgrid to the grid,and
is the maximum power that can be transmitted from the microgrid to the grid.
5) Load reduction constraint

where is the minimum load value that can be reduced by the microgrid at time t;and
is the maximum load value that can be reduced by the microgrid at time t.
2.2.2 Constraints for day-ahead scheduling of microgrid in islanded mode
When the microgrid operates in the islanded mode,there are no transmission capacity constraints of the PCC,and the power balance constraint is different from that when the microgrid operates in the grid-connected mode.The details are as follows:
1) Power balance constraint
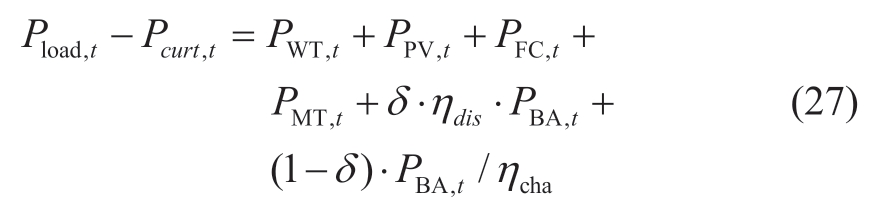
3 Case studies
To illustrate the effectiveness of the optimal day-ahead scheduling strategy proposed in this study,it is necessary to have typical daily load data of the microgrid,prices for buying and selling electricity from the microgrid,and pollutant emission coefficients of the power supplies.In this case, ε1,ε2,ε3,ε4,ε5 are 0.2,0.4,0.6,0.75,0.9,respectively.μ1, μ2, μ3, μ4, μ5 are 0.05,0.1,0.2,0.3,0.5,respectively.The data in this study were obtained from the typical daily load in a certain area.The experiments were implemented on a PC with a Windows 11 operating system,Intel Core i7-8565U,1.80 GHz CPU,and 16 GB RAM.Cbase is 0.41¥/kwh.The peak periods were from 9:00 to 12:00 and 17:00 to 21:00.The flat periods were from to 13:00-16:00 and 22:00.The valley period lasts from 23:00 to 8:00.The detailed data settings are as follows:
Typical daily load data,including the curtailable load and wind speed curve for a typical day,are shown in Figs.4 and 5,respectively.
The prices of buying and selling electricity from microgrid are shown in Table 1.
Table 1 Prices for buying and selling electricity from microgrid
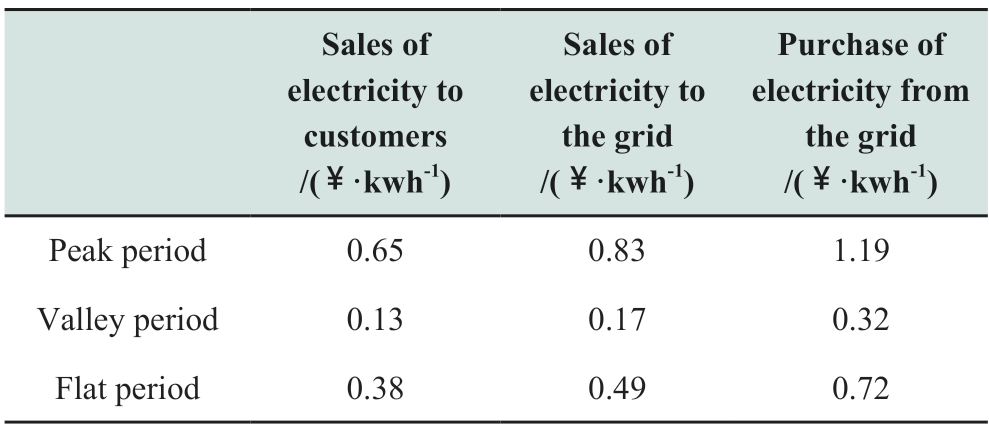
The pollutant emission coefficients for each power supply and the treatment cost (p.u.) for each pollutant are listed in Table 2.The MT used in this study is a hydrogen gas turbine.
Table 2 Emission coefficients and pollutant treatment costs of power supplies
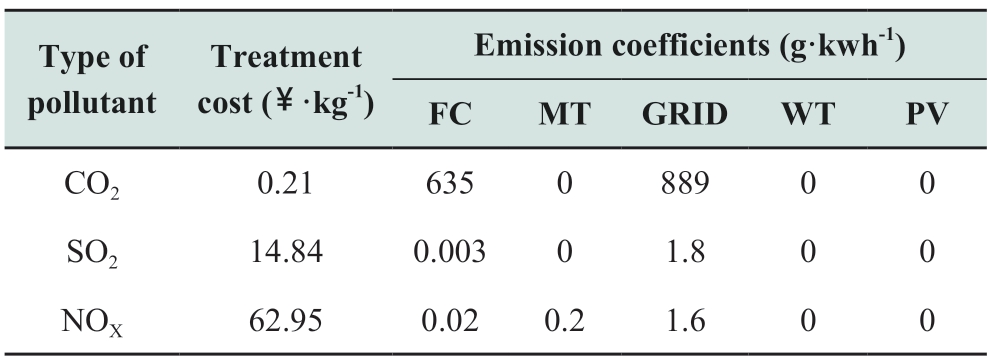
Light intensity of a typical day is shown in Fig.6.
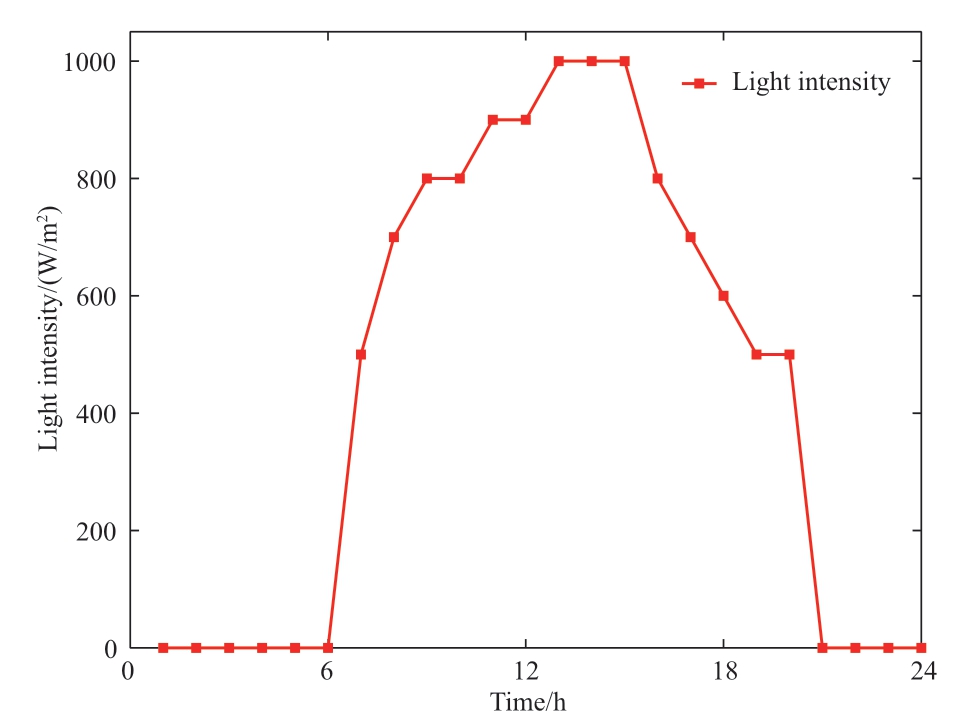
Fig.6 Light intensity of a typical day
The basic parameters of each power supply used in this study are listed in Table 3.
Table 3 Basic parameters of power supplies
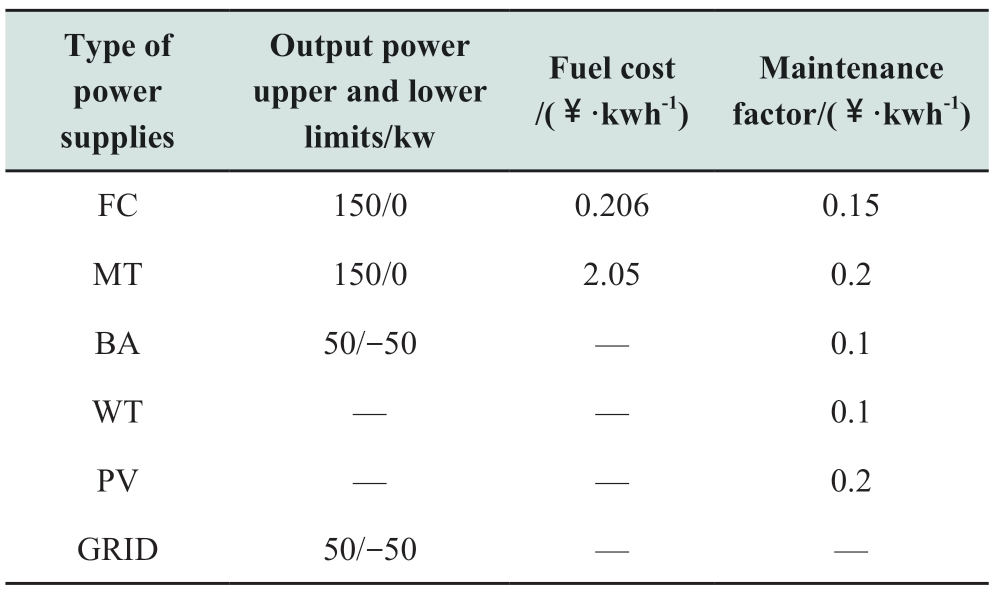
3.1 Scheduling results
The RPI proposed in this study is based on the scheduling results when the microgrid is in islanded mode and the curtailable load is completely curtailed.The scheduling results for the microgrid in the above scenario are presented in Fig.7.
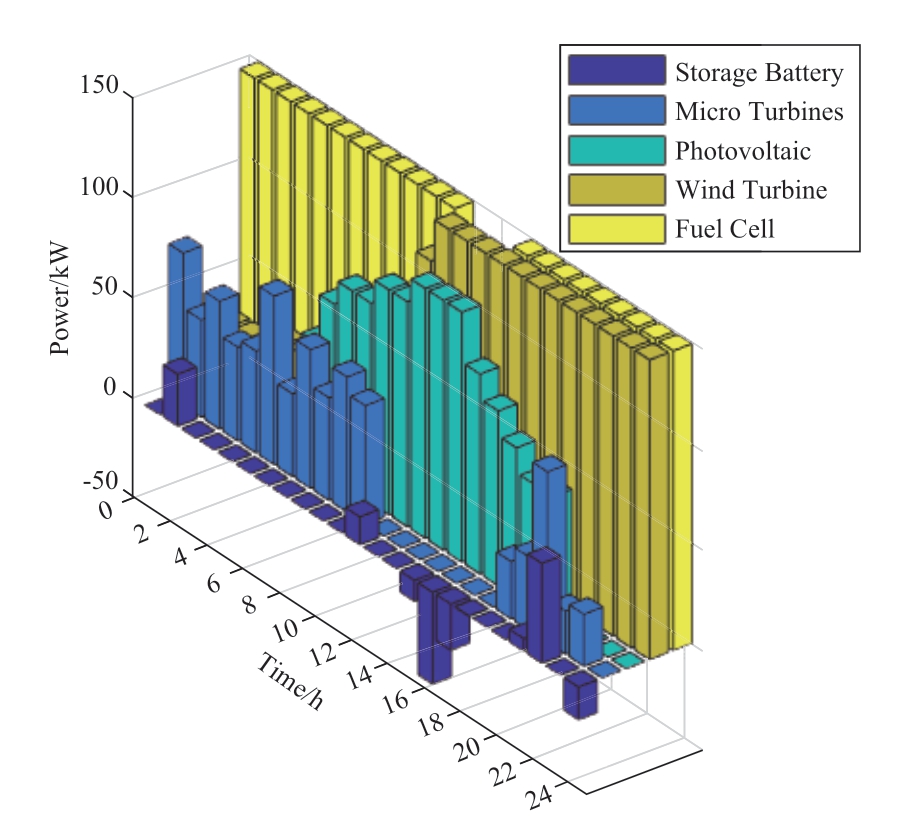
Fig.7 Scheduling result of the microgrid in islanded mode
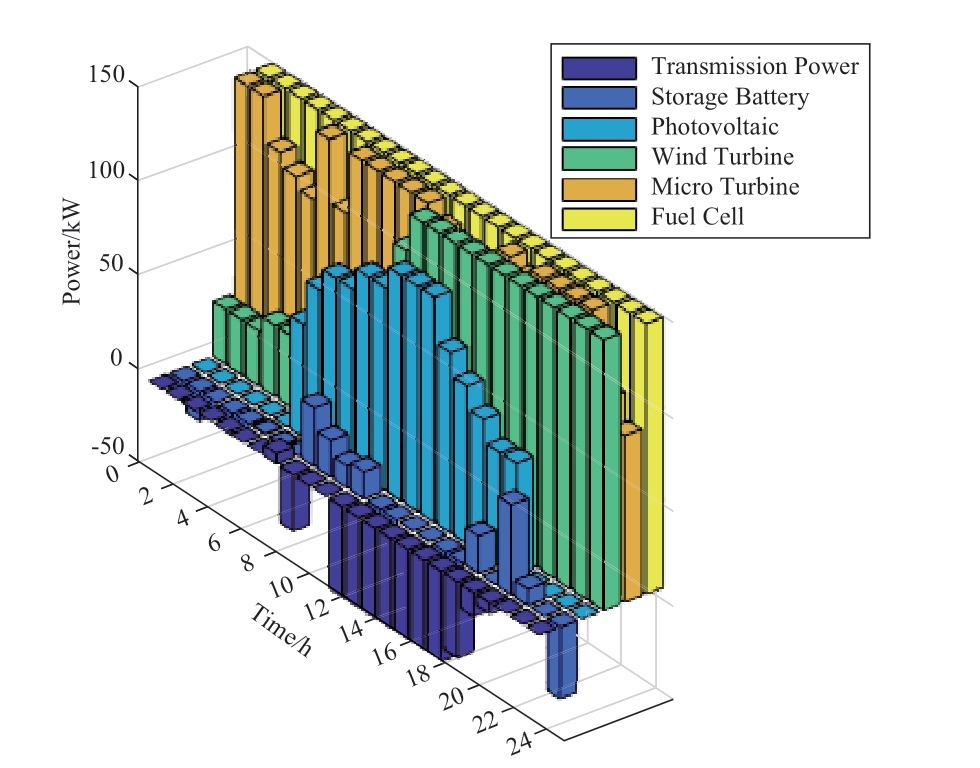
Fig.8 Scheduling results of the conventional scheduling strategy
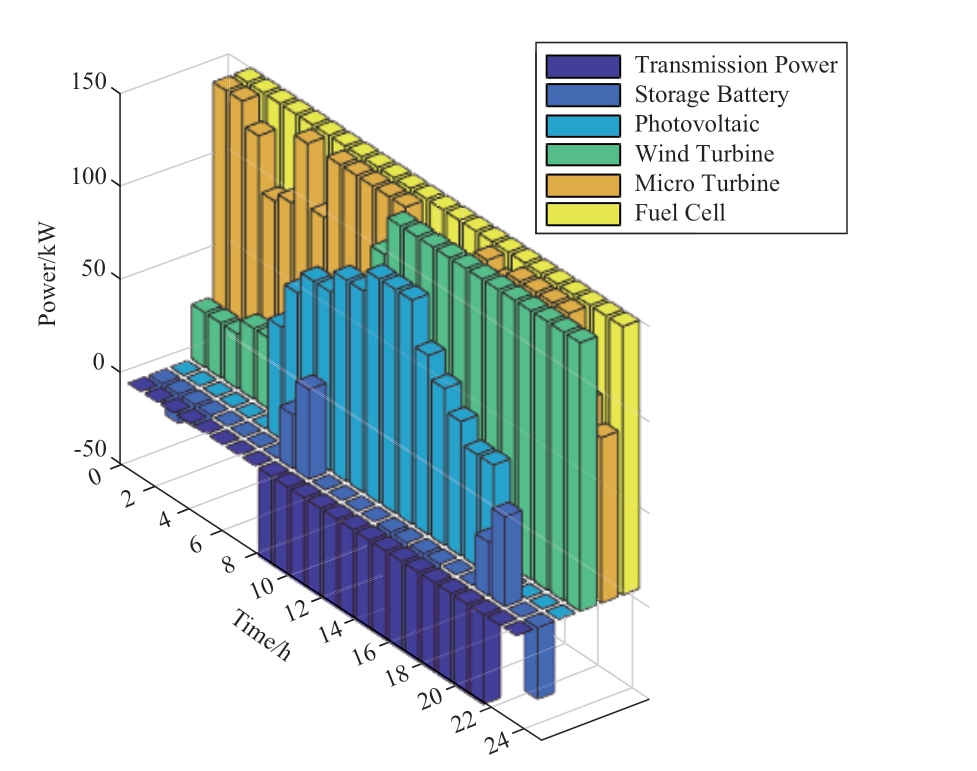
Fig.9 Scheduling results of the scheduling strategy only considering potential load curtailment
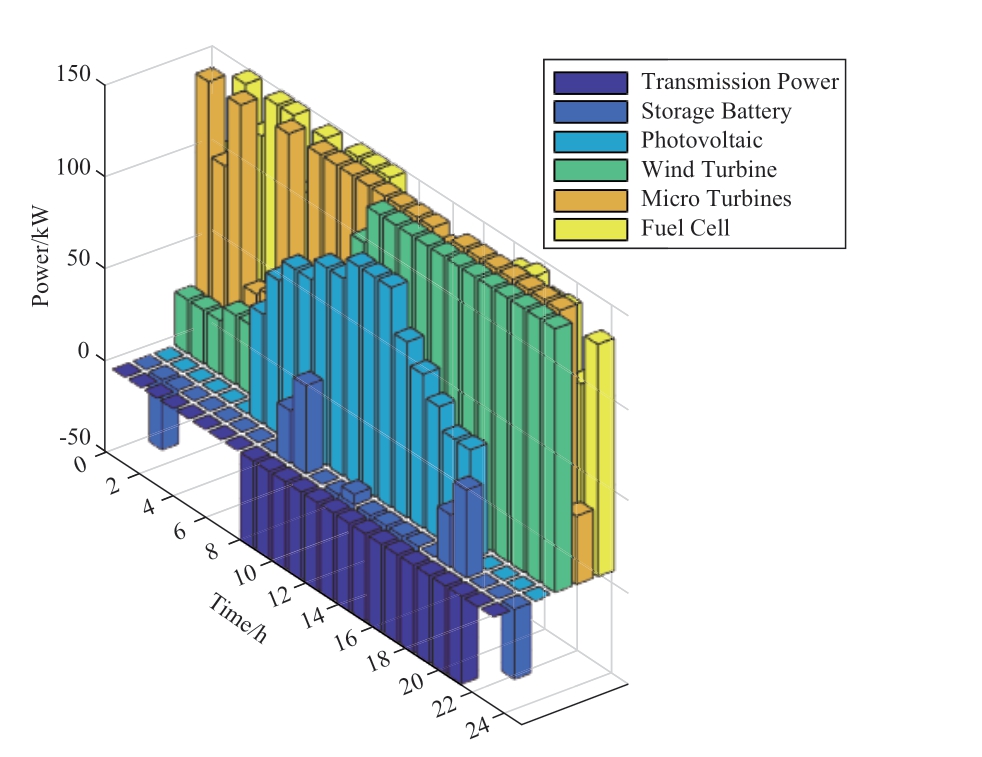
Fig.10 Scheduling results of the scheduling strategy considering the RPI and potential load curtailment
As can be observed in Fig.7,when the curtailable load is completely curtailed and the microgrid is in the islanded mode,to maximize the profitability of the microgrid while satisfying the load demand,the microgrid will prioritize renewable energy to generate electricity because renewable energy sources do not require fuel or produce pollutants,and only require maintenance of units.In this microgrid,the WT is not able to generate the rated output power from 1:00-12:00 due to the low wind speed,and it produces full output from 13:00-24:00 when the wind speed is greater than the rated wind speed and less than the cut-out wind speed.The light intensity was zero from 1:00-5:00,gradually increased to a maximum from 6:00-13:00,and then gradually decreased after 15:00,reaching zero at 21:00.The PV system is affected by the light intensity;therefore,the trend of changes in the power generation of the PV system is the same as that of changes in the light intensity.When the generation of renewable energy sources cannot fulfil the load demand,the microgrid calls on the FC because the fuel cost of the FC is lower than that of the MT.The MT generates electricity when there is a shortage of renewable energy,such as at 1:00-11:00,and 19:00-20:00.The BA discharges at 2:00,12:00,21:00-22:00,in this mode.Here,12:00,21:00 belong to the peak period,and the BA discharges to increase the profitability of the microgrid.The BA discharges at 2:00,22:00 because the output power of the PV is low owing to the low light intensity;the WT and FC cannot satisfy the load demand,and the power generation cost of the MT is high.Therefore,the BA discharges at 2:00,22:00 to minimize the operating cost of the microgrid.The BA is charged during the valley period or when there is no need for the MT to generate power;this is to discharge at the peak period for profit.
Based on the results shown in Fig.7,the denominator of the RPI can be obtained by adopting the conventional scheduling strategy,scheduling strategy considering only the potential load curtailment,and scheduling strategy considering both the RPI and potential load curtailment for the microgrid.The specific scheduling results are shown in Figs.8,9,and 10.
From Figs.8 and 9,it can be observed that the microgrid prioritizes renewable energy to generate electricity because of the high price of purchasing power from the grid and the higher emission factor of pollutants generated by grid generation.When the internal power generation of the microgrid satisfies its own load demand,it sells power to the grid to obtain higher profits.Comparing Figs.8 and 9,it can be noted that in the period 9:00-22:00,the scheduling strategy that only considers potential load curtailment obtains a stronger ability to transmit power to the grid;thus,the microgrid obtains higher profits by curtailing the customers’ loads.
Comparing Figs.8,9,and 10,it can be observed that both the strategy proposed in this study and the strategy that only considers potential load curtailment obtain a stronger ability to transmit power to the grid than the conventional scheduling strategy.However,the scheduling strategy proposed in this study depends more on the MT and reduces the use of the FC compared with the other two strategies.This situation occurs because the scheduling strategy proposed in this study considers the RPI,and when the RPI is high,the penalty mechanism based on the RPI will make the combined cost of the FC power generation higher than that of the MT.
3.2 Comparison of benefits
To illustrate the effectiveness of the proposed scheduling strategy,comparison of benefits under different scheduling strategies is listed in Table 4.In Table 4,Case 1 denotes the conventional scheduling strategy,Case 2 denotes the scheduling strategy that only considers the potential load curtailment,and Case 3 denotes the scheduling strategy that considers the RPI and potential load curtailment.
Table 4 Comparison of benefits under different scheduling strategies
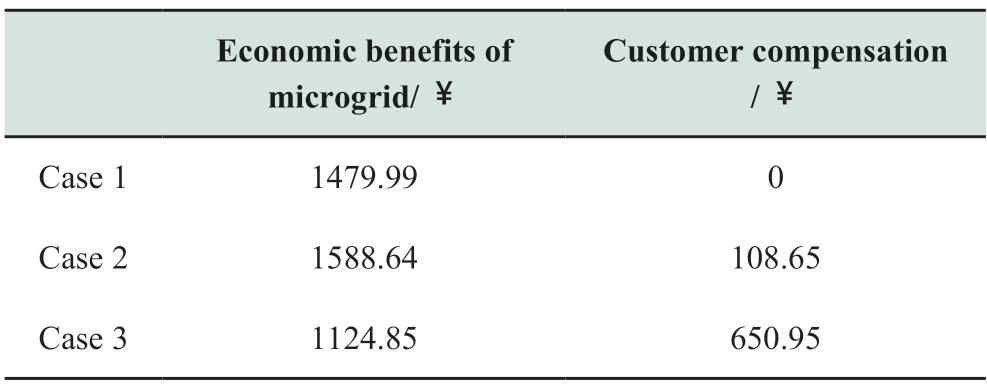
As shown in Table 4,under the strategy that considers only potential load curtailment,the profitability of the microgrid is improved by 7.34% compared with the conventional scheduling strategy.Under the strategy proposed in this paper,the profitability of the microgrid falls by 24% compared to the conventional scheduling strategy,and by 29.2% compared to the strategy that only considers potential load curtailment.Customer compensation consists of two components: compensation based on the amount of load curtailment,and compensation based on the RPI punishment for environmental pollution.Under the strategy proposed in this study,customer compensation is improved by 499.13% compared to the strategy that only considers potential load curtailment.This suggests two points:1) the use of DLC can effectively improve the economic efficiency of microgrids as electricity sales companies;2) the penalty mechanism for microgrid operators based on the RPI reduces the economic efficiency of the microgrid to a certain extent,and customers receive higher compensation compared to the other two strategies.The environmental impact of the mechanism proposed in this study will be further analyzed later.
3.3 Comparison of regional pollution levels
To illustrate the effectiveness of the proposed RPI and RPI-based penalty mechanisms,the RPI of the microgrid under different scheduling strategies has been compared,as shown in Fig.11.
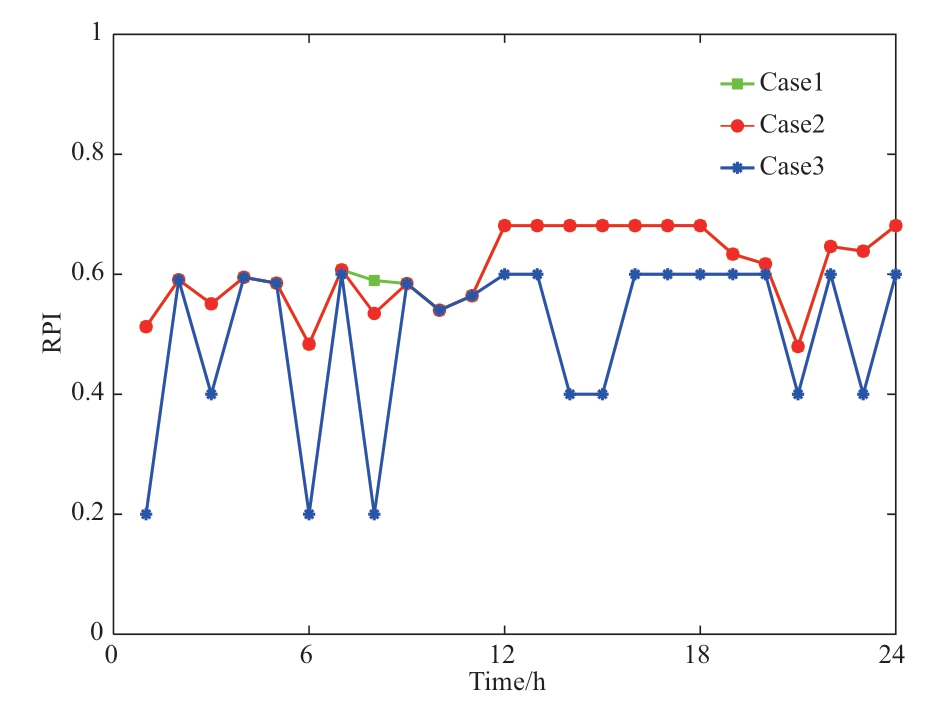
Fig.11 RPI of the microgrid under different scheduling strategies
As shown in Fig.11,the RPI of the scheduling strategy proposed in this study is less than or equal to that of the scheduling strategy that only considers potential load curtailment and the conventional scheduling strategy throughout the day.This indicates that the scheduling strategy that considers the RPI and potential load curtailment has less pollutant emissions than the other strategies.A comparison of the total pollutant emissions under the different strategies is shown in Fig.12.
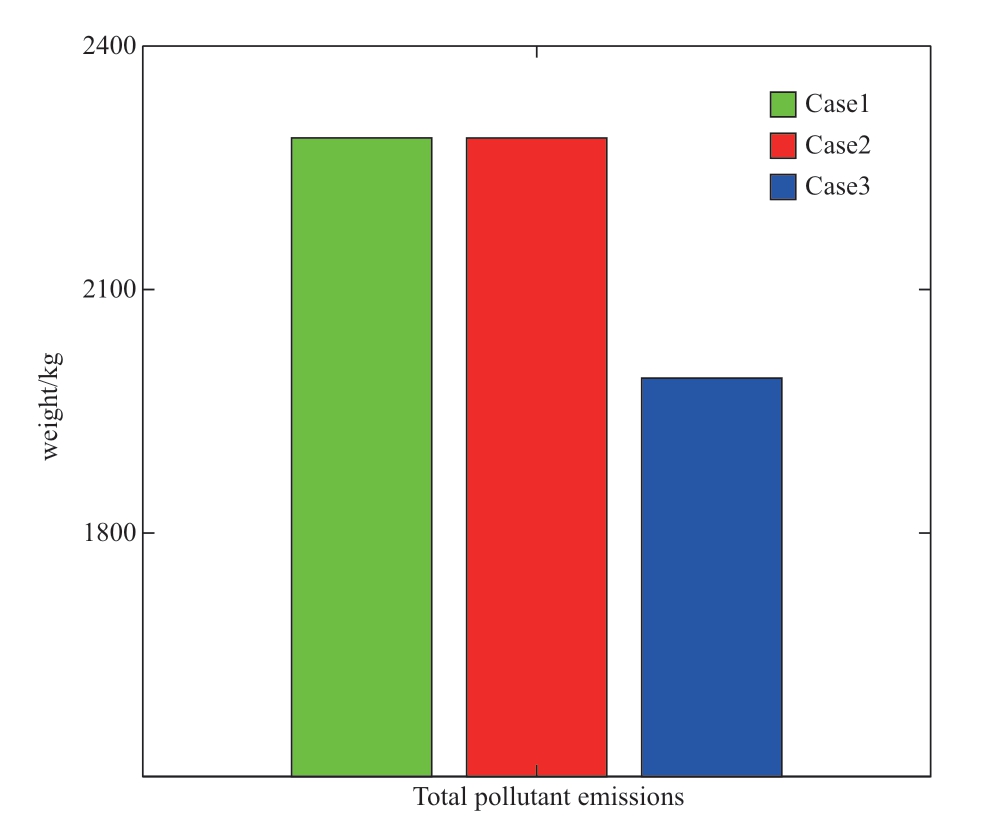
Fig.12 Comparison of total pollutant emissions under the different strategies
From Figs.11 and 12,it can be observed that there is little difference in pollutant emissions between the scheduling strategy only considering potential load curtailment and the conventional scheduling strategy.This is because although the scheduling strategy only considering potential load curtailment curtails the customers’ loads,it transmits more power to the grid to gain more profit.The scheduling strategy proposed in this study also transmits more power to the grid to gain more profit;however,the RPI-based penalty mechanism proposed in this study makes the microgrid making use of MT more effective in reducing pollutant emissions.Pollutant emissions were reduced by 12.94% for the scheduling strategy proposed in this study compared to the conventional scheduling strategy and by 12.93% when compared to the scheduling strategy that only considers potential load curtailment.This suggests that the RPI-based penalty mechanism proposed in this study can effectively reduce the pollutants emitted during microgrid operation.
3.4 Comparison of customer loads curves
The impacts of the scheduling strategy proposed in this study,which only considers potential load curtailment,and the conventional scheduling strategy on customer loads are shown in Fig.13.
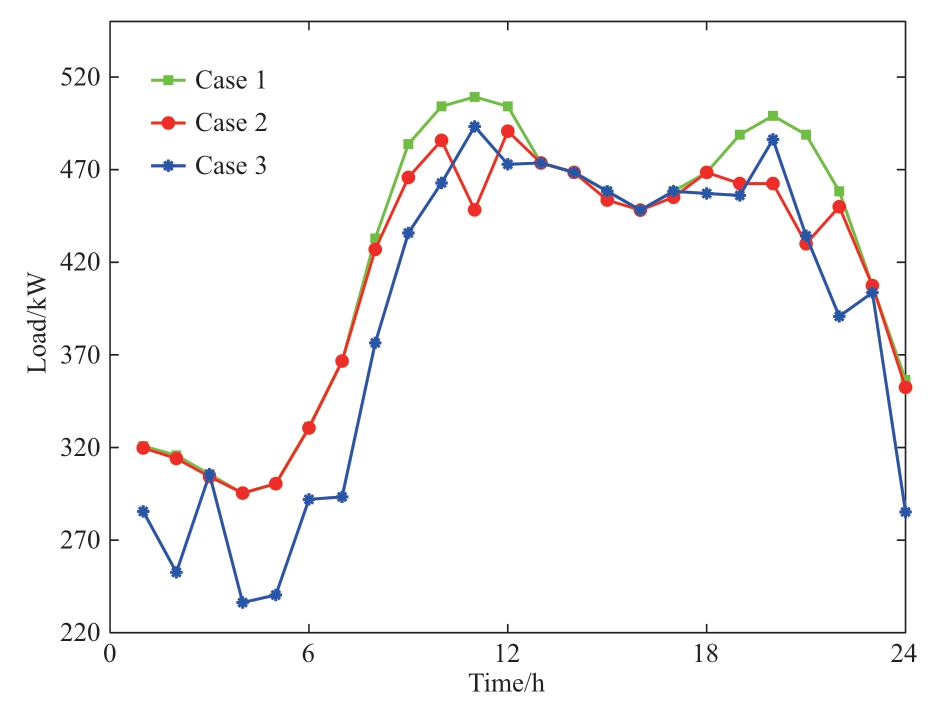
Fig.13 The impact of different scheduling strategies on customer loads
As shown in Fig.13,the scheduling strategy that only considers potential load curtailment curtails the customer loads from 9:00-12:00 because renewable energy generation is insufficient during this time,and the microgrid operator curtails the customer loads to gain the ability to transmit more power to the grid to obtain more profit.The scheduling strategy that only considers the potential load curtailment does not curtail the customer loads from 13:00 to 17:00 because renewable energy generation is sufficient at this time.The integrated generation cost is lower than the microgrid’s selling price to the customer,and the microgrid operator does not curtail the customer loads to gain profit.For the microgrid to minimize RPI-based penalties while transmitting more power to the grid,the scheduling strategy proposed in this study curtails customer loads at most times of the day.
3.5 Comparison of curtailable loads curtailment
The final curtailment of the curtailable load is determined by the amount of curtailable load that can be curtailed at each moment and the optimal curtailment required by the microgrid.The microgrid curtails the amount of curtailable load that is optimal for the benefits of the microgrid through DLC.The curtailments of the curtailable loads for the scheduling strategy considering only the potential load curtailment versus the scheduling strategy proposed in this study are shown in Fig.14.The ratios of the curtailment of the curtailable loads to the total curtailable loads under different scheduling strategies are shown in Fig.15.
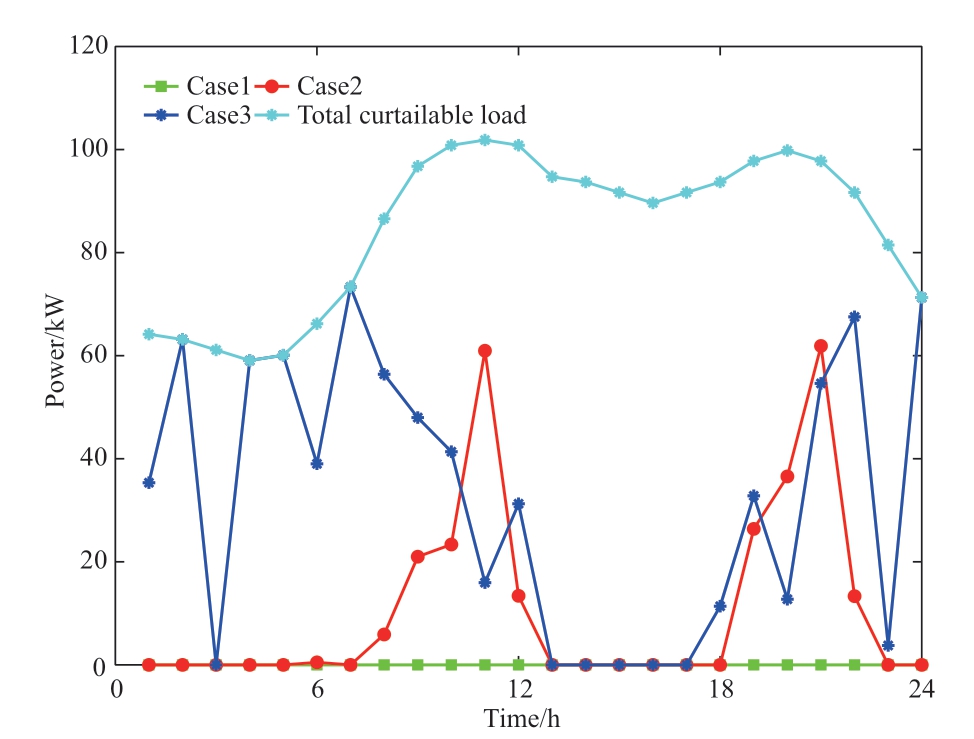
Fig.14 Curtailments for curtailable loads of different scheduling strategies
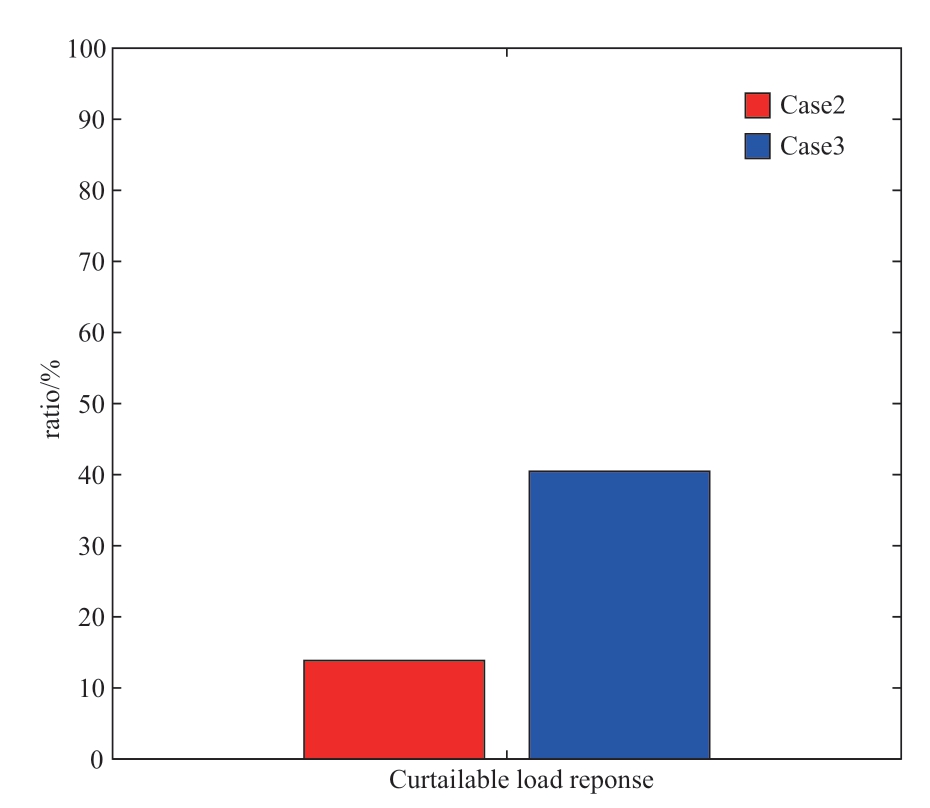
Fig.15 Ratio of the curtailments of curtailable load to the total curtailable load under different scheduling strategies
As shown in Figs.14 and 15,the scheduling strategy only considers potential load curtailment and curtails the curtailable loads only from 8:00-12:00 and from 21:00-22:00 to enhance the ability to transmit power to the grid.The scheduling strategy proposed in this paper curtails curtailable load in almost every period,except for 13:00-17:00 and 3:00,when there is an abundance of renewable energy or low customer load.The curtailment of curtailable load in the scheduling strategy that only considers potential load curtailment is 13.85% of the total curtailable load,and the curtailment of curtailable load in the scheduling strategy proposed in this paper is 40.47% of the total curtailable load.From the above analysis,it can be observed that the response of the curtailable load is more positive in the scheduling strategy proposed in this study.
4 Conclusion
In this study,an optimal day-ahead scheduling strategy for a microgrid considering regional pollution and potential load curtailment was proposed,which considers pollutant emissions and DR in each period.First,the RPI is proposed to assess the degree of environmental pollution caused by the microgrid during each period.On this basis,an RPIbased penalization mechanism for microgrid operators was proposed to reduce the generation of nonclean power supplies in microgrids.Second,a day-ahead scheduling strategy for a microgrid that considers the RPI and the curtailment potential of the curtailable load is proposed to enable the microgrid to obtain a stronger ability to transmit power to the grid and further reduce pollutant emissions during microgrid operation through direct load control.Finally,a microgrid was used as a case study to illustrate the superiority of the proposed optimal day-ahead scheduling strategy.
The case study demonstrated that the proposed RPI can accurately portray the degree of environmental pollution caused by microgrids.The penalty mechanisms based on RPI and customer compensation mechanisms based on curtailable load proposed in this study can effectively reduce pollutant emissions from microgrids in all periods.The optimal day-ahead scheduling strategy considering the RPI and potential load curtailment can reduce pollutant emissions from microgrids and improve the curtailable load responsiveness.However,the optimal RPI division values and penalty coefficients need to be explored further in future research.In subsequent studies,the types of loads that can be curtailed can be further divided in detail to analyze the effect of the customer compensation mechanism on the guidance of curtailable loads.
Acknowledgments
This work was supported in part by the Anhui Provincial Natural Science Foundation (No.2208085UD07) and National Natural Science Foundation of China (52377089).
Declaration of Competing Interest
The authors declare no conflicts of interest.
References
[1] Zhang J,Qin D,Ye Y C,et al.(2021) Multi-time scale economic scheduling method based on day-ahead robust optimization and intraday MPC rolling optimization for microgrid.IEEE Access,9:140315-140324
[2] Zhang Z T,Shi J,Yang W W,et al.(2023) Deep reinforcement learning based Bi-layer optimal scheduling for microgrids considering flexible load control.CSEE Journal of Power and Energy Systems,9(3): 949-962
[3] Liu Z H,Liu S L,Li Q H,et al.(2021) Optimal day-ahead scheduling of islanded microgrid considering risk-based reserve decision.Journal of Modern Power Systems and Clean Energy,9(5): 1149-1160
[4] Wei Z B,Zhang Z Q,Li Y J,et al.(2024) An integrated model of microgrid planning and operation under a multi-subject cooperation model in electric power construction.Electric Power Construction,early access: 1-15 (in Chinese) https://link.cnki.net/urlid/11.2583.TM.20240402.1123.012
[5] Xin X,Ouyang S,Huang Y,et al.(2024) Optimal economic configuration and reliability evaluation of multiple power supply ensuring microgrid considering energy storage capacity attenuation.Electric Power Construction,early access: 1-12 https://link.cnki.net/urlid/11.2583.TM.20240402.0936.006
[6] Zhang L,Liu Y H,Jia C H,et al.(2023) A coordinated control strategy for a wind/ photovoltaic/ gas turbine/ storage microgrid based on a multi-partition adaptive improved fruit fly algorithm.Power System Protection and Control,51(22): 13-23 (in Chinese)
[7] Yousif M,Ai Q,Gao Y,et al.(2020) An optimal dispatch strategy for distributed microgrids using PSO.CSEE Journal of Power and Energy Systems,6(3): 724-734
[8] Ding Z H,Lee W J (2016) A stochastic microgrid operation scheme to balance between system reliability and greenhouse gas emission.IEEE Transactions on Industry Applications,52(2):1157-1166
[9] Hou H,Gan M,Wu X X,et al.(2023) Real-time energy management of low-carbon ship microgrid based on name="ref10" style="font-size: 1em; text-align: justify; text-indent: 2em; line-height: 1.8em; margin: 0.5em 0em;">[10] Ishraque M F,Shezan S A,Rashid M M,et al.(2021) Technoeconomic and power system optimization of a renewable rich islanded microgrid considering different dispatch strategies.IEEE Access,9: 77325-77340
[11] Mutarraf M U,Guan Y J,Terriche Y,et al.(2022) Adaptive power management of hierarchical controlled hybrid shipboard microgrids.IEEE Access,10: 21397-21411
[12] Parks K (2019) Declining capacity credit for energy storage and demand response with increased penetration.IEEE Transactions on Power Systems,34(6): 4542-4546
[13] Kovačević M,Vašak M (2023) Aggregated representation of electric vehicles population on charging points for demand response scheduling.IEEE Transactions on Intelligent Transportation Systems,24(10): 10869-10880
[14] Wang S Y,Bi S Z,Zhang Y J A (2018) Demand response management for profit maximizing energy loads in real-time electricity market.IEEE Transactions on Power Systems,33(6):6387-6396
[15] Zhu L,Yan Z,Lee W J,et al.(2015) Direct load control in microgrids to enhance the performance of integrated resources planning.IEEE Transactions on Industry Applications,51(5):3553-3560
[16] Gao Y,Ai Q (2021) Demand-side response strategy of multimicrogrids based on an improved co-evolution algorithm.CSEE Journal of Power and Energy Systems,7(5): 903-910
[17] Freire V A,De Arruda L V R,Bordons C,et al.(2020) Optimal demand response management of a residential microgrid using model predictive control.IEEE Access,8: 228264-228276
[18] Ali M,Abdulgalil M A,Habiballah I,et al.(2023) Optimal scheduling of isolated microgrids with hybrid renewables and energy storage systems considering demand response.IEEE Access,11: 80266-80273
[19] Trujillo D,García Torres E M (2022) Demand response due to the penetration of electric vehicles in a microgrid through stochastic optimization.IEEE Latin America Transactions,20(4):651-658
[20] Dou C X,Zhou X H,Zhang T F,et al.(2020) Economic optimization dispatching strategy of microgrid for promoting photoelectric consumption considering cogeneration and demand response.Journal of Modern Power Systems and Clean Energy,8(3): 557-563
[21] Wang B,Zhang C,Dong Z Y (2020) Interval optimization based coordination of demand response and battery energy storage system considering SOC management in a microgrid.IEEE Transactions on Sustainable Energy,11(4): 2922-2931
[22] Li Y,Li K (2019) Incorporating demand response of electric vehicles in scheduling of isolated microgrids with renewables using a Bi-level programming approach.IEEE Access,7:116256-116266
[23] Muqeet H A U,Ahmad A (2020) Optimal scheduling for campus prosumer microgrid considering price based demand response.IEEE Access,8: 71378-71394
[24] Tang Z Y,Hill D J,Liu T (2018) A novel consensus-based economic dispatch for microgrids.IEEE Transactions on Smart Grid,9(4): 3920-3922
[25] Kanchev H,Colas F,Lazarov V,et al.(2014) Emission reduction and economical optimization of an urban microgrid operation including dispatched PV-based active generators.IEEE Transactions on Sustainable Energy,5(4): 1397-1405
[26] Zhang Y,Hajiesmaili M H,Cai S N,et al.(2018) Peak-aware online economic dispatching for microgrids.IEEE Transactions on Smart Grid,9(1): 323-335
[27] Babazadeh-Dizaji R,Hamzeh M (2020) Distributed hierarchical control for optimal power dispatch in multiple DC microgrids.IEEE Systems Journal,14(1): 1015-1023
[28] Mahmoodi M,Shamsi P,Fahimi B (2015) Economic dispatch of a hybrid microgrid with distributed energy storage.IEEE Transactions on Smart Grid,6(6): 2607-2614
[29] Sun J L,Hu C H,Liu L,et al.(2022) Two-stage correction strategy-based real-time dispatch for economic operation of microgrids.Chinese Journal of Electrical Engineering,8(2): 42-51
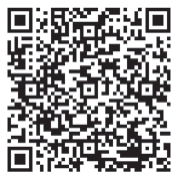
Scan for more details
Received: 26 January 2024/Revised: 22 April 2024/Accepted: 31 May 2024/Published: 25 December 2024
Hejun Yang
cquyhj@126.com
Xinghua Xie
2021170461@mail.hfut.edu.cn
Bo Wang
atzs_99@hotmail.com
Yinghao Ma
yinghao_ma@126.com
Dabo Zhang
zhangdb2004@163.com
Yuming Shen
syming1988@126.com
2096-5117/© 2024 Global Energy Interconnection Group Co.Ltd.Production and hosting by Elsevier B.V.on behalf of KeAi Communications Co.,Ltd.This is an open access article under the CC BY-NC-ND license (http://creativecommons.org/licenses/by-nc-nd/4.0/).
Biographies
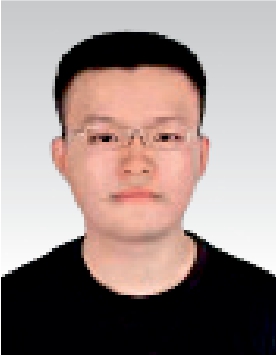
Xinghua Xie received the B.S.degree at North China Electric Power University,Hebei,China,in 2018.He is working towards the M.S.degree at Hefei University of Technology,Hefei,China.His research interests include planning and operation of microgrid and demand response.
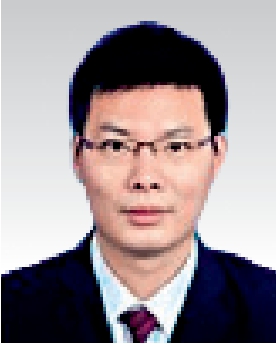
Hejun Yang received the Ph.D.degree in electrical engineering from Chongqing University,Chongqing,China,in 2014.He is currently an associate professor in school of electrical engineering and automation,Hefei University of Technology,Hefei,China.His main research interests include power system planning and reliability and demand response.
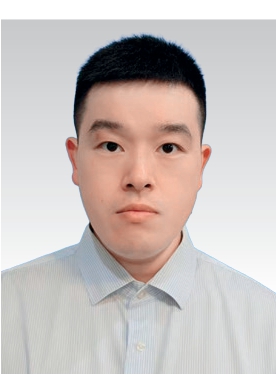
Bo Wang obtained a bachelor’s degree in Power System and Automation from Xi’an Jiaotong University in 2014.He is currently employed at Hangzhou Science and Technology Development Branch of Zhejiang Dayou Industrial Co.,Ltd.His research interests focus on the reliability and implementation of automated operation in power communication distribution networks.
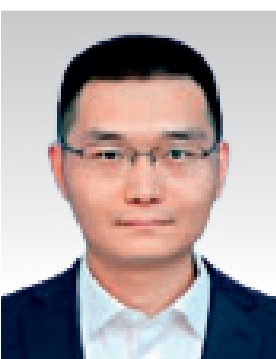
Yinghao Ma received the Ph.D.degree in electrical engineering from Chongqing University,Chongqing,China.He is currently an Assistant Professor in school of electrical engineering and automation,Hefei University of Technology,Hefei,China.His research interests include power system planning and reliability.
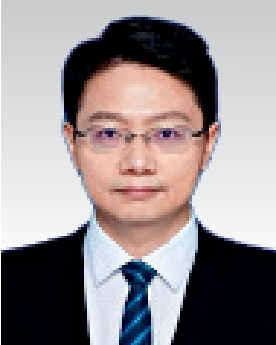
Dabo Zhang received a Ph.D.degree in Electrical Engineering from Chongqing University,Chongqing,China,in 2012.He is currently an associate professor with the School of Electrical Engineering and Automation,Hefei University of Technology,China.His research interests include power system reliability evaluation,security monitoring,and artificial intelligence.
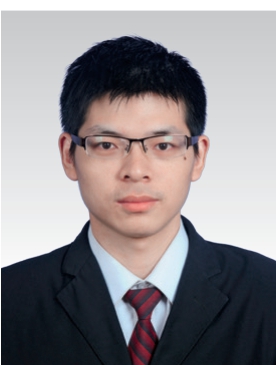
Yuming Shen received a M.S.degree in electrical engineering from Chongqing University,Chongqing,China,in 2014.He is currently an senior engineer in Institute of Economy and Technology,State Grid Anhui Electric Power Co.,Ltd.,Hefei,China.His main research interests include main network planning,new energy and energy storage planning.
(Editor Yanbo Wang)