0 Introduction
As per the “Guiding Opinions on Accelerating the Development of a National Unified Electricity Market System” jointly published by the National Development and Reform Commission and the National Energy Administration,China is pursuing to “improve the market mechanism for adaptability with new electric power systems,develop a national unified electricity market system,accelerate the development of electricity ancillary service markets,and promote trial operation of electricity spot markets in key regions.” Several Chinese provinces have been conducting trials on electricity market trading.As a major aspect of electric power system reforms,the development of an efficient and safe electricity market promotes the sustainable development of the power industry and contributes to the realization of “carbon peak and carbon neutrality” goals.Safe and efficient operation of the electricity market is dependent on proper regulation and effective assessment of the market operation mechanism.
In the wake-up of the power market reform,issues have emerged in terms of development and operation.The development of electricity market operational assessment systems capable of effectively diagnosing vulnerabilities has attracted increasing attention from researchers [1,2].Currently,different countries place different emphases on the establishment of an evaluation index system and evaluation methods for their own electricity markets.This has hampered the development of a uniformly recognized system for electricity market operational assessment.The European Commission assesses the efficiency of electricity markets using criteria and big data,considering four dimensions: market design,market power,market regulation,and sustainable development [3].The United States implements a regional electricity market system,with the Federal Energy Regulatory Commission enacting laws to regulate the market behavior of market entities and independent regulators of regional electricity markets performing long-and short-term market assessments.The electricity market assessment system of the United Kingdom comprises three levels: analysis of market performance by researchers based on local market criteria,assessment of market entities from the company’s perspective,and assessment of the effectiveness of market reforms via consulting firms.In general,these methods investigate and analyze the operation of electricity markets by calculating quantitative criteria.
Dong et al.proposed an improved matter-element extension cloud model for the operational assessment of electricity markets.Consequently,they developed an index system for electricity market operational assessment comprising indexes in five categories: supply side,demand side,coordinated operation,market security,and sustainable development [4].Yu et al.proposed a multi-agent system method for evaluating market rules and constructed simulation scenarios for evaluating the California electricity market.They demonstrated that in the absence of market power mitigation,generation companies facilitated by Q-learning could exploit market flaws and realize higher profits [5].Xia et al.investigated electricity market regulation systems in the United States and other countries,and provided an analysis and summary of these systems in terms of the classification of disclosed information,definition of regulation and assessment criteria,and key regulatory measures [6].Jian et al.considered the different needs of different market participants,including electricity price,reliability of electricity supply,service level,selectivity concerning users,market information disclosure,and market power behavior concerning market mechanism designers.Based on this,they proposed that electricity market assessment should provide the different information required by different market participants [7].Research on electricity market assessment in China has primarily focused on trading mechanisms,regulations,and risk methods.Wang et al.compared the trading mechanisms and backgrounds of electricity markets in China and other countries.They discussed the principles and objectives for the design of electricity market trading mechanisms.Consequently,they developed a hierarchical index system for electricity market trading assessment using market liquidity,stability,economic efficiency,coordinated development,and openness as first-level indices [8].Based on the development and information disclosure of the electricity market in the Guangdong Province,Shi et al.developed a model for the comprehensive assessment of electricity markets that covered three dimensions: efficiency,fairness,and development,to identify the key indexes affecting the Guangdong electricity market [9].Zhao et al.developed an index system for the self-regulation of electricity markets.This was intended to help trading participants implement advanced self-regulation to comply with the regulatory requirements for electricity trading and provide decisionmaking criteria for improving trading behavior through the analysis of key information affecting the effectiveness of self-regulation [10].Zhang et al.developed a model to comprehensively assess the operational risk of power grid companies participating in competitive electricity markets.They aimed to provide reference criteria for controlling the operational risk of power grid companies by quantitatively assessing the risks associated with their participation in competitive markets using an index system [11].Wang et al.proposed a new medium -and long-term power security analysis model based on market members’ willingness to trade,taking into account power security analysis and power constraints to reduce the production and operation risks of market members [12].Ren et al.proposed a joint clearing model for both sides of power generation and power consumption,and designed the settlement and distribution mechanism of auxiliary peak regulation services considering the contribution and benefit degree of each market entity[13].Based on the development trend of the Chinese electricity market and the current status of the Chinese power industry,Li and Wang designed an index system for an electricity market trading assessment that covered three dimensions: fundamentals of the power industry,market design,and actual trading,and realized cross-sectional and longitudinal comparisons of different electricity markets at different times [14].Pu et al.proposed an index system for electricity balance analysis comprising the following indices: congestion,adjustable capacity,load,adequacy,electricity supply order,energy conservation,environmental conservation,and economic efficiency [15].Liu et al.evaluated China’s regional auxiliary service market transactions by applying the gray correlation TOPSIS method on the basis of analyzing the development of power markets in Europe and the United States [16].Fang et al.designed an evaluation index system for the shared energy storage market based on the trading rules and structurebehavior-performance (SCP) analysis model of Qinghai Province,China,and applied AHP-TOPSIS method for evaluation [17].
Studies on electricity market evaluation have focused on the behavior of the main body exercising market power.Cheng et al.[18] evaluated the market power of hydropower enterprises in the power market by constructing an improved Weighted Maintenance object (WMO) model.They found that hydropower stations and large installed entities had strong market power.Further,collusion was common in market transactions.Lin et al.constructed a joint market power assessment and mitigation framework based on an emerging power day-ahead market and applied the proposed CMO-BWM-entropy model to evaluate power markets with construction maturity in different provinces in China [19].Lee et al.studied the market power of firms in a transmission-constrained power market and used two methods to evaluate the market power of firms,considering the complementarity of the results [20].
To evaluate market operations,scholars have their own focuses.Su et al.studied the tools and methods of electricity market evaluation and found that the main evaluation indicators included factors such as the optimal allocation of resources and market efficiency of sustainable development[21].Cui et al.established a hierarchical evaluation system for electricity markets based on the construction of electricity markets in developing countries,including market competition,level of openness,and market efficiency [22].To address the problems of fuzziness and randomness in power market operation evaluation,Dawei et al.combined the uncertainty analysis method of a cloud model with the matter-element extension method and introduced credibility and risk factors to reflect the credibility degree and risk of the model [23].Credit evaluation is an important component of power-market management.Xie et al.established a subject-oriented power market credit evaluation index system from the three aspects of market behavior,financial status,and social responsibility and realized the credit evaluation of enterprises with different market behaviors [24].Liu et al.proposed a performance evaluation system for the power market.They constructed a power market operation performance evaluation model based on the AHP-IEWM-ITOPSISI [25].Streimikiene et al.studied the impact of the opening up of the electricity market on the sustainability of the electricity market model and selected the indicators that exerted the greatest impact on sustainability through a sustainability assessment of the organizational structure of different global electricity markets [26].Li et al.evaluated the effect of energy saving and emission reduction of market players on the electricity selling side,determined the index weights using an analytic hierarchy process and entropy weight method,and performed a multilevel fuzzy comprehensive evaluation [27].
By reading and summarizing the above literature,it was found that existing research on electricity market evaluation has the following aspects that require further improvement.1) Numerous studies on electricity market evaluation at home and abroad have primarily focused on transaction user behavior in the market and rarely considered the transaction structure,transaction type,and other factors in the existing market from the perspective of market transaction institutions.2) Few studies have conducted in-depth evaluations on the construction of provincial power markets based on the actual situation of China’s power market.3) The power market evaluation index system constructed in the existing literature is limited to the availability of data.However,there are more qualitative indicators in the index,which can add more subjective factors.
Therefore,this study aimed to design a comprehensive and systematic evaluation system for power market construction.Three core areas of market design,market efficiency,and development coordination were considered.The index system was rendered as quantitative as possible.Its primary purpose is to accurately evaluate the perfection of China’s provincial power market construction,reveal its advantages and disadvantages,extract meaningful power market construction schemes for reference,and provide strong support for the further development of China’s power market.The major contributions of this study are summarized as follows:
1) A system for the operational assessment of electricity markets was proposed based on a review of research on the operational assessment of electricity markets and the trial operation of provincial electricity markets in China,while considering China’s objective of developing a national unified electricity market using the experience gained from the trial operation of provincial electricity markets.Different from other research subjects’ behavior in the market and market transaction risk assessment,this study focused on the market construction,and introduced indices such as“Medium-and long-term contract ratio (C1)” and “KWh revenue cost index(C5)” into the index system to conduct a more extensive and in-depth study on the construction perfection of the power market.Simultaneously,to make the evaluation results more accurate,this study constructed an evaluation index system based on the principle of selecting as many quantitative indicators as possible.
2) A multi-criterion decision-making method for power market operation evaluation combining the fuzzy BWM and fuzzy COPRAS was proposed.By extending the traditional COPRAS method to the fuzzy environment,the obtained fuzzy COPRAS method incorporates the uncertainty in the evaluation,thus increasing the robustness of the evaluation results and avoiding the influence of extreme index values in the traditional COPRAS on the evaluation results.
The remainder of this paper is organized as follows.Section 1 describes the index system for the electricity market operational assessment,which covers three dimensions: market design,market efficiency,and coordinated development.Section 2 describes the operational assessment method that combines a fuzzy BWM to determine the weights of indices and a fuzzy COPRAS method to improve the traditional COPRAS method.Section 3 presents a case analysis wherein the proposed model is applied to an operational assessment of four provincial electricity markets in China to verify its effectiveness.Recommendations for market development are also presented.Section 4 provides an in-depth discussion of the assessment results.Finally,Section 5 summarizes the conclusions of the study.
1 Index system for comprehensive operational assessment of Chinese electricity markets
A comprehensive assessment of the effects of potential changes on market rules is difficult because of the complexities associated with the market structure,strategic interactions between participants,and potential physical factors.The index system should be designed according to the principles described below to ensure a comprehensive and accurate operational assessment of the electricity markets.
1) Economics: The economics of an electricity market trading mechanism reflects its “price discovery” function.Therefore,the operational assessment system of an electricity market must include price-related criteria.
2) Reliability: The energy and power industries are critical to the national economy and people’s livelihoods.The reliable and stable operation of the electricity market is a fundamental necessity for the steady development of the national economy.Therefore,an assessment of electricity markets must consider indices that measure the stability and reliability of their development and operations.
3) Fairness and equity: One of the primary objectives of China’s electricity reforms is to break monopolies,introduce orderly competition into the power industry,and assess the market’s decisive role in resource allocation.This necessitates an index system for electricity market operational assessment,comprising indices that measure fairness.Good electricity market design must facilitate orderly competition,efficient operation,and the dynamic development of market entities.
Following basic principles and general ideas,an index system for electricity market operational assessment was designed by first identifying the major indices.An expert group comprising six experts was established,with three experts in electricity trading with extensive experience working at electricity trading centers,and three researchers with experience in electricity market development and electricity trading rules.The major indices for the electricity market operational assessment were identified by an expert group after several discussion sessions.Many factors affect the operations of electricity markets.However,according to the enslaving and order parameter principles of synergetics,the evolution of a system is dominated by a few order parameters [28].Therefore,a preliminary list of indices was reviewed by an expert group and screened.Finally,an index system for the electricity market operational assessment comprising nine indices in three dimensions was obtained,as shown in Fig.1.
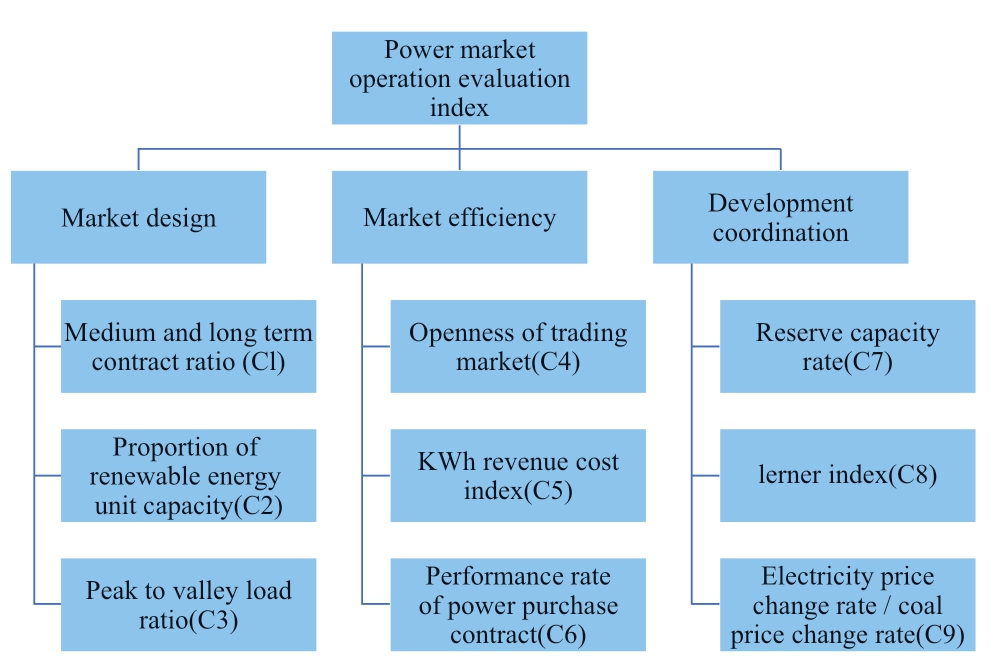
Fig.1 Index system for electricity market operational assessment
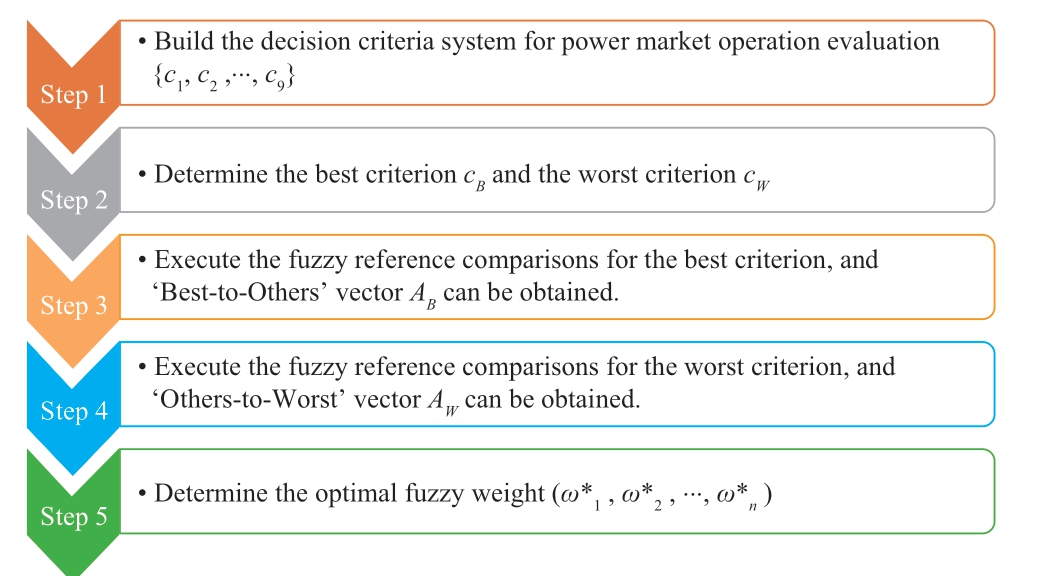
Fig.2 Operating steps of the fuzzy BWM
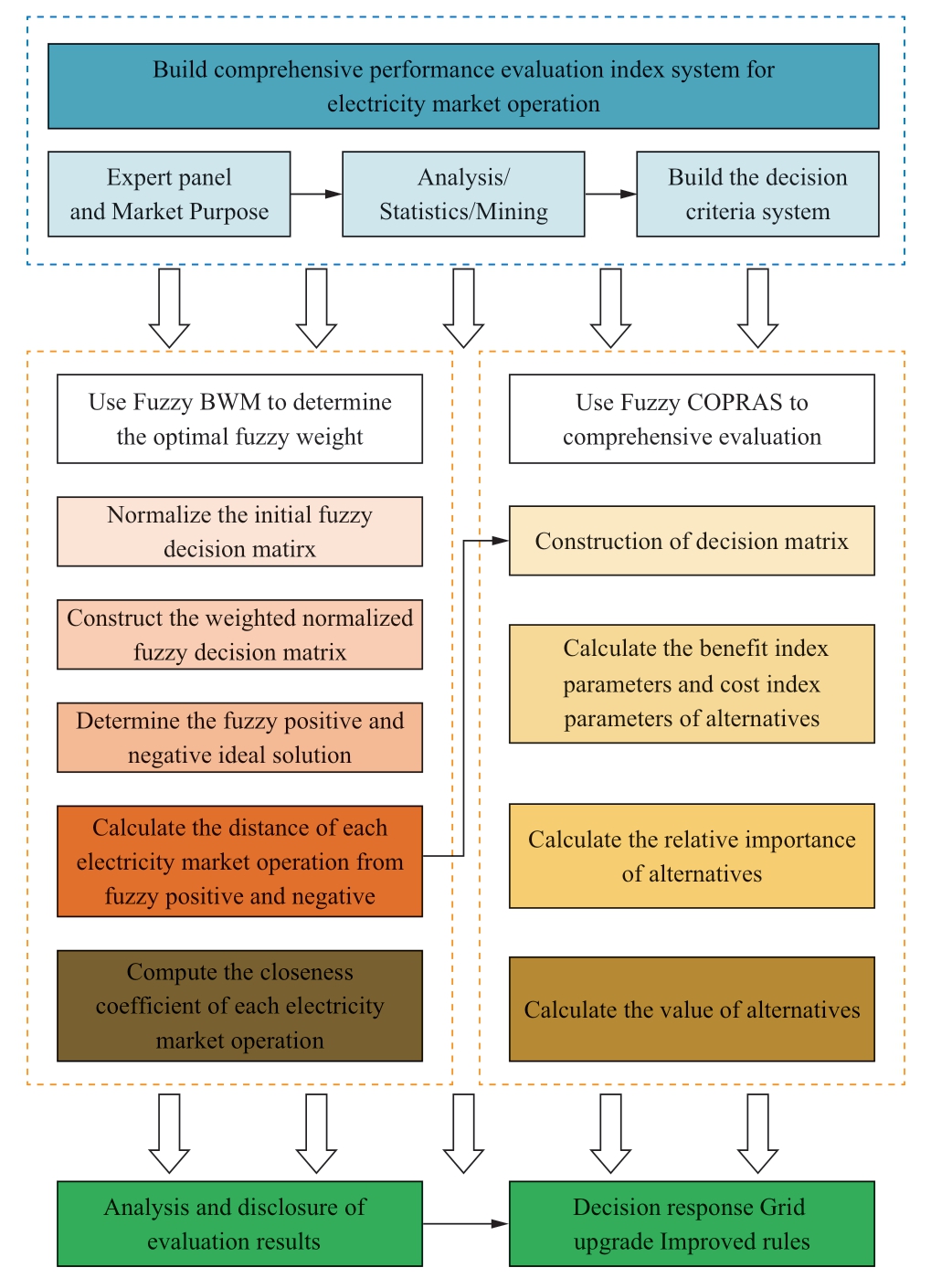
Fig.3 Framework of the proposed method for electricity market operational assessment and its implementation procedure
The index system comprised both quantitative and qualitative indices.The quantitative indices included positive,reverse,and neutral indices.For a positive index,a larger value is more desirable.A smaller value indicates a negative index.For a neutral index,a value closer to the center is more desirable.A qualitative index cannot be easily measured using specific data.Considering that different indices have different definitions and measurement criteria,the indices were divided into two categories: qualitative and quantitative.There is only one qualitative index:openness of the trading market.There are eight quantitative indices: medium-and long-term contract ratio,proportion of renewable energy unit capacity,load peak-to-valley ratio,kWh revenue cost index,performance rate of power purchase contract,reserve capacity rate,Lerner index,and electricity price change rate/coal price change rate.
Table 1 lists the index system for the electricity market operational assessment with a definition for each index.These nine indexes help simplify the question and reflect the inherent characteristics of the effective operation of electricity markets.
Table 1 Definition of indexes for electricity market operational assessment
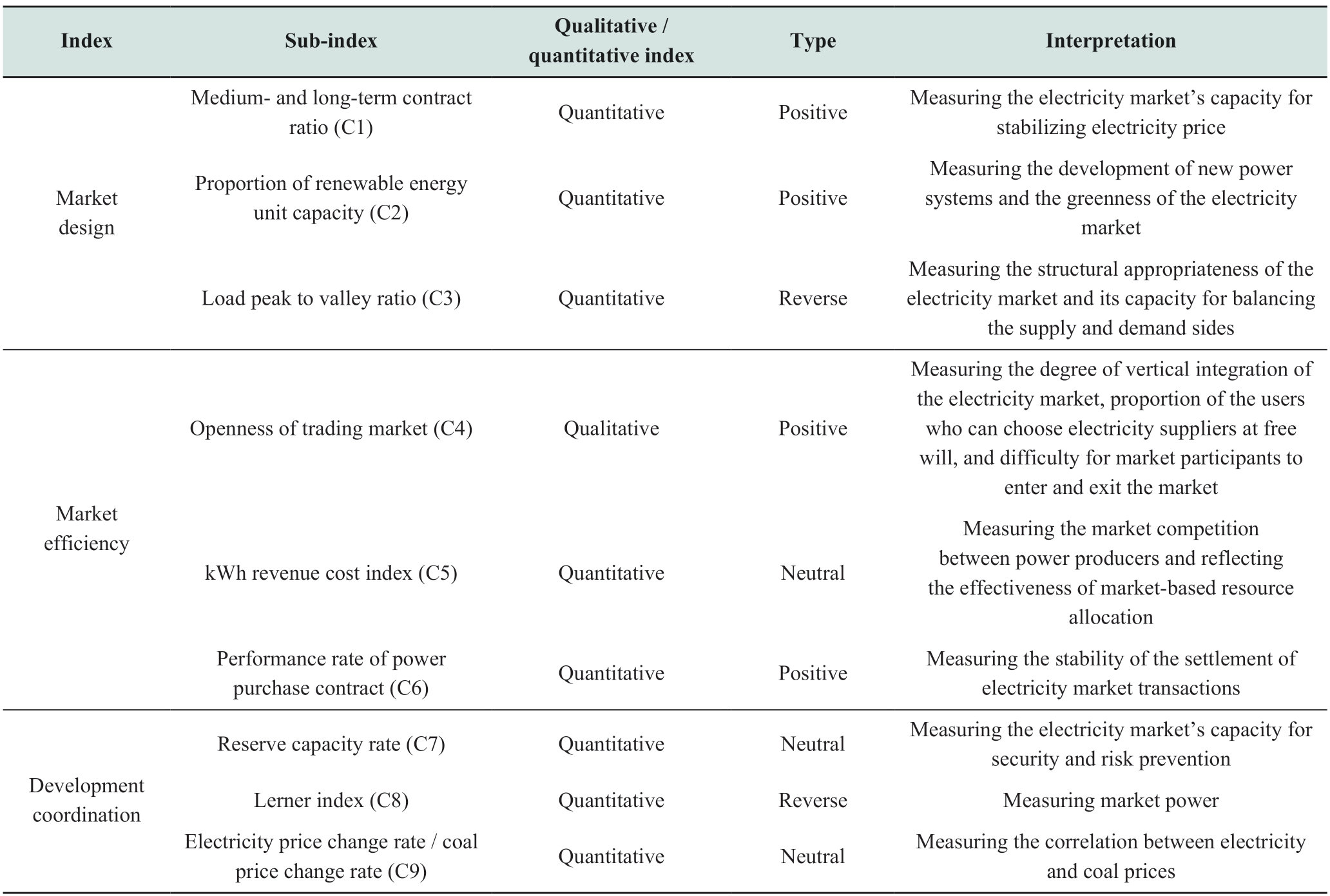
There are three indices in the market design dimension.First,the medium-and long-term contract ratio (C1) for measuring the electricity market’s capacity for stable control of electricity prices.Second,the proportion of renewable energy unit capacity (C2) for measuring the development of new energy and greenness of the electricity market.Third,the load peak-to-valley ratio (C3) for measuring the structural appropriateness of the electricity market and its capacity to balance the supply and demand sides,which is defined as the ratio of the peak to the valley of the load curve for a particular period.
There are three indices in the market efficiency dimension.First,the openness of the trading market (C4) for measuring the degree of integration of the electricity market,the proportion of users who can choose power suppliers at their free will,and the difficulty for market participants to enter and exit the market.Second,the kWh revenue cost index (C5) for measuring the market competition between power producers and the efficiency of market-based resource allocation.Third,the performance rate of power purchase contracts (C6) for measuring the stability of the settlement of electricity market transactions,which is defined as the ratio of the performed electricity purchase contracts to all electricity purchase contracts in a given period.
There are three indices in the coordinated development dimension.First,the reserve capacity rate (C7) for measuring the level of security of the electricity market and its capacity for risk prevention,which is defined as the ratio of the reserve capacity to the peak load.Second,the Lerner index (C8) for measuring market power,which is defined as the ratio of the electricity price to the marginal cost of power generation Third,the electricity price change rate/coal price change rate (C9) for measuring the correlation of electricity price change with coal price change;to a certain degree,this reflects the interactions between the electricity and other markets.
2 Fuzzy multi-criteria decision-making(MCDM) method for electricity market operational assessment
The development of an index system and model for an electricity market operational assessment is a systematic project.Assessment indices can reflect the operational performance of the electricity market under the facilitations and constraints imposed by the market operation rules.An assessment of an electricity market against these indices can reveal the strengths and weaknesses of its operations.The Chinese electricity market should be designed according to the principles of safe and efficient operation and sustainable development [28,29].The proposed electricity market operational assessment system was hierarchical Any factor at a particular level could be influenced by the combined effects of multiple factors at the next-lowest level.The operational assessment of electricity markets involves the following major problems:
1) Assessment indices are fuzzy or uncertain and may be correlated with each other.
2) The assessor may use fuzzy language to assess an index because of his/her limited understanding,leading to uncertainties in the subjective judgment.Moreover,the collected information may be incomplete for index assessment.
3) Different assessor types may provide conflicting judgments regarding the same assessment index under the same circumstances because of their different interests or cognitions.
4) Inherent conflicts may exist between different assessment indexes,such as market efficiency and market risk.
Such fuzziness and uncertainties associated with assessment indices and assessors can be reduced using a fuzzy multi-attribute decision-making method.Therefore,in this study,a fuzzy multi-attribute decision-making method was developed for electricity market operationalassessment that combines a fuzzy BWM to determine the weights of indices and an improved COPRAS method for the operational assessment of electricity markets.
2.1 Fuzzy BWM for determining the weights of indexes
BWM is an MCDM method first proposed by Rezaei[30].Compared with other MCDM methods [31-34],the BWM calculates and comprehensively assesses the weights of indices through pairwise comparisons.However,in the index decision-making process,owing to the low accuracy of complete information and uncertainties in expert qualitative judgments,the weights of a set of indices determined using exact values are often flawed and less fault-tolerant.Therefore,several studies have attempted to improve BWM,for example,fuzzy BWM [35,36].In this study,the fuzzy BWM was used to determine the weights of the nine indices in the three dimensions of the index system for electricity market operational assessment.Subsequently,the indices were ranked based on expert consensus against the requirements for the development of the Chinese electricity market.The fuzzy BWM was implemented using the following procedure:
Step 1: Building an assessment index system.
The index system for electricity market operational assessment comprises nine indexes: {c1,c2,...,c9 }.
Step 2: The best (most important) and worst (least important) indices are determined.
The best (most important) and worst (least important)indices in the set of indices for electricity market operational assessment were determined based on expert consensus.The best and worst indices were designated CB and CW,respectively.
Step 3: Performing fuzzy reference comparisons.
Fuzzy reference comparisons refer to fuzzy comparisons between the reference indices and other indices,which may involve the use of linguistic variables provided by the expert group.These linguistic variables are converted to degrees of fuzziness,expressed as triangular fuzzy numbers (TFNs),using the rules given in Table 2 [37,38].
Table 2 Rules for converting decision maker linguistic variables to TFNs
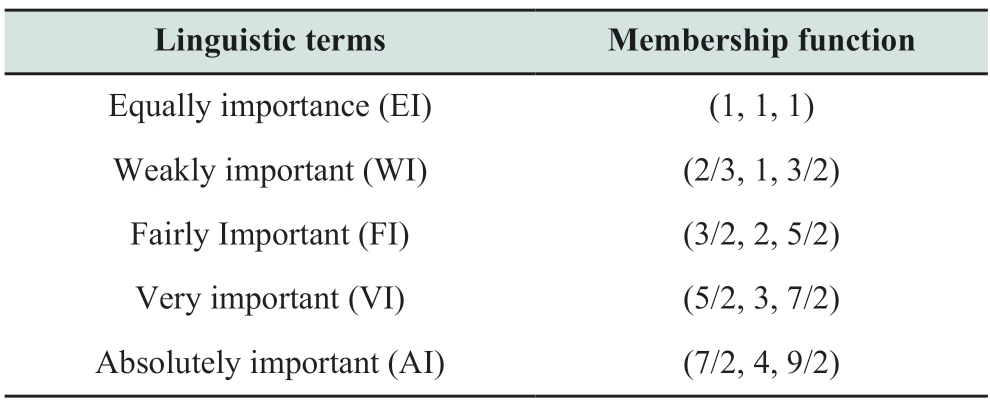
Thereby,the following fuzzy comparison matrix can be obtained:
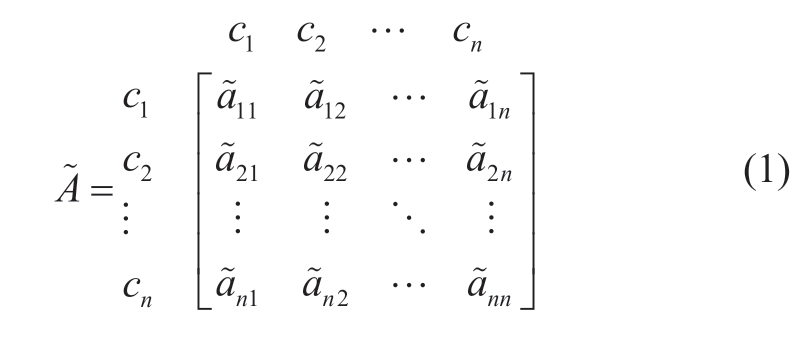
where is the relative fuzzy preference of the i-th index over the j-th index,and is a triangular fuzzy number.In the fuzzy BWM,fuzzy reference comparisons include fuzzy pairwise comparisons between the best index and other indexes and between the worst index and other indices.In this step,fuzzy pairwise comparisons between the best index and other indices are performed first [39]:

where is the fuzzy best-to-others vector and
is the fuzzy preference of the best index CB relative to the j-th index.
Step 4: Performing fuzzy reference comparisons with the worst index.
In this step,fuzzy pairwise comparisons between the worst index and other indices are performed as follows:

where is the fuzzy worst-to-others vector and
is the fuzzy preference of the i-th index relative to the worst index,i=1,2,…,n .
Step 5: Determining the optimal fuzzy weights
For each fuzzy pairand
and
are used [40].To determine the optimal weight of an index,the distance between the resulting weight and the decision maker’s pairwise comparison is minimized.Therefore,to determine the weights of the indices in the BWM,the following constrained optimization problem is formulated [41]:
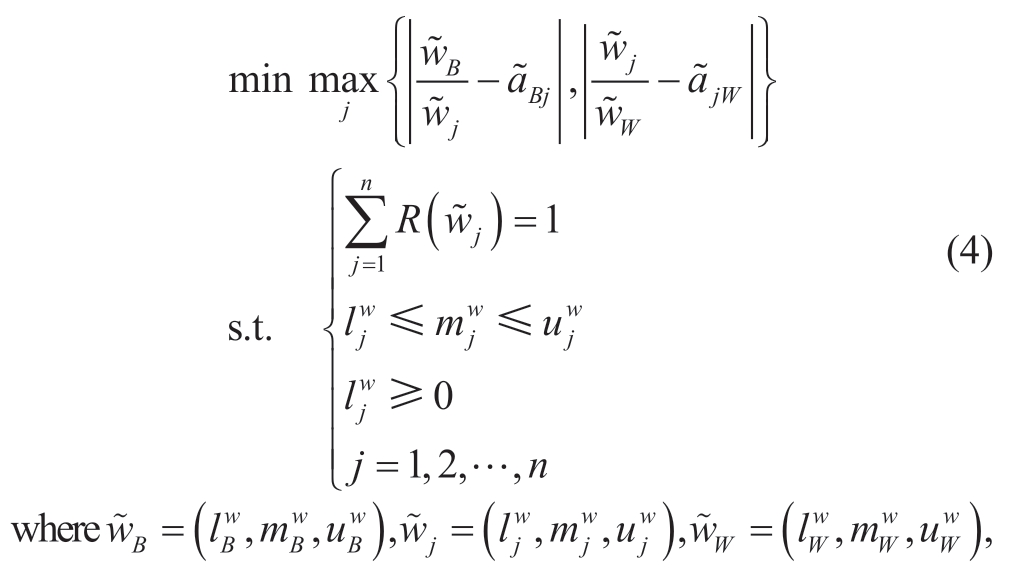

To obtain a more accurate optimum solution,(4) is transformed using the method presented in [39] to treat nonlinear constrained optimization problems as follows:
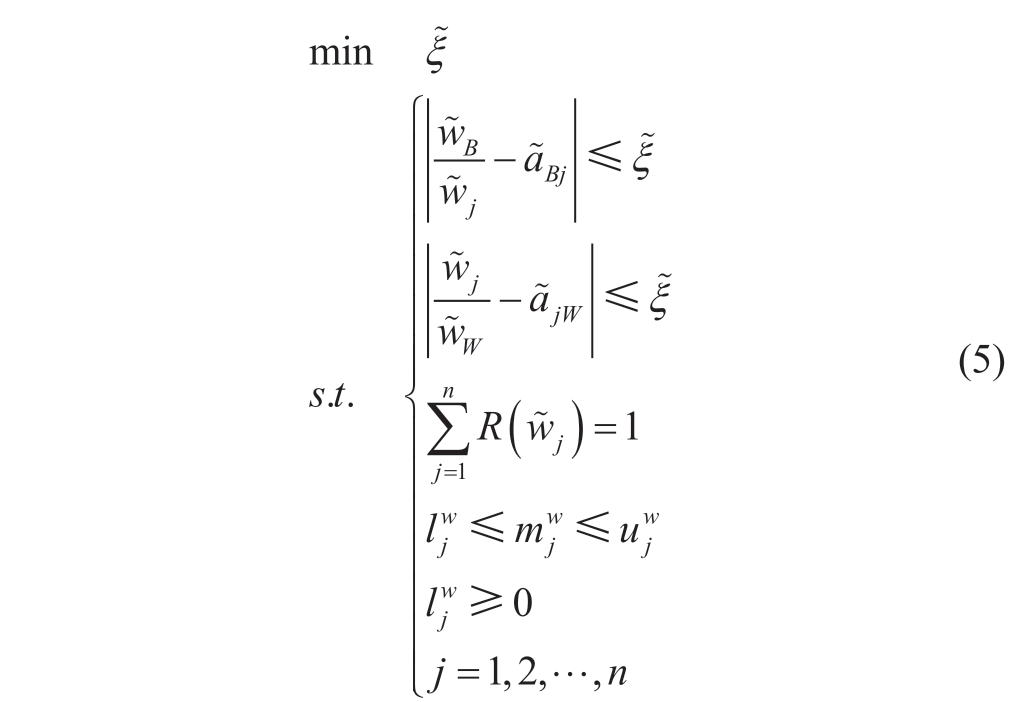
where ξ~=(lξ,mξ,uξ).
Considering lξ ≤ mξ ≤ uξand simplifying the solution,=(k *,k *,k*) and k*≤ lξ were assumed.Therefore,(5)can be transformed as follows:
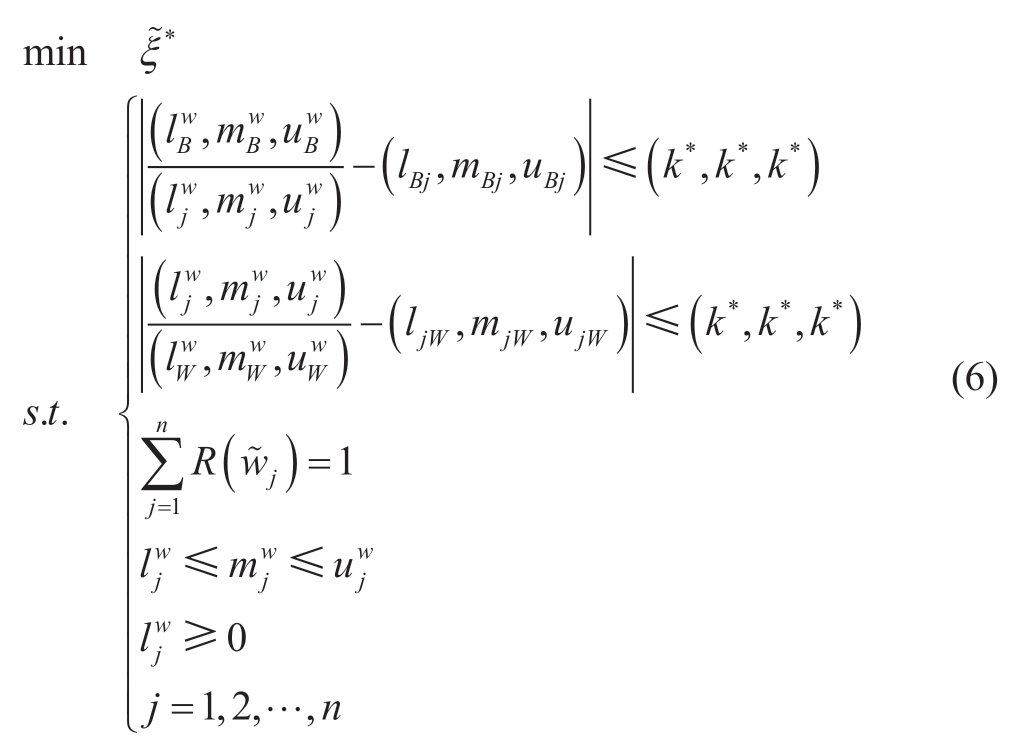
By solving this equation,the optimum fuzzy weights of the indices for the electricity market operational assessmentare obtained.
Figure 2 shows the operating steps of the fuzzy BWM.
The fuzzy BWM was incorporated with a consistency ratio (CR) to measure the consistency of fuzzy pairwise comparisons,which was calculated using (7).Table 3 lists the CR values of the BWM for different values of CB and CW.A CR value smaller than 0.1 indicates that the fuzzy pairwise comparisons are consistent and the resulting fuzzy weights are acceptable.Table 4 lists the CR values of the fuzzy BWM.

Table 3 Consistency index (CI)

Table 4 CR values of fuzzy BWM
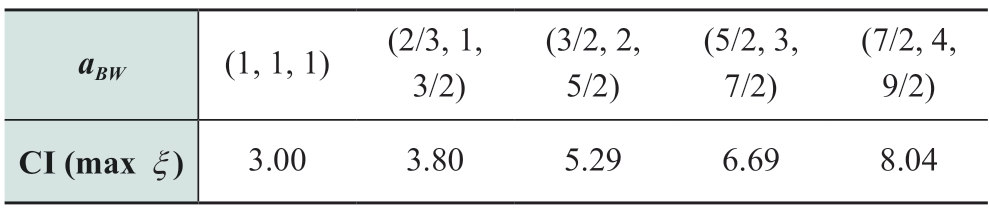
2.2 Fuzzy COPRAS method for electricity market operational assessment
The COPRAS method was proposed by Zavadskas et al.to improve the overall efficiency of the solutions to be assessed [41].Previously,the COPRAS method was widely used in areas such as photovoltaic plant location selection[42-44],energy [45-47] and climate sustainability research[48-50],and distributed energy systems [51-53].Therefore,in this study,the COPRAS method was extended to the fuzzy domain and used for the operational assessment of electricity markets.
In contrast to the traditional COPRAS method,the fuzzy COPRAS method yields a decision-making matrix of variables expressed in TFNs [54].The operating steps of the fuzzy COPRAS method for electricity market operational assessment are as follows:
1) Formulation of the decision-making matrix
For a decision-making problem involving m alternative solutions and n indices,the index score for each alternative can be expressed using a decision-making matrix.
The proposed index system for the electricity market operational assessment comprised both qualitative and quantitative indices.The quantitative index was assessed using data from research reports and internal data from electricity trading centers.The qualitative index was converted to TFNs.
To obtain the fuzzy linguistic value of the qualitative index in the index system for the electricity market operational assessment,the fuzzy linguistic value of each qualitative index for the electricity market operational assessment was provided by the expert group,as shown in Table 5.
Table 5 Rules for quantification of linguistic variables
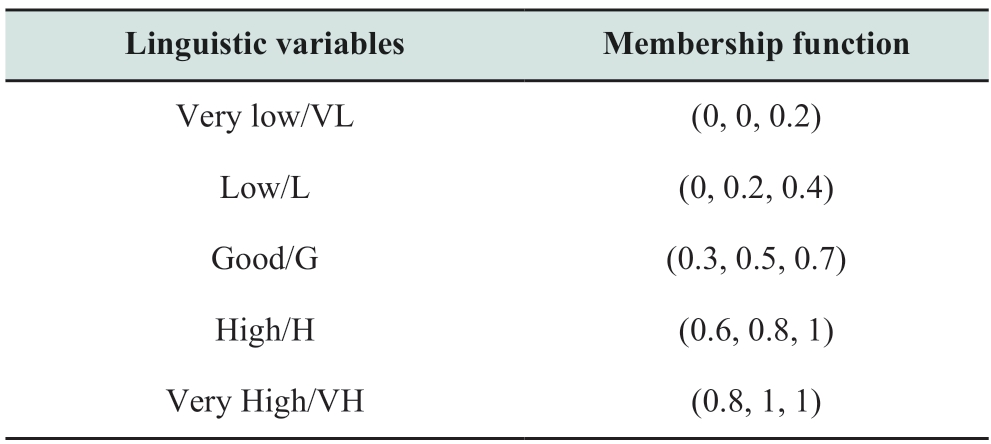
The matrix was formulated and treated as follows:
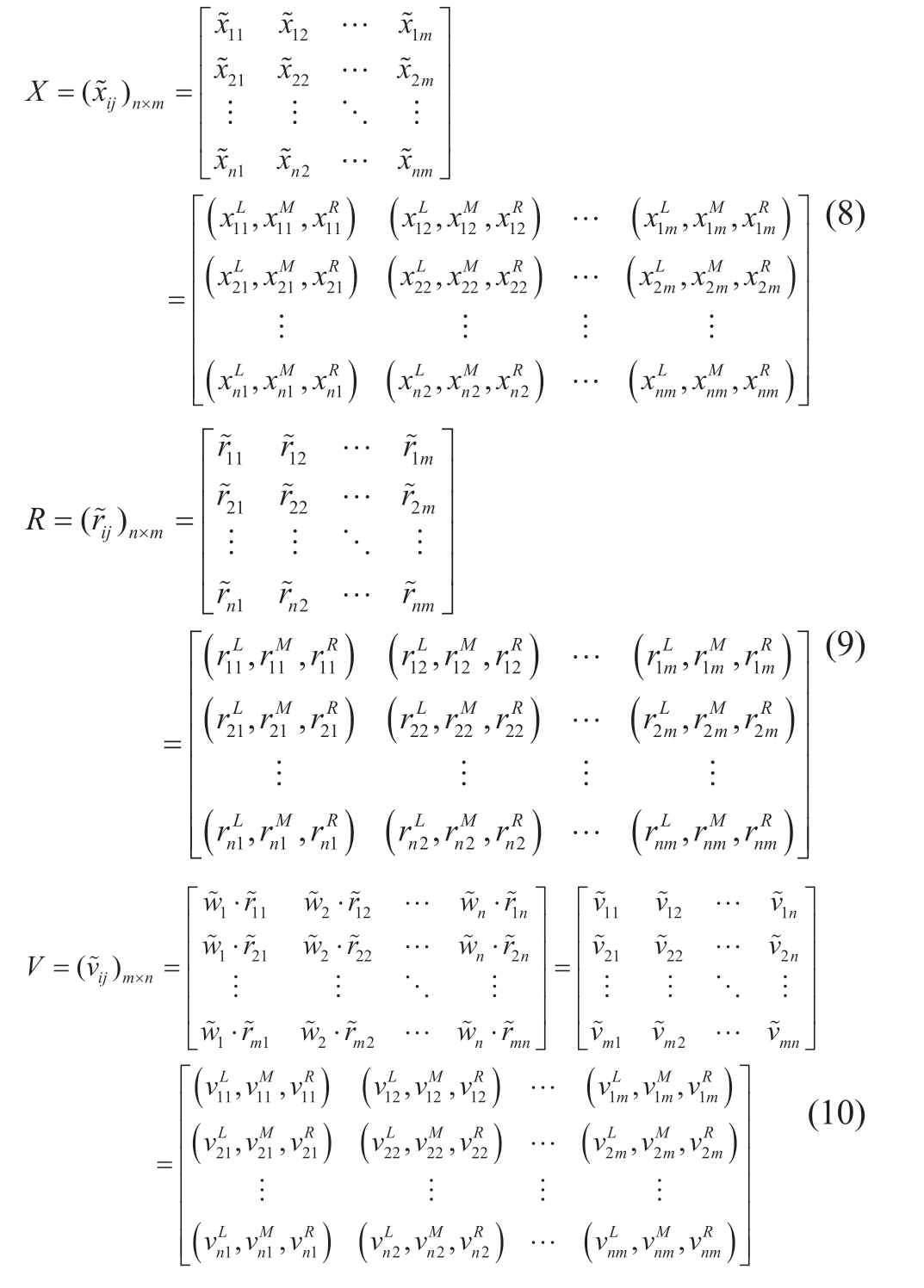
where X is the original decision-making matrix;R is the normalized decision-making matrix with efficiency and cost indices normalized using (11) and (12),respectively;and V is the weighted normalized decision-making matrix.
Efficiency indexes:
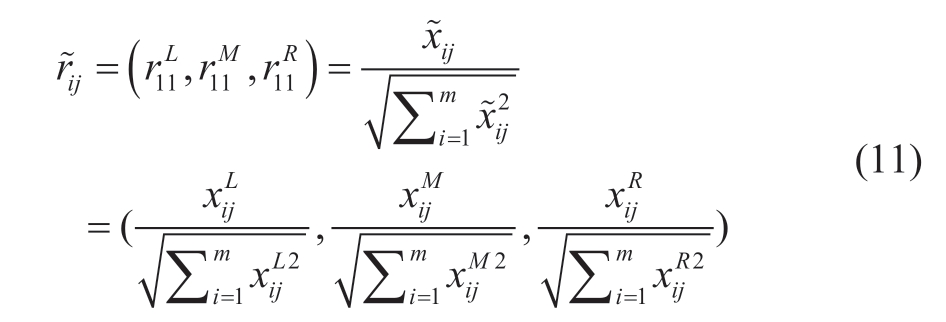
Cost indexes:
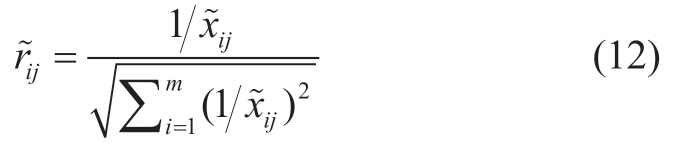
2) Calculation of efficiency and cost indices of alternative solutions

where the first k indices are efficiency indices and the remaining (n-k) indices are cost indices.
Equation (13) represents the sum of all the operational efficiency indices of the j-th electricity market,with a larger value indicating better operational performance of the electricity market.Equation (14) represents the sum of all cost indices of the j-th electricity market,with smaller values indicating better operational performance of the electricity market.
3) Calculation of the relative importance of alternative solutions
Defuzzification was performed on and
,followed by the calculation of the relative importance of the alternative solutions.
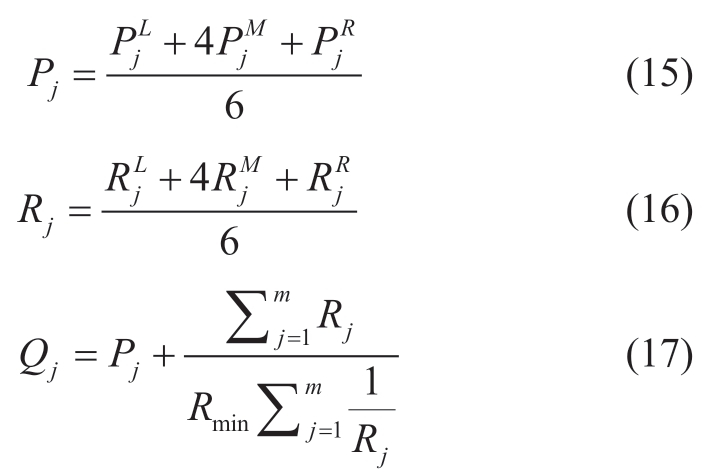
4) Calculation of alternative solution values

The alternative solutions were ranked according to their respective values.A larger value indicates a better operational performance of the electricity market.
2.3 Framework for operational assessment of the Chinese electricity markets
A novel fuzzy mixed MCDM method that combines fuzzy BWM and fuzzy COPRAS was proposed for the operational assessment of electricity markets.An index system for electricity market operational assessment was established based on expert consensus,following the purpose of electricity market development,with indices measuring the characteristics of the object of assessment in different dimensions.The proposed COPRAS method combines the objectivity of the membership of electricity market operational indices with the subjectivity of expert qualitative analysis,thereby improving the accuracy of the assessment.The assessment results can provide a reference for electricity-interested parties” or “stakeholders,trading organizations,and regulators.Figure 3 illustrates the framework of the proposed method for the electricity market operational assessment and its implementation procedure.
3 Empirical analysis
In August 2017,the National Development and Reform Commission and the National Energy Administration issued a Notice on Developing Experimental Electricity Spot Markets.The following regions/provinces were selected to develop the first batch of experimental electricity spot markets: southern China (beginning with Guangdong),west Inner Mongolia,Zhejiang,Shanxi,Shandong,Fujian,Sichuan,and Gansu.In this study,a comprehensive assessment of the following four provincial electricity markets was conducted: Zhejiang (A1),Guangdong(A2),Sichuan (A3),and Shandong (A4).The assessment procedure was as follows.This study employed the Lingo software (version 18.0) to program the Fuzzy BWM method,allowing the determination of indicator weights.In addition,MATLAB 2020b was used to program the Fuzzy COPRAS method,enabling the evaluation of the four selected provincial electricity markets.
3.1 Calculating the weights of electricity market operational assessment indexes
The weights of the indices were determined using the BWM method.Six experts were invited to form an evaluation expert group,including experts from electricity market trading institutions in four provinces and scholars engaged in research on electricity market trading from universities.First,the best and worst indices (designated as AB and AW,respectively) were determined based on expert consensus.Next,pairwise comparisons between the best index and other indices were performed by the expert group.Table 6 presents the results of the study.Subsequently,the vectors of pairwise comparisons with the best index are obtained,as shown in (19).
Table 6 Pairwise comparisons between the best index C1 and other indexes


Next,pairwise comparisons between the worst index and other indices were performed by the expert group.Table 7 presents the results of the study.Subsequently,the vectors of pairwise comparisons with the worst index are obtained,as shown in (20).
Table 7 Pairwise comparisons between the worst index (C3)and other indices
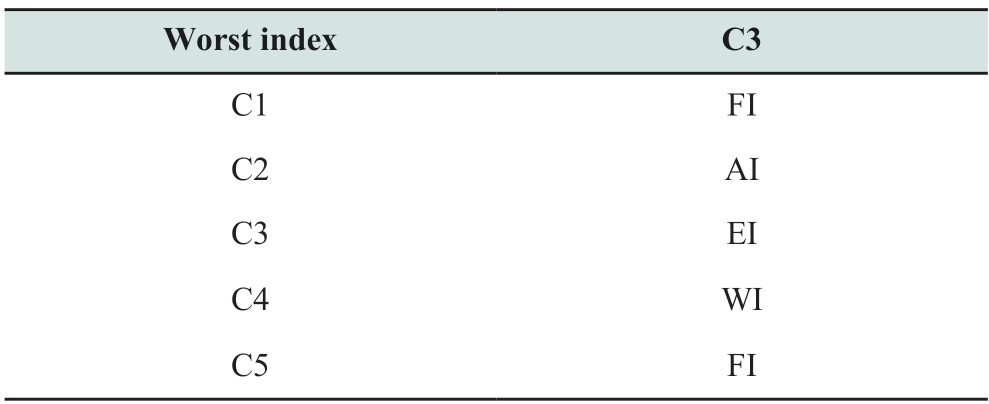
continue
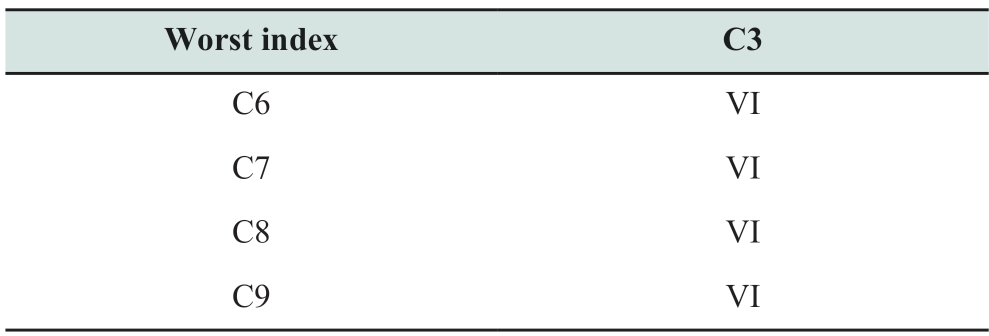

Finally,the weights of the indices were calculated,as shown in Fig.4.
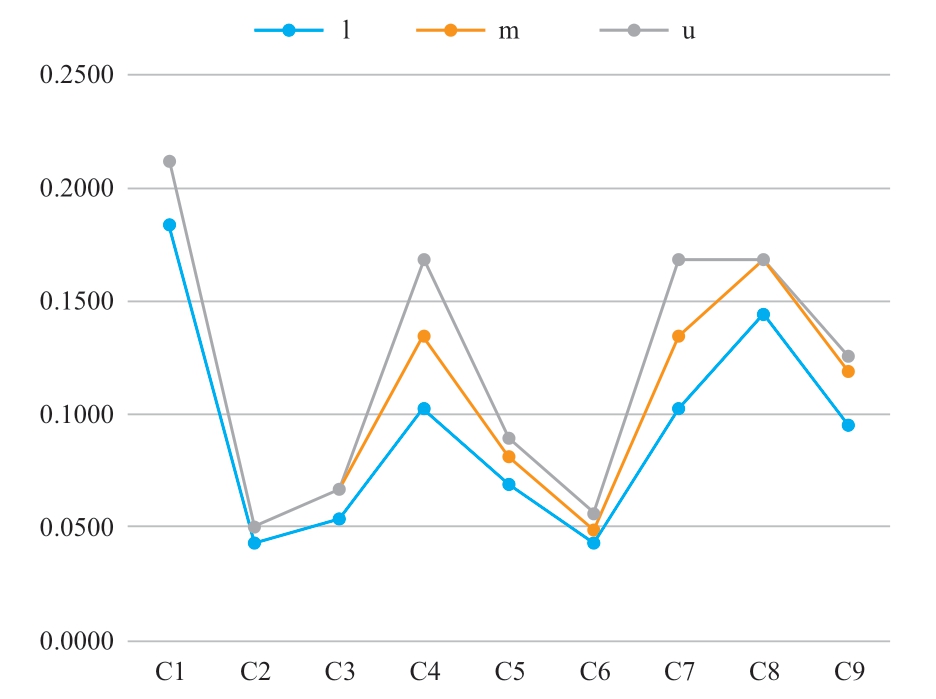
Fig.4 Weights of electricity market operational assessment indices
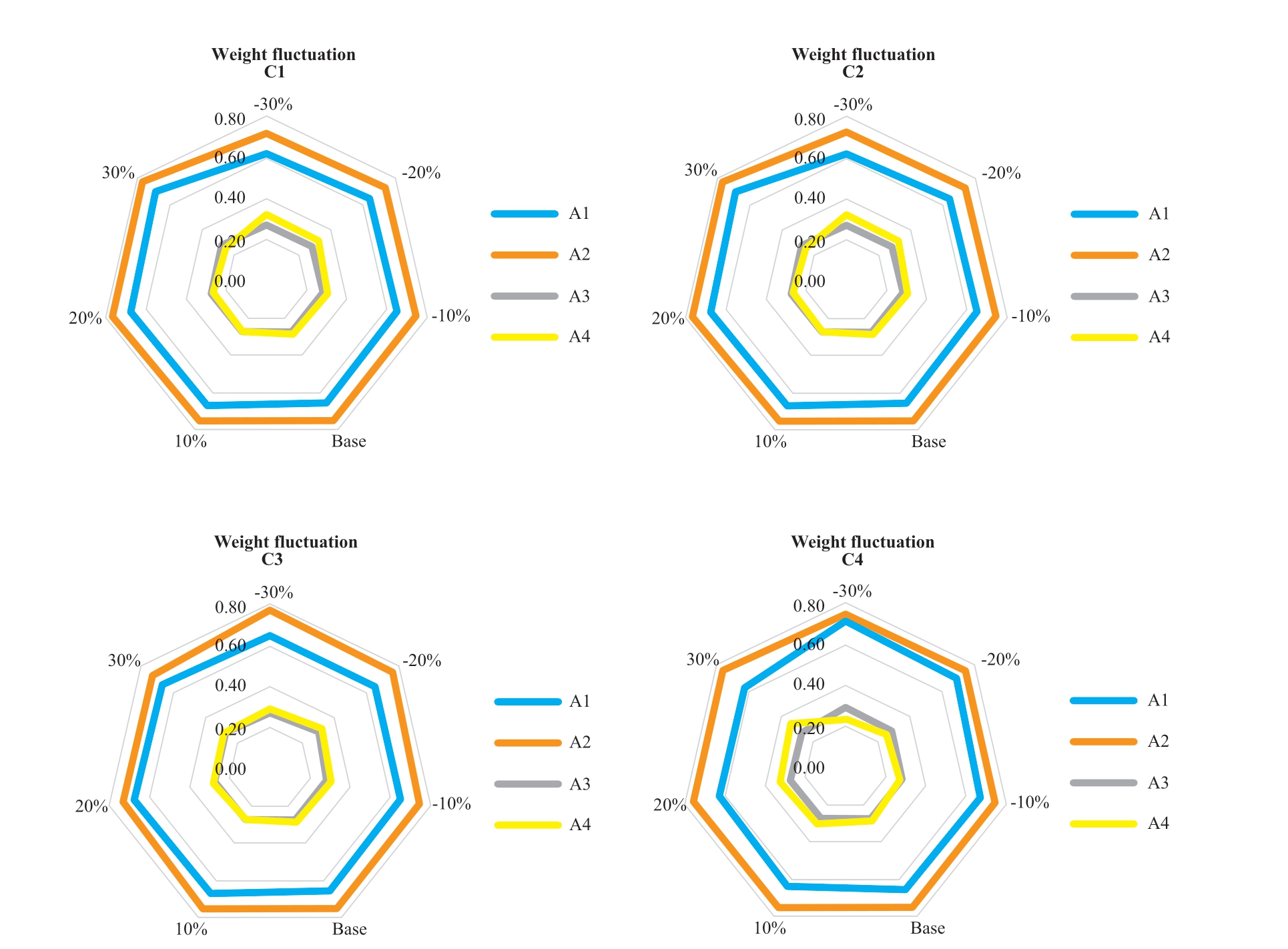
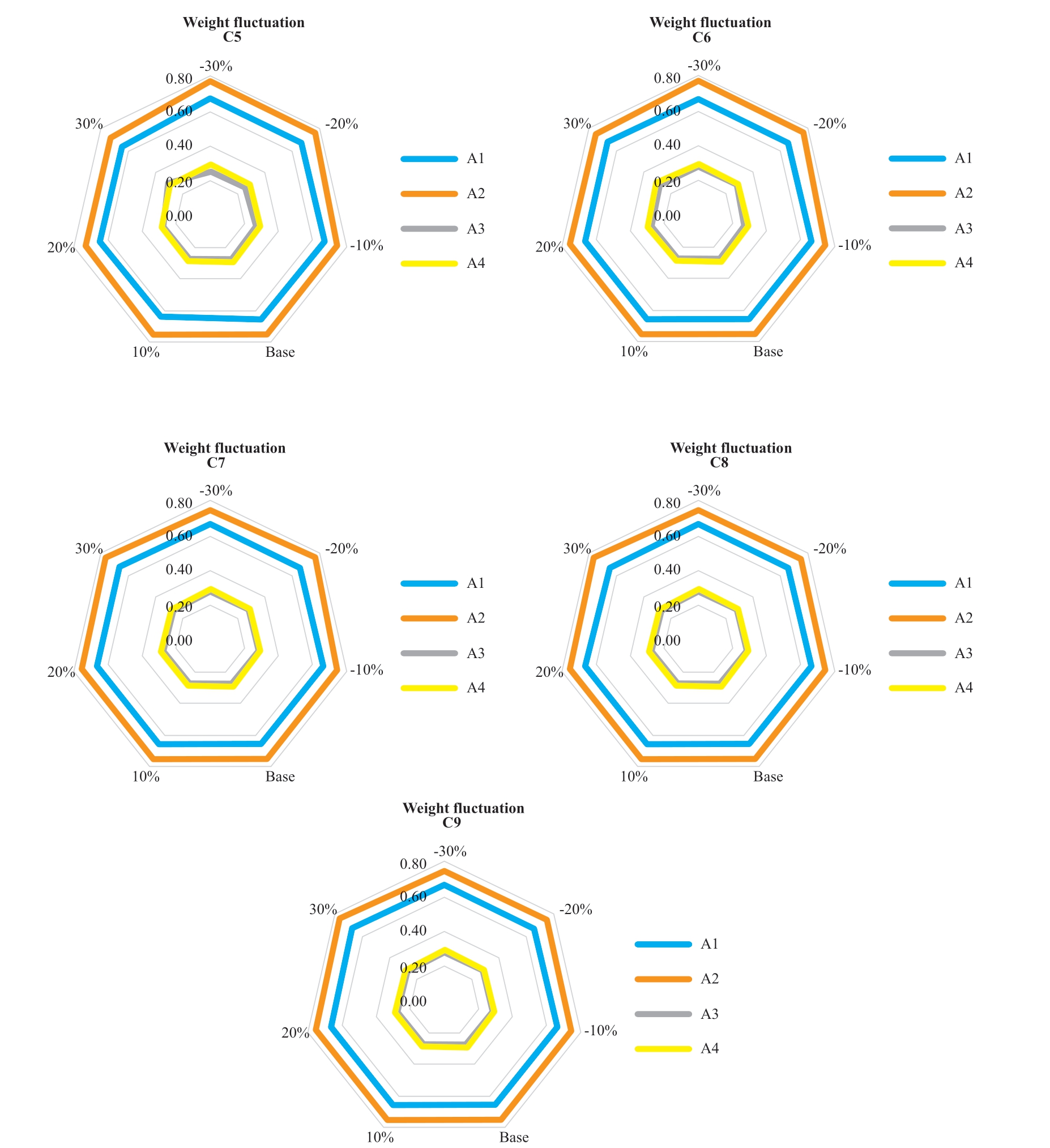
Fig.5 Results of sensitivity analysis
3.2 Comprehensive operational assessment of four electricity markets
The quantitative indices were assessed using original data collected from on-site surveys and data mining.The qualitative index was assessed using expert consensusbased qualitative judgment.The resulting numerical values of the indices were substituted into (8) -(18) to assess the overall operational performance of the four electricity markets,yielding (19).The results showed that Guangdong performed the best,followed by Zhejiang and Shandong.The Sichuan electricity market performed the worst.
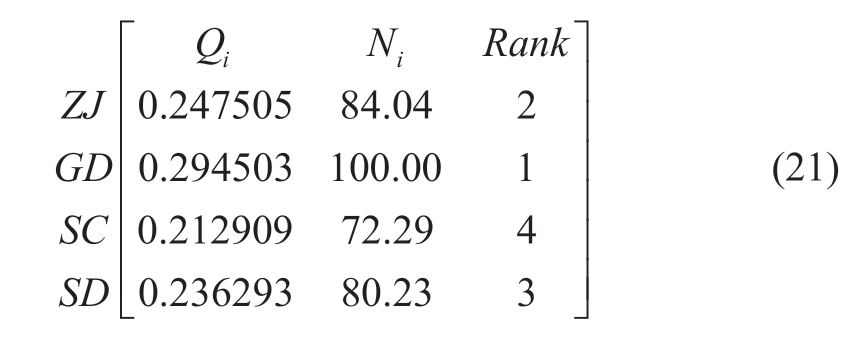
4 Discussion
In this study,a novel fuzzy MCDM method combining a fuzzy BWM and a fuzzy COPRAS method was proposed,considering nine indices (including the ratio of medium-and long-term contracts and the ratio of installed capacity of renewable energy) for electricity market operational assessment.This method was applied to the operational assessment of four provincial electricity markets (Zhejiang,Guangdong,Sichuan,and Shandong).The assessment results showed that the Guangdong electricity market performed the best.To better understand the applicability of the proposed fuzzy MCDM method to electricity market operational assessment,an in-depth analysis of the nine indexes and a sensitivity analysis were performed.The results are presented in the following sections.
4.1 Analysis of assessment results
An analysis of the data in Table 4 shows that the indices C1,C4,C7,and C8 had higher weights.This indicates that,for an electricity market to have a well-developed system and operate efficiently,a high ratio of medium-and longterm contracts is necessary to alleviate spot market trading pressure.In addition,fair competition among all participants and the absence of a monopoly on market trading are necessary to promote market efficiency and long-term coordinated development.Furthermore,the electricity market should be capable of preventing risks and coping with emergencies in a timely manner,thereby improving its resilience.
Equation (21) shows the rankings of the four provincial electricity markets,based on their operational performance.Guangdong performed best,followed by Zhejiang and Shandong.The Sichuan electricity market performed the worst.The assessment of the Guangdong electricity market using the fuzzy COPRAS method showed that indices C4 and C7 had higher positions than those of the other three provincial electricity markets.In addition,the Lerner index (C8) score was lower,indicating more competition in the Guangdong electricity market.Therefore,after considering the weights of the other relevant indices,the Guangdong electricity market ranked first.Further,to verify the effectiveness of the fuzzy BWM and fuzzy COPRAS methods for the operational assessment of electricity markets,a sensitivity analysis was performed,and the results are presented in Section 4.2.
4.2 Sensitivity analysis
To verify the robustness of the proposed fuzzy MCDM method for electricity market operational assessment,a sensitivity analysis was performed to assess the effects of the weights of the indices on the ranking of the electricity markets.The weights of the indices were reduced by 10%,20%,and 30%,and then increased by 10%,20%,and 30%,respectively.Figure 5 presents the resulting rankings for the electricity markets.
For example,as the importance of index C1 decreased,the total assessment scores of the Zhejiang,Guangdong,and Sichuan electricity markets decrease,whereas those of the Shandong electricity market increased.As the weight of index C1 increased,the total assessment scores of the Zhejiang,Guangdong,and Sichuan electricity markets increased,whereas those of the Shandong electricity market decrease.In addition,as the weight of index C1 increased,the total assessment score of the Sichuan electricity market exceeded that of the Shandong electricity market.However,among the four provincial electricity markets,Guangdong had the highest total assessment score.This was also the case when the weights of the other indices varied.This verifies that the proposed fuzzy MCDM method is robust and effective for the operational assessment of the electricity market.
4.3 Sorting consistency test
To verify the validity of the proposed model,we selected two other models to perform a sequencing consistency test [55,56],as shown in Table 8.The test indicators were as follows:
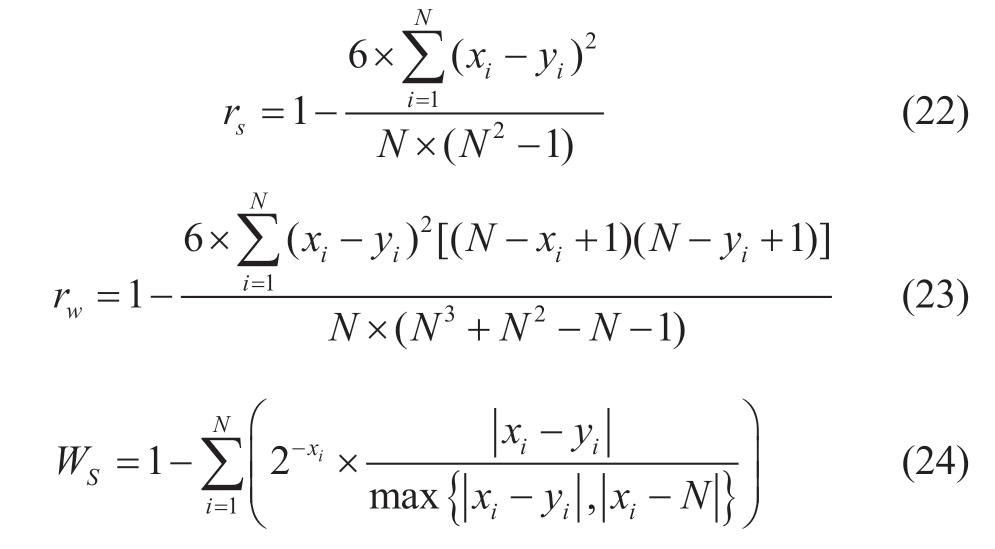
Table 8 Basic information of comparison model
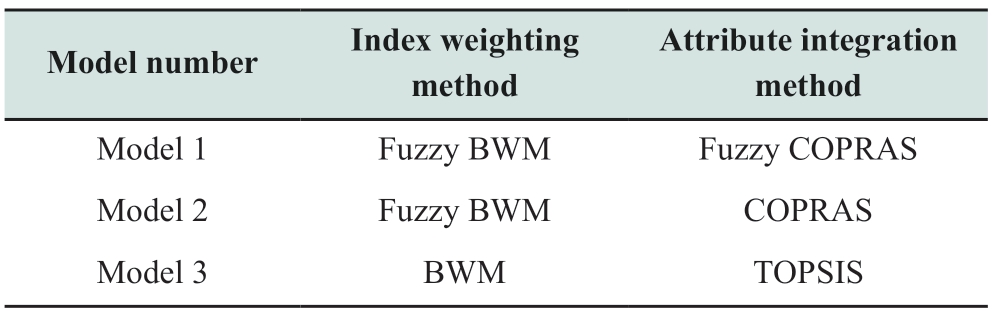
where rs,rw,and WS are the Spearman ranking correlation coefficient,weighted Spearman ranking correlation coefficient,and ranking correlation coefficient respectively,xi and yi are types kinds of sorting results,and N is the number of alternatives in the sort.The larger the correlation coefficient,the closer the sorting results of the two schemes.
By comparing the ranking results of the two models with those of the proposed model,the ranking consistency of the proposed models was verified,as shown in Table 9.
Table 9 Results of model sequencing consistency test
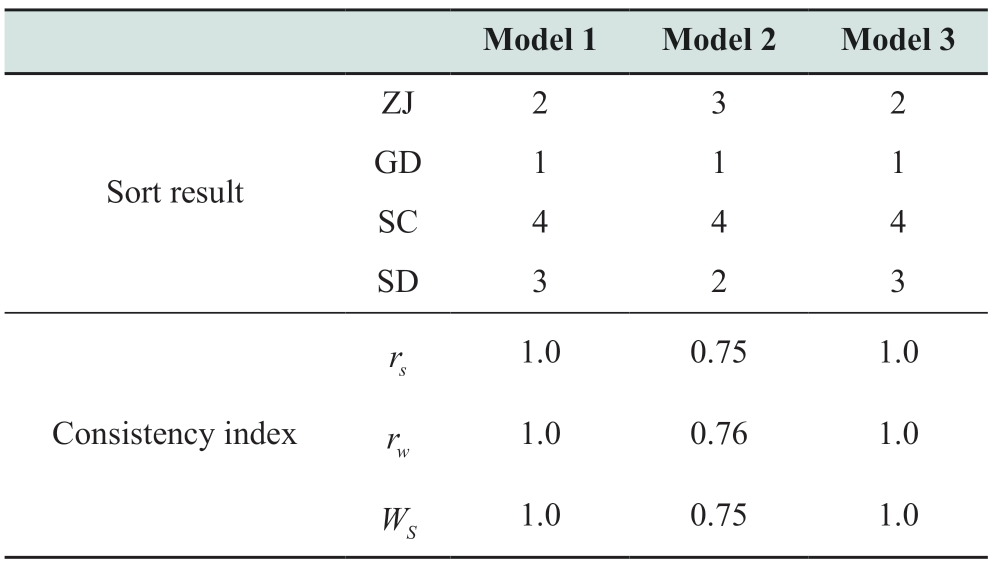
According to Table 9,in terms of the ranking results,the values of the two comparison models were the same as those of the first and last among all models,and the consistency index was greater than 0.75,indicating that the ranking results of the comparison model were highly consistent with those of the model proposed in this study,thus verifying the reliability of the ranking results of the proposed model.
5 Conclusion
Power systems are critical to the national economy and people’s livelihoods,and the safe and stable operation of power systems is a priority for the industry.The introduction of electricity markets with fierce competition has introduced several opportunities along with risks and challenges to market entities such as grid operators and power distributors.Maintaining safe and stable operation of the power industry in a market environment requires a well-developed operation management system and scientific risk management philosophy on the part of market entities.A comprehensive operational assessment of the electricity market provides an understanding of the current status of the design,efficiency,and coordinated development.Based on this,necessary measures can be adopted to maximize socioeconomic benefits.
In this study,we proposed an index system and a novel fuzzy MCDM method for the comprehensive operational assessment of electricity markets.This method combined a fuzzy BWM to determine the weights of the indices and a fuzzy COPRAS method to rank electricity markets according to their operational performance.The proposed method was applied to the operational assessment of four provincial electricity markets in China.The results showed that the Guangdong electricity market performed the best,followed by Zhejiang and Shandong electricity markets.The Sichuan electricity market performed the worst.In addition,four indices: the ratio of medium-and long-term contracts(C1),openness of trading markets (C4),ratio of reserve capacity (C7),and Lerner index (C8),had higher weights than the other indices,indicating that these four indexes had a greater impact on the comprehensive operational assessment of electricity markets and should receive priority attention during the operation of electricity markets.
In general,the assessment results showed that the proposed index system and model for electricity market operational assessment reflected the characteristics of electricity market operation well,considered the characteristics of all indices,and yielded results that reflected the overall operational status of the assessed electricity market.Our results have practical significance for the development of the Chinese electricity market.
This study had certain limitations.For instance,owing to data availability,only four provincial electricity markets were evaluated,and subjective weighting was used to select the weights of the indicators.In future research,we expect to obtain more construction data on provincial electricity markets to verify the effectiveness of the proposed model and to extract more experience with electricity market construction.It is also important for future research to improve the model by using a combination of subjective and objective methods to assign weights to indicators,thereby making the evaluation of electricity market construction more accurate.
Acknowledgments
We thank the editor and reviewers for their thorough review.
Declaration of Competing Interest
The authors declare no conflicts of interest.
References
[1] He J K,Li Z,Zhang X L,et al.(2020) Comprehensive report on China’s long term low carbon development strategies and pathways.China Journal of Population,Resources and Environment,30(11): 263-295
[2] Li Z,Chen S Y,Dong W J,et al.(2021) Feasible and affordable pathways to low-carbon power transition in China.Clean Coal Technology,27(2): 1-7
[3] Li Z,Chen S Y,Dong W J,et al.(2021) Low carbon transition pathway of power sector under carbon emission constraints.Proceedings of the CSEE,41(12): 3987-4001
[4] State Grid Energy Research Institute Co.,Ltd.(2020) China energy and power development outlook 2020.China Electric Power Press
[5] Zhang Y Z,Zhang N,Dai H C,et al.(2021) Model construction and pathways of low-carbon transition of China’s power system.Electric Power,54(3): 1-11
[6] Liu K C,He G X,Wang J Y,et al.(2018) Low carbon planning and economic analysis for China’s power sector towards 2030.Energy Conservation Technology,36(3): 263-269
[7] Chen Y,Tian C,Cao Y,et al.(2020) Research on peaking carbon emissions of power sector in China and the emissions mitigation analysis.Climate Change Research,16(5): 632-640
[8] Yu B Y,Zhao G P,An R Y,et al.(2021) Research on China’s CO2 emission pathway under carbon neutral target.Journal of Beijing Institute of technology (Social SciencesEdition),23(2): 17-24
[9] Wang K,Liu F M,Yin M J,et al.(2021) Research on China’s carbon emissions pathway under the 1.5 ℃ target.Climate Change Research,17(1): 7-17
[10] Wang L N,Peng T D,Xiang Z J,et al.(2021) Analysis of China’s energy transition pathways under the goal of carbon neutrality.International Petroleum Economics,29(1): 2-8
[11] Jenkins J D,Luke M,Thernstrom S (2018) Getting to zero carbon emissions in the electric power sector.Joule,2(12): 2498-2510
[12] Wadn G,Li L,Wang Z,et al.(2022) Mechanism design for ex-ante electric quantity security analysis adapted to largescale medium and long-term market trading.Electric Power Engineering Technology,41(1): 34-39
[13] Ren J,Zhou X,Xue C,et al.(2022) Spot market joint clearing mode with both sides of generation and customer participating in peak regulation.Electric Power Engineering Technology,41(1):26-33
[14] Davis S J,Lewis N S,Shaner M,et al.(2018) Net-zero emissions energy systems.Science,360(6396): eaas9793
[15] Oecd,Agency N E (2012) Nuclear energy and renewables:System effects in low-carbon electricity systems.OECD Publishing,Paris,https://doi.org/10.1787/9789264188617-en
[16] Cheng X,Lv X,Li X S et al.(2023) Market power evaluation in the electricity market based on the weighted maintenance object.Energy,284: 129294
[17] Liu L,Chen X,Li D,et al.(2023) Evaluation method for assessing the auxiliary service transaction mechanism in regional power markets.Global Energy Interconnection,6(2): 234-248
[18] Fang B,Qiu W,Wang M,et al.(2022) Evaluation index system of shared energy storage market towards renewable energy accommodation scenario: A China’s Qinghai province context.Global Energy Interconnection,5(1):77-95
[19] Lin X S,Huang T,Bompard E et al (2023) Ex-ante market power evaluation and mitigation in day-ahead electricity market considering market maturity levels.Energy,278: 127777
[20] Lee Y Y,Baldick R,Hur J (2011) Firm-based measurements of market power in transmission-constrained electricity markets.IEEE Transactions on Power Systems,26(4): 1962-1970
[21] Yang S,Ma L,Fan M H,et al.(2019) Research on evaluation index system for power market in China.IOP Conference Series:Earth and Environmental Science,267(4): 042045
[22] Cui J R,Li Y T,Lin Z M,et al.(2022) Multi-dimensional evaluation of power market based on multiple attribute decision making [J].Energy Reports,8: 59-65
[23] Song D W,Yang M,Bai H K,et al.(2019) A comprehensive evaluation model of power market operation efficiency based on extension cloud theory.Energy Procedia,156: 302-309
[24] Xie J D,Wang S Y,Zhou X M,et al.(2021) Credit evaluation method of generating companies considering the market behavior in China electricity market [J].Energy Science &Engineering,9(9): 1554-1567
[25] Liu J,Liu W D,Jin L S,et al.(2020) A performance evaluation framework of electricity markets in China.2020 5th Asia Conference on Power and Electrical Engineering (ACPEE).Chengdu,China,IEEE,1043-1048
[26] Streimikiene D,Siksnelyte I (2016) Sustainability assessment of electricity market models in selected developed world countries.Renewable and Sustainable Energy Reviews,57(3): 72-82
[27] Li S,Niu D X,Wu L F (2018) Evaluation of energy saving and emission reduction effects for electricity retailers in China based on fuzzy combination weighting method.Applied Sciences,8(9): 1564
[28] Nuclear E A (2019) The costs of decarbonization: System costs with high shares of nuclear and renewables.OECD Publishing,Paris
[29] National Development and Reform Commission,Ministry of Construction (2006) Economic Evaluation Methods and Parameters of Construction Projects.China Planning Press
[30] Chen S Y,Liu P,Li Z (2020) Low carbon transition pathway of power sector with high penetration of renewable energy.Renewable and Sustainable Energy Reviews,130: 109985
[31] China Electricity Council (2020) Annual development report of China’s electric power industry 2020.China Construction Industry Press: China Power Enterprise Management
[32] Wang Y,Su X L,Qi L et al (2019) Feasibility of peaking carbon emissions of the power sector in China’s eight regions:Decomposition,decoupling,and prediction analysis.Environmental Science and Pollution Research,26(28): 29212-29233
[33] Zhang W R,Yuan J H (2021) China’s coal power sector stranded assets under the 2 ℃ global carbon constraint.Climate Change Research,17(1): 36-44
[34] Kan X M,Hedenus F,Reichenberg L (2020) The cost of a future low-carbon electricity system without nuclear power-the case of Sweden.Energy,195: 117015
[35] Luh S,Budinis S,Giarola S,et al.(2020) Long-term development of the industrial sector -Case study about electrification,fuel switching,and CCS in the USA.Computers &Chemical Engineering,133: 106602
[36] Zhang H (2019) Comprehensive analysis of power cost.Energy,10: 63-68
[37] Ziegler M S,Mueller J M,Pereira G D,et al.(2019) Storage requirements and costs of shaping renewable energy toward grid decarbonization.Joule,3(9): 2134-2153
[38] Tang B J,Li R,Yu B Y,et al.(2018) How to peak carbon emissions in China’s power sector: A regional perspective.Energy Policy,120: 365-381
[39] Zhang N,Xing L,Lu G (2018) Prospects and challenges of medium and long term energy and power transformation in China.China Power Enterprise Management,13: 58-63
[40] Jiang K J,Zhuang X,He C M,et al.(2016) China’s low-carbon investment pathway under the 2 ℃ scenario.Advances in Climate Change Research,7(4): 229-234
[41] Hou W W,Allinson G,MacGill I,et al.(2014) Cost comparison of major low-carbon electricity generation options: An Australian case study.Sustainable Energy Technologies and Assessments,8:131-148
[42] Wang Z W,Lu X,Diao R S,et al.(2020) Deep-reinforcementlearning based autonomous control and decision making for power systems.Electric Power Engineering Technology,39(6):34-43
[43] Zhao J B,Zhang S C,Liao S W (2020) Analysis and reflection for the rotating outages in mid-August 2020 in California.Electric Power Engineering Technology,39(6): 52-57
[44] Chen Y X,Cao X D,Wang J,et al.(2020) Integrated demand response behavior of integrated energy system.Electric Power Engineering Technology,39(6): 89-97
[45] Fu X,Yan H,Li F C,et al.(2020) Influence of energy storage power station on the power purchase characteristics of power grid.Electric Power Engineering Technology,39(6): 98-103
[46] Zhou J S,Wang C,Xiao X L et al (2020) Optimal modeling of integrated energy demand response under time-shared electricity price [C].2020 15th IEEE Conference on Industrial Electronics and Applications (ICIEA).Kristiansand,Norway.IEEE,PP:1214-1219
[47] Fan C,Yao J G,Zhang Q B,et al.(2020) Reflection and analysis for oscillation of the blackout event of 9 August 2019 in UK.Electric Power Engineering Technology,39(4): 34-41
[48] Shu G D,He P P,Ma R (2020) Multi-time scale unit combination method considering precision characteristics of wind power and solar power forecasting.Electric Power Engineering Technology,39(3): 78-83
[49] Wang Y,Zhang N,Kang C Q,et al.(2019) Electrical consumer behavior model: Basic concept and research framework.Transactions of China Electrotechnical Society,34(10): 2056-2068
[50] Li J L,Niu M,Zhou X C,et al.(2020) Energy Storage capacity planning and investment benefit analysis of micro-energy system in energy interconnection.Transactions of China Electrotechnical Society,35(4): 874-884
[51] Feng Y,Ying Z F,Yan J H (2021) Optimized operation of energy storage in a multi-energy complementary micro-energy network considering carbon emission cost.Power System Protection and Control,49(8): 92-99
[52] Chen H H,Mao W L,Zhang R F,et al.(2021) Low-carbon optimal scheduling of a power system source-load considering coordination based on carbon emission flow theory.Power System Protection and Control,49(10): 1-11
[53] Zhang L H,Dai G Y,Nie Q Y,et al.(2020) Economic dispatch model of virtual power plant considering electricity consumption under a carbon trading mechanism.Power System Protection and Control,48(24): 154-163
[54] Zhu Y,Lan Z B,Wei Z,et al.(2019) Research on optimal operation of wind-PV-ES complementary system considering carbon emission cost.Power System Protection and Control,47(10): 127-133
[55] Sałabun W,Wątróbski J,Shekhovtsov A (2020) Are MCDA methods benchmarkable? A comparative study of TOPSIS,VIKOR,COPRAS,and PROMETHEE II methods.Symmetry,12(9): 1549
[56] Kizielewicz B,Wątróbski J,Sałabun W (2020) Identification of relevant criteria set in the MCDA process—Wind farm location case study.Energies,13(24): 6548
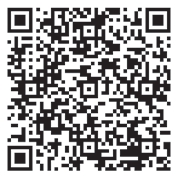
Scan for more details
Received: 22 November 2023/ Accepted: 5 May 2024/ Published: 25 December 2024
Dongwei Li
lidongwei@cec.org.cn
Weijie Wu
wuweijie@gd.csg.cn
Hui Sun
13925410199@139.com
Yixin Li
liyixin@gd.csg.cn
Yining Zhang
zhangyining@gd.csg.cn
2096-5117/© 2024 Global Energy Interconnection Group Co.Ltd.Production and hosting by Elsevier B.V.on behalf of KeAi Communications Co.,Ltd.This is an open access article under the CC BY-NC-ND license (http://creativecommons.org/licenses/by-nc-nd/4.0/).
Biographies
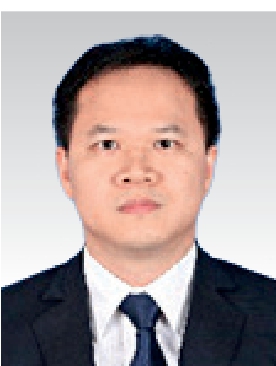
Weijie Wu received his Master’s degree from Tsinghua University,in 2004.He is working at the Guangdong Power Grid Planning and Research Center,Guangzhou.His research interests include power system planning and power system analysis.
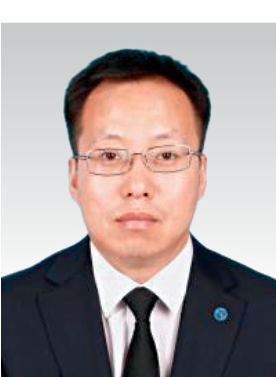
Dongwei Li received his Master’s degree from North China Electric Power University,in 2007.He is working at the CEC Technical &Economic Consulting Center of Power Construction,Beijing.His research interests include Energy research.
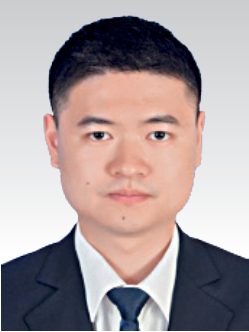
Hui Sun received his Master’s degree from North China Electric Power University,in 2013.He is working at the Guangdong Power Grid Planning and Research Center,Guangzhou.His research interests include power system planning and power system analysis.
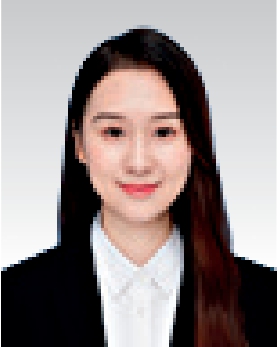
Yixin Li received her Master’s degree from Wuhan University,in 2019.She is working at the Guangdong Power Grid Planning and Research Center,Guangzhou.Her research interests include power system planning and energy planning.
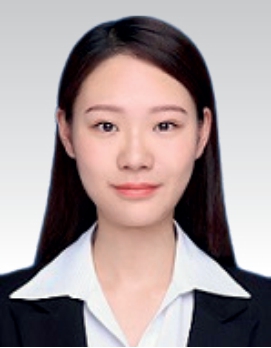
Yining Zhang received her Ph.D.degree from Zhejiang University,in 2019.She is working at the Guangdong Power Grid Planning and Research Center,Guangzhou.Her research interests include power system planning and energy planning.
(Editor Zedong Zhang)