0 Introduction
The new round of power system reform in China has entered a critical period,and the originally underdeveloped,immature,and unsound auxiliary service market has been unable to meet the growing demand for a variety of auxiliary services from relevant market participants [1].The regional power market is an arena for power trading between provincial (city) power grids,mainly composed of inter-provincial (city) power grid buyers,sellers,and direct dispatching of power plants by regional power grids.The auxiliary market must be reformed and adjusted in a timely and effective manner,or the security and reliability of the power system will be unprecedentedly impacted.To avoid inadequate power supply,a systematic evaluation method for the auxiliary service trading mechanism of the regional power market [2] is essential for assessing power market simulation models objectively and scientifically,as is the adoption of diversified and scientific methods to adjust and improve the deficiencies revealed by the experimental results.
The establishment of a seamless and functional transaction mechanism in regional power market transactions in China is crucial.At present,minimal research has been conducted regarding the evaluation of the regional power market auxiliary service transaction mechanism; however,there is considerable research on the power market mechanism and regional market coordination optimization.In terms of research on the regional market mechanism,the authors of reference [3] analyzed regional market characteristics,proposing an organizational model that is conducive to transitioning to a regional unified market and is decoupled between provinces.The authors of reference [4,5] designed a monthly matchmaking transaction bidding mechanism for regional power markets considering transmission price,transmission loss discount,and environmental protection factors.To address the problem of competitive transactions in regional power markets.This research work is expected to provide a theoretical basis and reference for the design of the bidding trading mechanisms in China’s regional power market.The authors of reference [6] analyzed the transaction mechanism of a layered regional power market,and proposed several integration schemes,from a layered regional power market to a unified regional power market.In terms of optimizing regional market coordination,the authors of reference [7]quantitatively investigated the characteristics of common and unified regional markets under conditions of demand elasticity and demand rigidity.The authors concluded that the resource allocation efficiency of unified regional markets is higher than that of common regional markets.The authors of reference [8] based on the current peakregulation auxiliary service market in the northwestern China region,a coordinated source-grid-load flexible interaction framework was proposed according to the load characteristics of the northwest grid and the accommodation of renewable energy demand.The authors of reference [9]analyzed the challenges of establishing a unified regional power market in northwest China by examining the microenvironment,market model,and price mechanism and proposed corresponding suggestions.The authors of reference [10] examined the pricing mechanism of a regional power market and elaborated on the principles of selecting different pricing mechanisms.The research results show that an organizational model of a decoupled provincial regional power market reduces market structure complexity and can well reflect market fairness.The authors of reference [11] investigated the balance between competition and coordination,demonstrating that the cost of poor coordination caused by decentralization could be enormous.Policies must prioritize market mechanisms and regulatory governance structures to ensure better coordination.
In addition,in terms of power market evaluation methods,the authors of reference [12] developed a Takagi–Sugeno–Kang fuzzy model that considers the uncertainty and nonlinearity of the power market and obtains realistic stability results.The study applied stability and instability tests to the power market under different market conditions as a basis for analyzing the problems related to the power market stability.The authors of reference [13] analyzed and evaluated power market accommodations based on the retention period and the strength of market power,measuring these two directions with an average value of the market power index and Lerner index.The authors of reference [14] also used the multilevel fuzzy comprehensive evaluation method.Taking Guangdong’s medium- and long-term power market as an example,the authors constructed a comprehensive hierarchical power market evaluation index for analysis.The authors of reference[15] established a set of evaluation indices that are suitable for evaluating the efficiency,fairness,and development of the current Guangdong power market,and analyzed the actual circumstances of the Guangdong power market and information disclosure.The authors of reference [16]applied power market value evaluation indicators to the United States Midcontinent Independent System Operator power market,including reliability improvement benefits,power-dispatching benefits,regulation benefits,and related concerns.
Although numerous studies have been conducted to evaluate the auxiliary power service trading mechanism,some deficiencies remain,including a lack of quantitative indicators,failure to consider the bid-winning rate,and providing a simple approach to empowerment.Furthermore,studies evaluating the auxiliary power service trading mechanism in regional power markets are rare.In this regard,we first conducted research from the perspectives of the various basic demands of the auxiliary service market,market entities,market products,and auxiliary services trading and bidding to identify the key influencing factors of auxiliary service trading mechanism design in regional power markets.Second,combined with the identified key influencing factors,we constructed an auxiliary service trading evaluation index for assessing regional power markets,including evaluation of auxiliary service trading development,reasonable compliance,the benefits and welfare of the trading mechanism,and other relevant considerations.Third,to analyze the scientificity and rationality of the auxiliary service trading evaluation index,we established an evaluation model for assessing the regional power market.Using the interval value quantitative estimation method and nonlinear dimensionless processing to preprocess the index data,the analytic network process(ANP) [17],RSM [18],and the least-squares method (LSM)[19] were selected to optimize the combination weight assignment,the gray correlation TOPSIS method [20]was applied to comprehensively evaluate auxiliary service trading in the regional power market.Finally,the application of the proposed evaluation method was briefly analyzed to examine four regional power markets in China and evaluate the effectiveness of current market construction in different regions and provide suggestions for future market construction.
1 Evolution and key issues of power market in typical countries
1.1 European Unified Power Market
(1) Market development status
Currently,the European unified power market has achieved the coupling of 23 national day-ahead markets and 14 national day-ahead markets,and is gradually promoting the coupling of day-ahead and day-ahead markets in more countries.
(2) Market evolution process
In 1993,the European Union proposed the reform goal of establishing a unified power market,opening the evolutionary process of power market-oriented trading and market integration construction.As shown in Fig.1,the evolution of the European unified power market has undergone three stages of development: from national power markets to regional power markets,and then to transnational power markets.In each stage of development,the EU first requires member states to open their transmission networks through the formation of corresponding energy bills,ensuring the fairness of the transmission environment to various market entities,and thereby providing free-flowing transmission channel support for market transactions.Meanwhile,in the procfree-flowingess of gradually integrating and expanding the market scope,the EU has established institutions such as the Agency for Cooperation of Energy Regulators (ACER) and the European Network of Transmission System Operators for Power (ENTSO-E) to uniformly coordinate and manage market transactions and system operations,Finally,in 2018,the day-ahead market coupling of the six regional power markets except for the Southeast European power market was achieved,and the integration of the day-ahead market was actively promoted in the future.
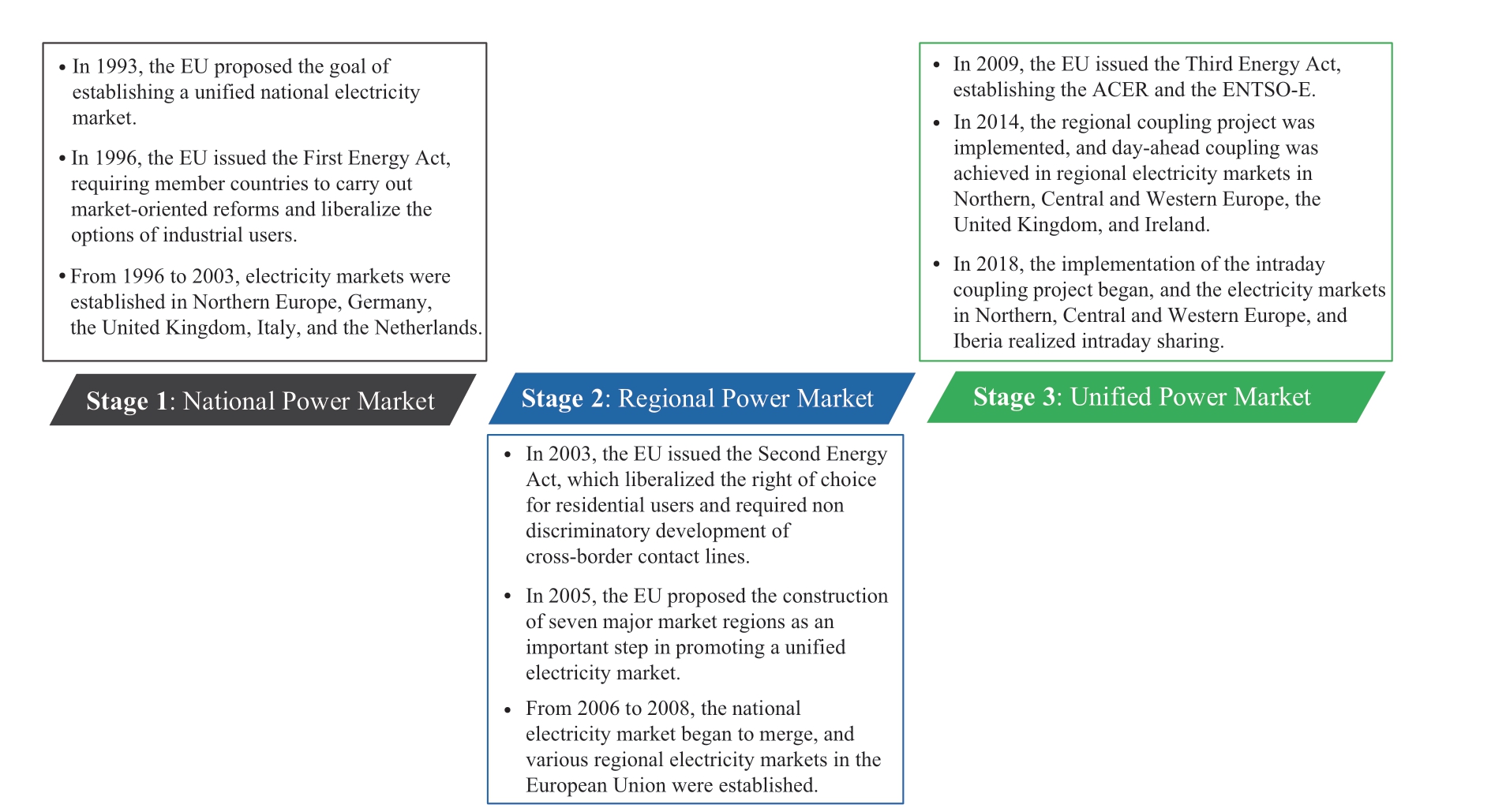
Fig.1 Evolution of European integrated power market
1.2 US Regional Power Market
(1) Market development status
Currently,the US power market mainly includes 10 regional power markets.The Midwest Power Market(MISO),which has the widest trading range,has covered 15 states in the central United States and parts of Canada.
(2) Market evolution process
The reform of power marketization in the United States began with the gradual liberalization of free competition on the power generation side in various regions.As shown in Fig.2,the construction of the regional power market in the United States has gone through three stages of development:the regional power market model of generation side competition,the regional power market model of the centralized organization by independent system operators(ISO),and the regional power market model of the centralized organization by regional transmission operators(RTOs).Similar to Europe,the United States first requires the effective separation of traditional vertical integration institutions in the form of bills or decrees at each stage of development,and ensures non-discriminatory opening of the transmission network.Unlike Europe,the integration of the US market has been closely focused on its longstanding system operation mode of joint dispatching by local power companies.To establish four major regional power markets,the evolution of the market from a regional power market to a regional power market has been achieved through the establishment of ISO and the gradual evolution into RTO through the transfer of dispatching rights.In addition,in response to the current situation of different regional market organizations,the United States has introduced a standard market design framework (SMD) for regional power markets based on the market design of the Pennsylvania New Jersey Maryland power market (PJM).The introduction of SMD has promoted the development of regional power market models towards uniformity,providing effective technical support for the consolidation and expansion of regional power markets.
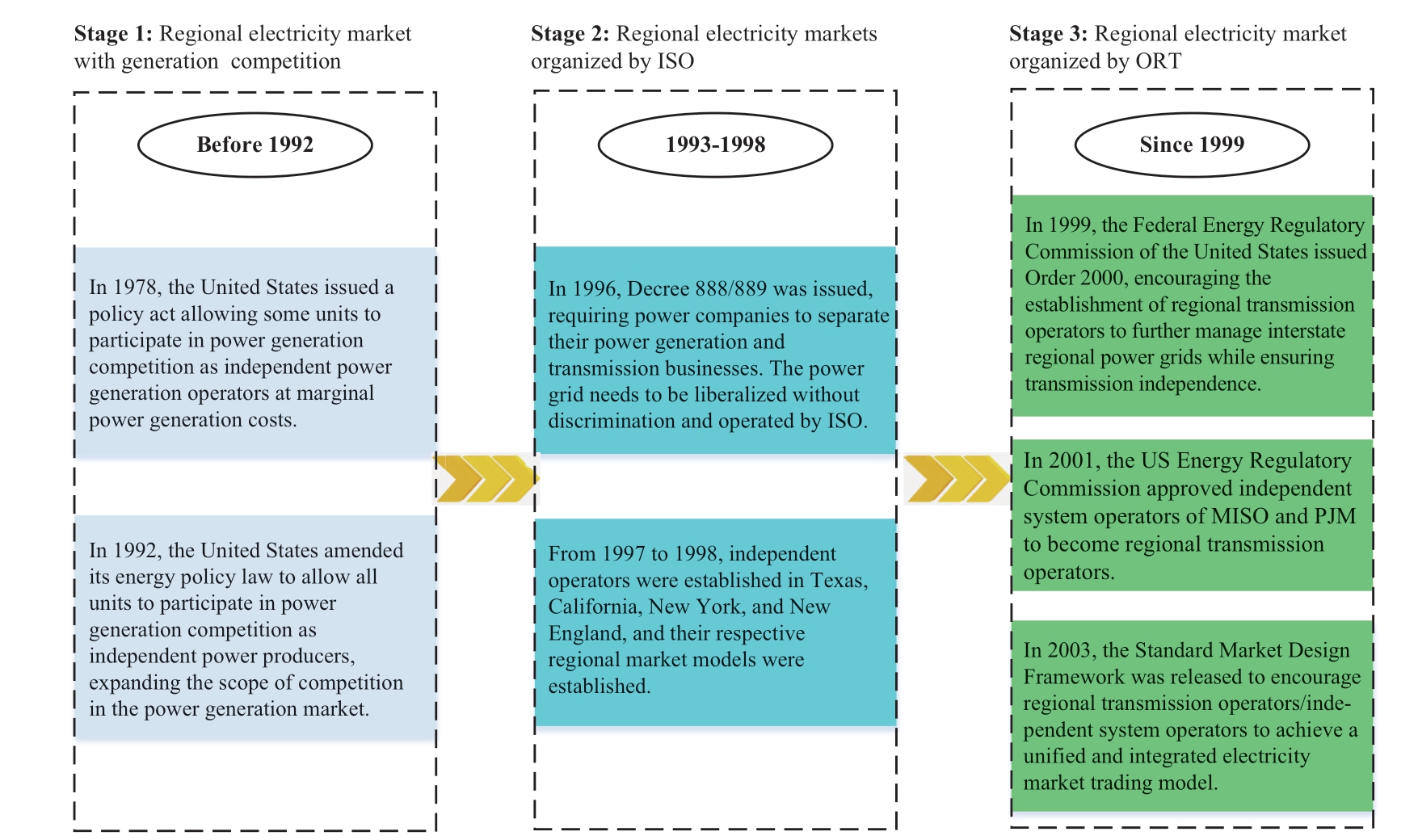
Fig.2 Evolution of regional power market in the U.S.
1.3 Development of China’s power market
(1) Market development status
Currently,The development of China’s power market starts with a “two-level operation” of inter-provincial and intra-provincial markets,in which the inter-provincial market mainly meets the demand for large-scale optimal allocation of resources,while the intra-provincial market mainly ensures the optimal allocation of resources within the province,the balance between power supply and demand,and the order of safe power supply.
(2) Inter provincial regional power market
Currently,China’s inter-provincial regional market is mainly dominated by regional medium and long-term contract transactions,while conducting incremental spot transactions aimed at the consumption of renewable energy within the region.Taking the inter-provincial regional market organized by the Beijing Electric Power Trading Center as an example,medium and long-term contract transactions include regional power energy transactions and regional power generation rights/contract transactions,which are divided into multiyear,annual,monthly,weekly,and multi-day transactions according to the transaction cycle.The transaction organization methods include bilateral negotiations,centralized transactions,and so on.Regional incremental spot trading includes day-ahead and intraday trading,mainly to meet the needs of renewable energy transmission and the balance between power supply and demand within the region.The transaction is organized based on the regional medium and long-term transaction contract curve.With the gradual improvement of the inter-provincial regional market,the transaction volume is also expanding.
1.4 Regional power market differences in typical countries
Although the scope of market competition in the power markets of Europe,the United States,and China is constantly expanding in the process of development,the construction goals and development paths of the power markets in these countries are not the same.Therefore,this section discusses some external conditions for market construction,including political background,power grid architecture,and technical conditions; The internal conditions for market construction,including driving forces and organizational structures,have been compared and analyzed in the United States,Europe,and China’s power markets,as shown in Table 1.
Table 1 Comparison of the construction conditions of typical countries
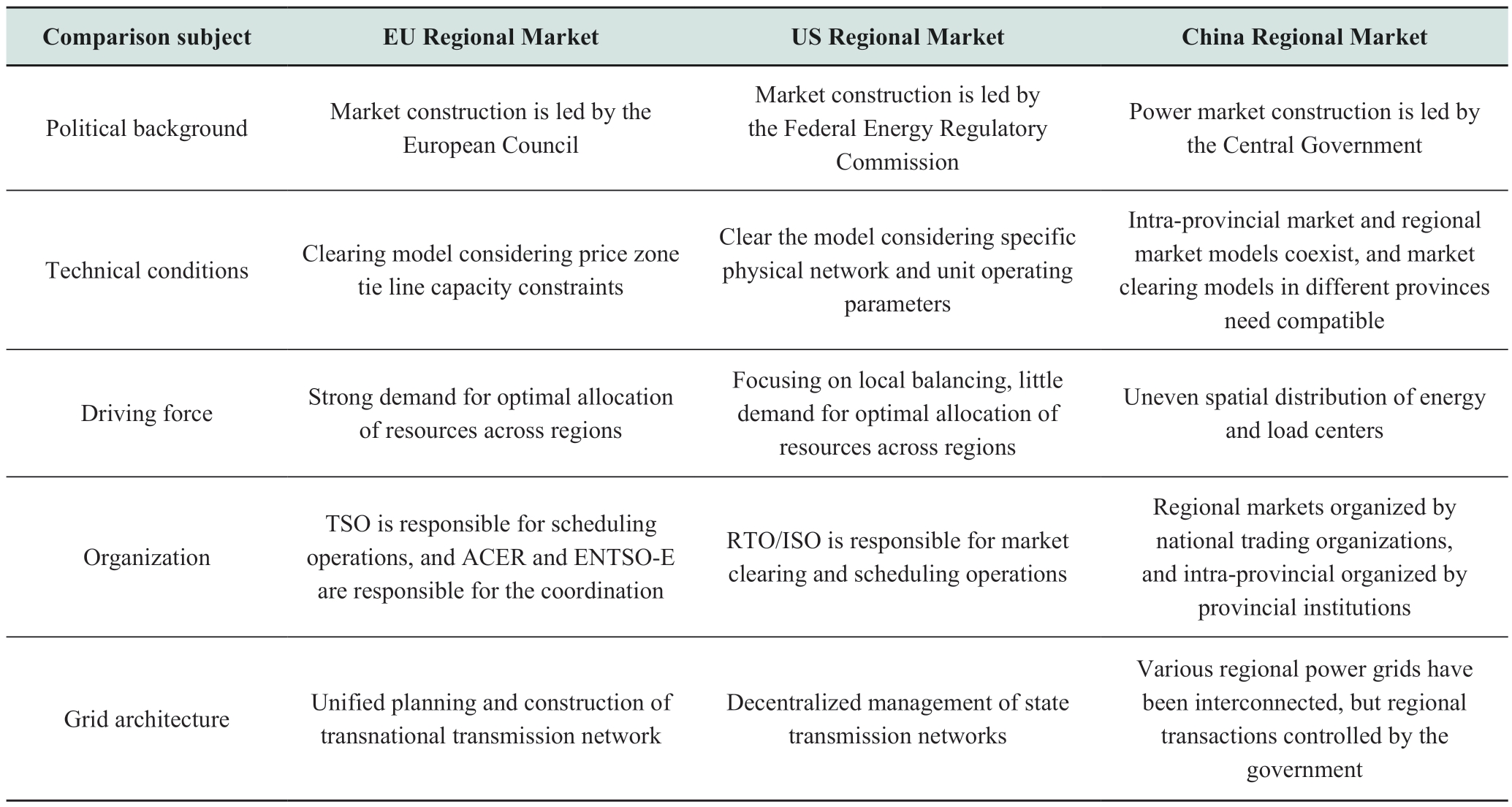
2 Constructing an evaluation index for the auxiliary service trading mechanism in regional power markets
2.1 Analysis of the influencing factors of auxiliary service in regional power markets
Auxiliary service in power markets range from the power production end to the power user end,and primarily refers to services provided to ensure the safe and stable operation of the power system [21] and maintain and improve power quality,in addition to the regular production,transmission,and use of power.A scientific and seamless market-oriented mechanism for auxiliary power services has begun to take shape in China,as shown in Fig.3.
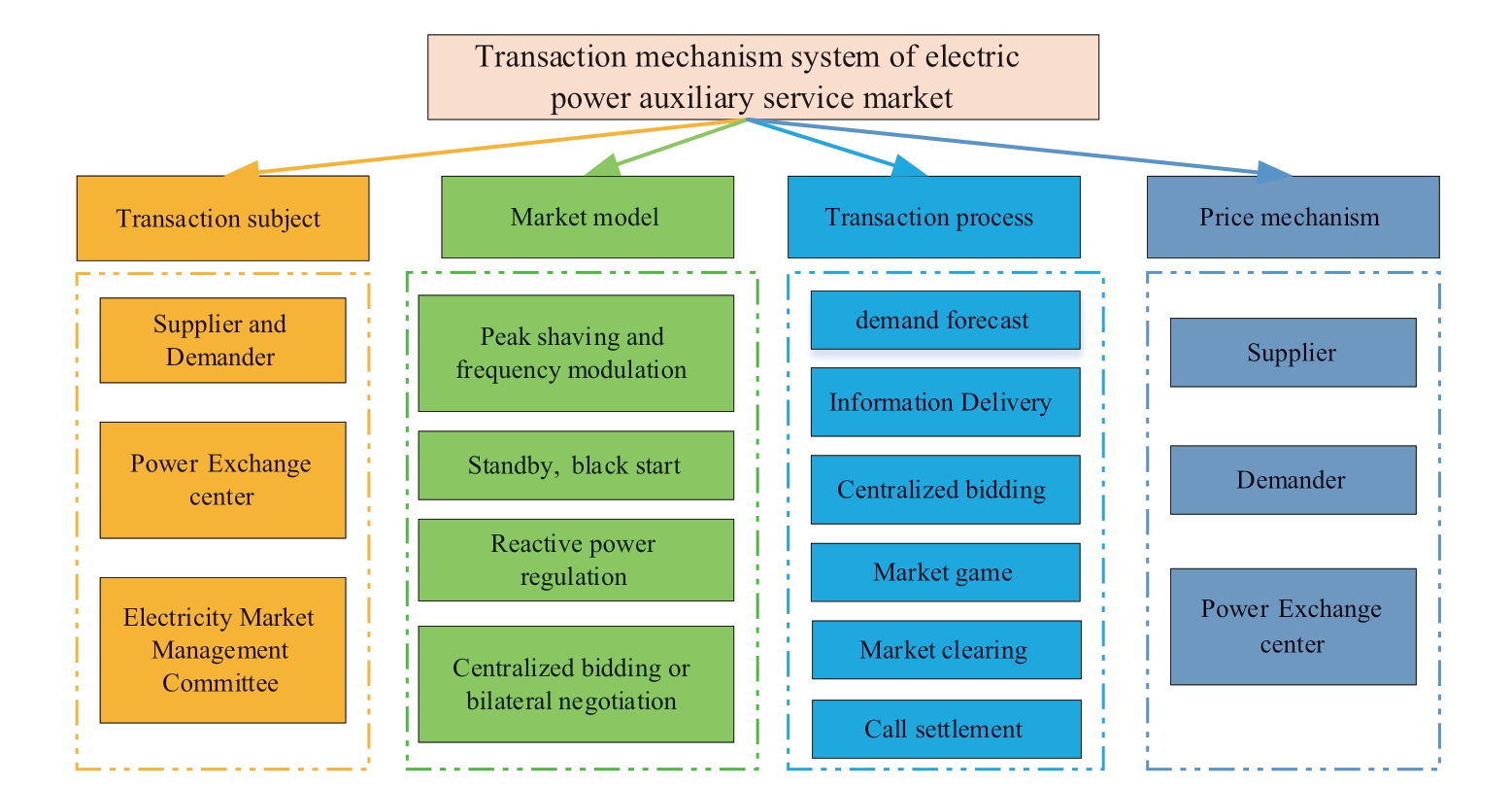
Fig.3 Auxiliary service trading system in the power market
2.2 Development of evaluation metrics for auxiliary service transactions
(1) Auxiliary services transaction varieties
The transaction varieties of auxiliary power services generally include primary FM,AGC,peak-shaving,reactive power regulation,standby,black start,and related concerns[22].The type of electric power auxiliary service transaction was used in the development evaluation index to measure auxiliary service transactions,which is a finalized index.
(2) Transaction scale of regional auxiliary services
The transaction scale of the regional power auxiliary service market was among the key indicators used to measure the development of regional auxiliary service market transactions.The calculation is as follows:

where represents the trading capacity of the participants in the regional power auxiliary service market,and
represents the transaction power of the auxiliary services of the kth variety.
(3) Supply/demand ratio of the interprovincial auxiliary service market [23]
The market supply/demand ratio was one of the indicators of effectiveness used to measure the development of auxiliary service market transactions and was also used to measure the development of the regional power market auxiliary service trading mechanism.The calculation is as follows:

where represents the marketsupply/demand ratio of regional power market auxiliary service transactions,
indicates the total demand of the interprovincial auxiliary service market predicted in a certain period,and
refers to the total supply of the interprovincial auxiliary service market representing actual demand.
(4) Proportion of auxiliary service option-trading power
The proportion of auxiliary service option-trading power refers to its proportion in total regional market-trading power.The specific calculation is as follows:

where represents the annual growth rate of the transactions in the auxiliary service market,
indicates the number of transactions in the auxiliary service market in the current year,and
indicates the number of market participants or market members registered in the previous year.
2.3 Reasonable compliance
(1) Annual growth rate of participating transactions
The annual growth rate of participant transactions in the electric power auxiliary service market refers to the difference between the number of participants in the market in the current year and the number of market participants in the previous year,reflecting increases or decreases in the number of participants in the electric power market.The specific calculation is as follows:

where represents the annual growth rate of the transactions in the auxiliary service market,
indicates the number of transactions in the auxiliary service market in the current year,and
indicates the number of market participants or market members registered in the previous year.
(2) Average zero compensation ratio
In power auxiliary service market transactions,setting an unreasonable compensation calculation threshold or a low level of the grid-connected unit operation management will lead to zero compensation fees for the auxiliary service provider.The average zero compensation ratio refers to the ratio of zero compensation for all auxiliary service varieties.The specific calculation is as follows:
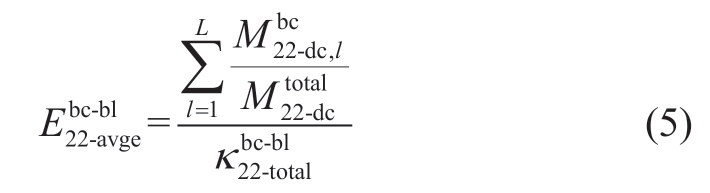
where represents the average zero compensation ratio,indicates the total amount of compensation for the auxiliary services,
indicates the number of compensated auxiliary service providers,and represents the total number of auxiliary service providers.
(3) Average compensation density
To reflect overall compensation in the auxiliary service market,average compensation intensity is calculated as follows:
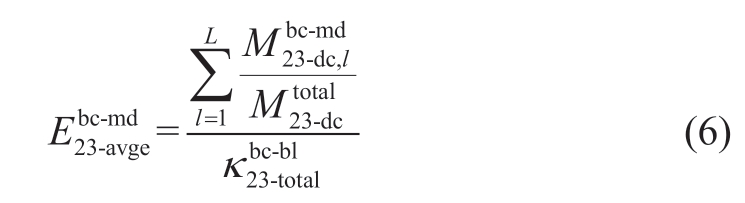
where represents the average compensation density,and
refers to the number of auxiliary service providers within the given scope of the compensation intensity.
(4) High-bid-winning ratio in the regional market
The high-bid-winning rate indicator reflects the match of an auxiliary service provider’s bidding strategy with existing strength.A high-bid-winning rate indicator was used to evaluate auxiliary service providers’ bid-winning rate and market strength as follows:
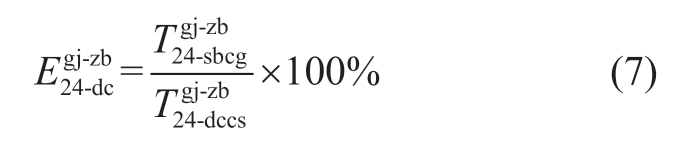
whererepresents the high-bid-winning rate of the regional auxiliary service market transaction,
indicates the number of times that the auxiliary service providers in the regional power market declared at a higher price and successfully bid,and
refers to the bidding times declared by the auxiliary service providers in the regional power market.
(5) Transaction price fluctuation ratio
The fluctuation ratio of the auxiliary service transaction price reflects the degree of fluctuation in the marginal price[24].When there is a higher degree of transaction price fluctuation,the regional auxiliary service market is more likely to be manipulated.The equation for determining the transaction price fluctuation ratio is as follows:

where represents the price fluctuation ratio of auxiliary service transaction prices;
and represents the maximum and minimum marginal prices of a certain type of auxiliary service when it is traded in the market,respectively; and
represents the average power price during the same period.
2.4 Evaluation index for assessing electric power auxiliary service transaction mechanisms
Many factors affect auxiliary service trading mechanism design in regional power markets,and the forms of evaluation index data are complex.In this study,we analyzed the key factors that affect auxiliary services transaction mechanism design in regional power markets from the perspectives of the various basic needs of the auxiliary service market,market entities,market products,and transactions and bidding for auxiliary services to construct a framework for evaluating of auxiliary service transactions in the power market and reasonable compliance.,as shown in Table 2.
Table 2 Evaluation index system of the power auxiliary service transaction mechanism
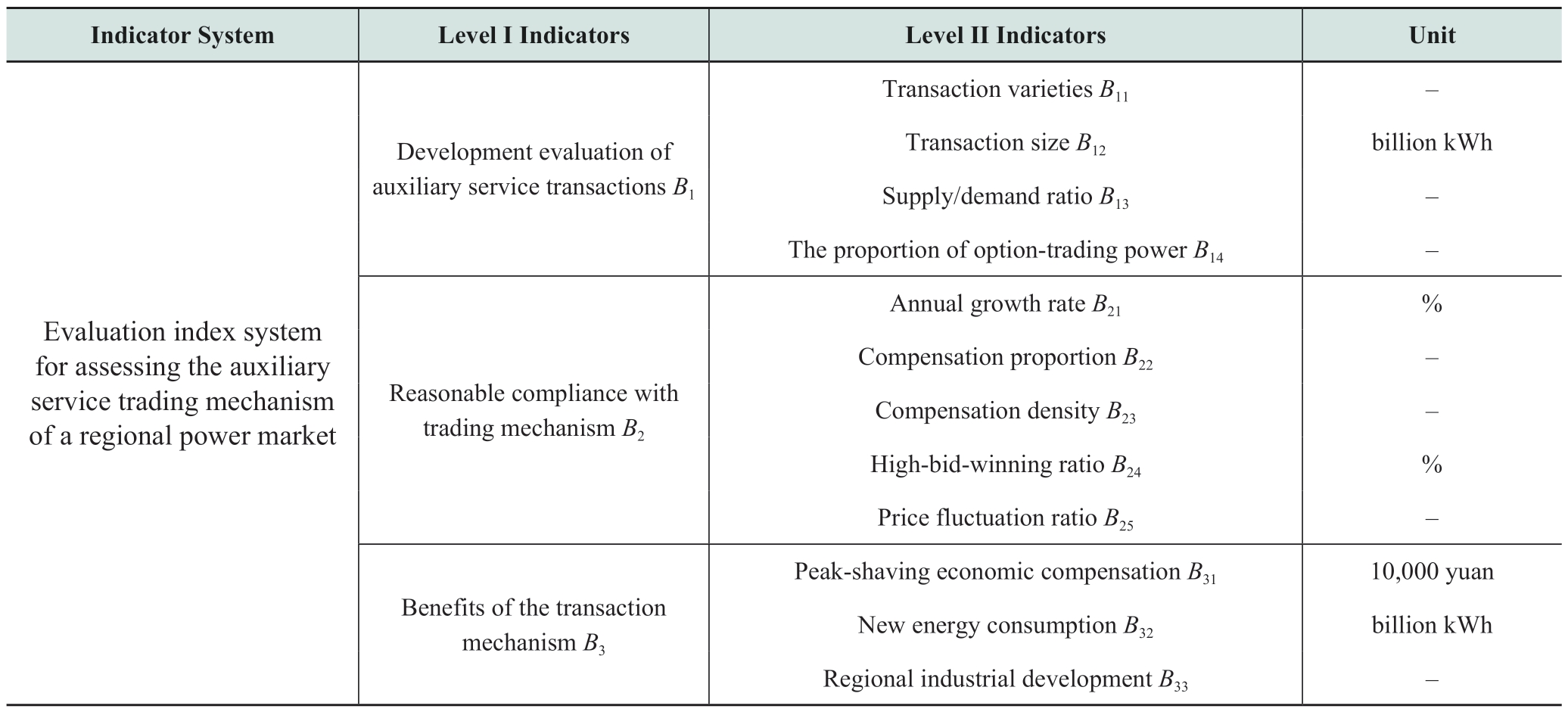
3 Evaluation model for assessing the auxiliary service transaction mechanisms in a regional power market
To analyze the scientificity and rationality of the proposed evaluation index system,the interval value quantitative estimation method and nonlinear dimensionless processing were first used to preprocess the evaluation index data.ANP and RSM were then used as single-weight approaches,and the LSM was used to optimize the weight assignment.Finally,the gray correlation TOPSIS method was used to comprehensively evaluate the auxiliary service trading mechanism of the regional power market.
3.1 Combination weighting optimization model
For the proposed evaluation index,a smaller deviation in the weight value under subjective and objective weighting is better; therefore,the LSM was used to optimize the combination of the evaluation index weights.The specific steps proceeded as follows:
(1) Determine the evaluation function of the combination weighting
The weight vector solved using the ANP method is as follows: ,and the weight vector solved using the RSM method is as follows:
The LSM combination weighting evaluation function is as follows:
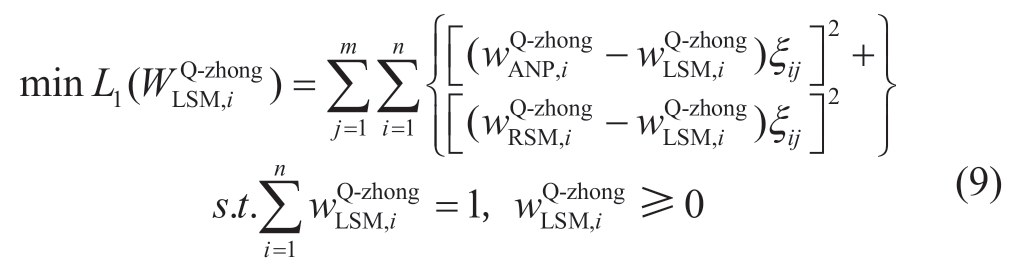
(2) Perform a Lagrange transformation
We then transformed the LSM combined weighting evaluation model using Lagrange transformation,and the changed combined weighting evaluation function is expressed as follows:

The differential solution of the changed LSM combination weighting evaluation function was performed to obtain equations (11) and (12):
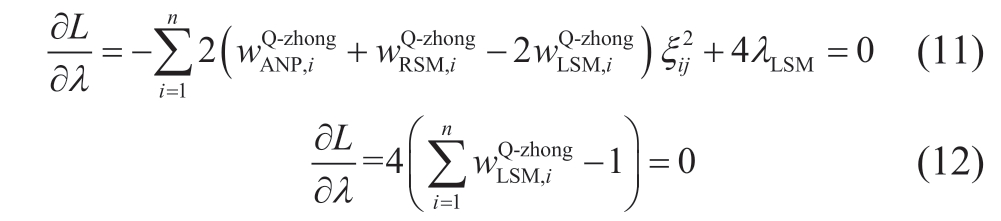
(3) Construct an equivalent matrix equation
The equivalent matrix equation is expressed as The matrix and weight vector in the equivalent matrix can then be expressed as follows:



(4) Solve the equivalent matrix equations
The equivalent matrix equations were solved to obtain the combined weight vector based on the proposed evaluation index,which can be expressed as follows:
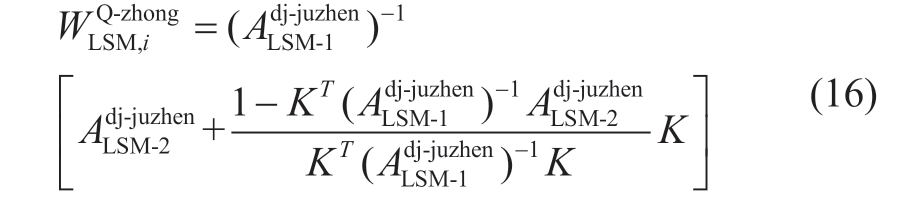
3.2 Evaluation model based on gray relational TOPSIS
TOPSIS ranks evaluation objects by virtue of ideal positive and negative solutions,and the traditional TOPSIS model is improved by using the gray correlation degree to describe the closeness between the evaluation and ideal indicators of each auxiliary service transaction mechanism more accurately,thereby enhancing the rationality and effectiveness of evaluation results.The specific steps are as follows:
(1) Build a multiattribute decision matrix
Suppose there are m regional electric power auxiliary service markets to be evaluated,the evaluation system of the auxiliary service market mechanism includes n indicators,andrepresents the ith attribute value of the jth regional electric power auxiliary service market to be evaluated; then,the multiattribute decision-making matrix
can be expressed as follows:
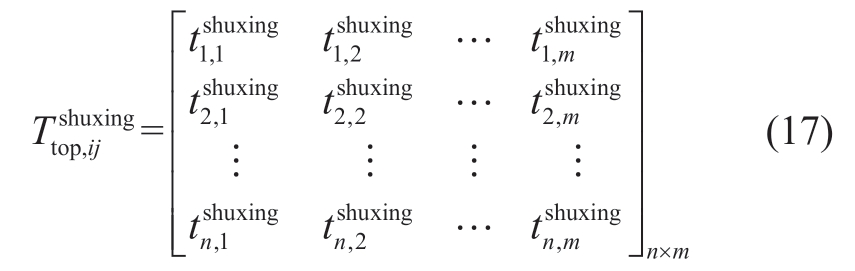
(2) Determine the positive and negative ideal solutions of the attribute index
The positive and negative ideal solutions of the attribute index are as follows:
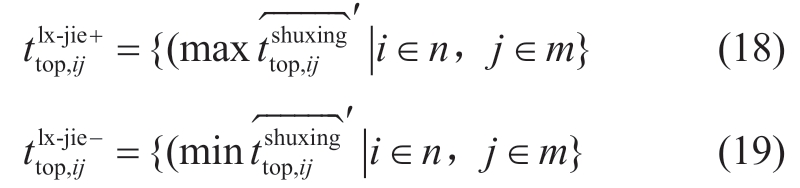
where represents the positive ideal solution of the attribute index,and
r epresents the negative ideal solution of the attribute index.For the inverse index (cost type or small),the equations of the positive ideal solution and negative ideal solution to determine the attribute index are the opposite.
(3) Euclidean space distance for solving positive andnegative ideal solutions
The sum of the Euclidean space distance (and
) from each (regional power auxiliary service market)evaluation object is calculated to determine the positive
and negative
ideal solutions,respectively,as follows:
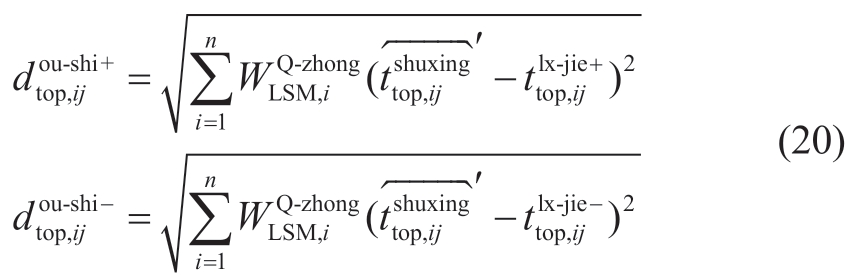
(4) Gray relational degree for solving positive and negative ideal solutions
The degrees of the positive and negative ideal solutions under the gray relational degree can be expressed asand
,respectively,as follows:
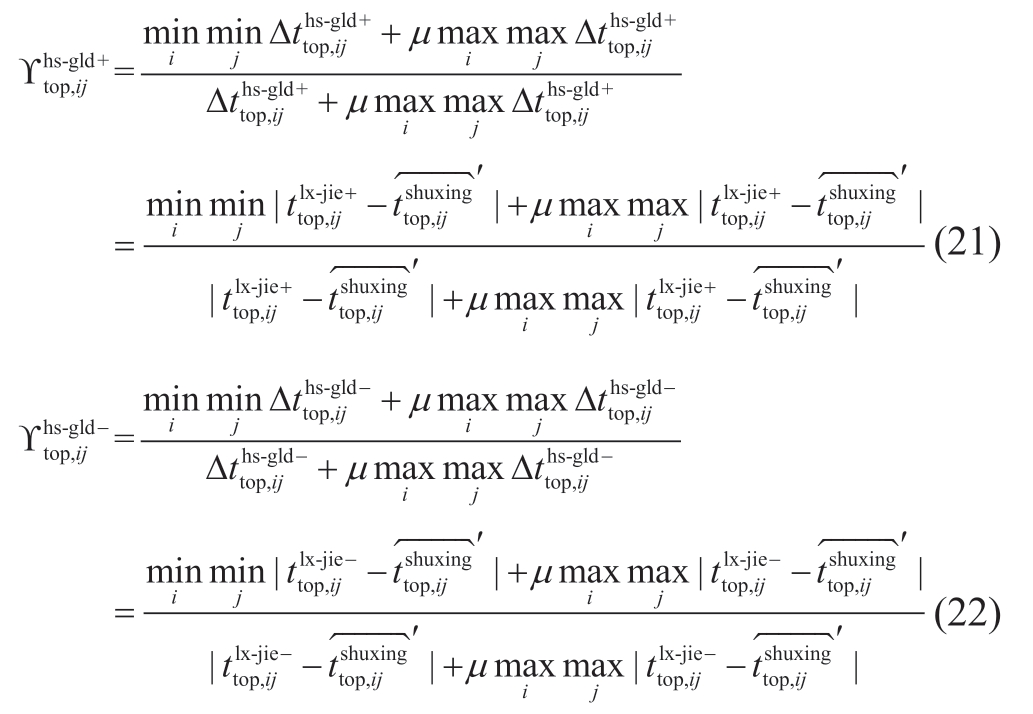
whererepresent the limits of positive and negative ideal solutions,respectively,and μ is usually 0.5.
Based on the above calculation steps,the gray correlation degree between the jth (regional power market)evaluation object and the positive and negative ideal solutionscan be obtained as follows:

(5) Determine the proximity between the evaluation object and positive and negative ideal solutions
The formula for calculating the proximity between the evaluation object and positive and negative ideal solutions is as follows:

where γ1 and γ2 represent the proximity coefficients,and 0.5 is generally taken for both.
(6) Determine relative closeness and rank advantages and disadvantages
The relative closeness of the regional power auxiliary service market can be expressed as,as shown in equation (25),where a larger value indicates a better effect of the regional power market auxiliary service trading mechanism and a worse effect of the trading mechanism:

We illustrate the calculation process used in the proposed evaluation method of the regional power market auxiliary service trading mechanism in Fig.4.
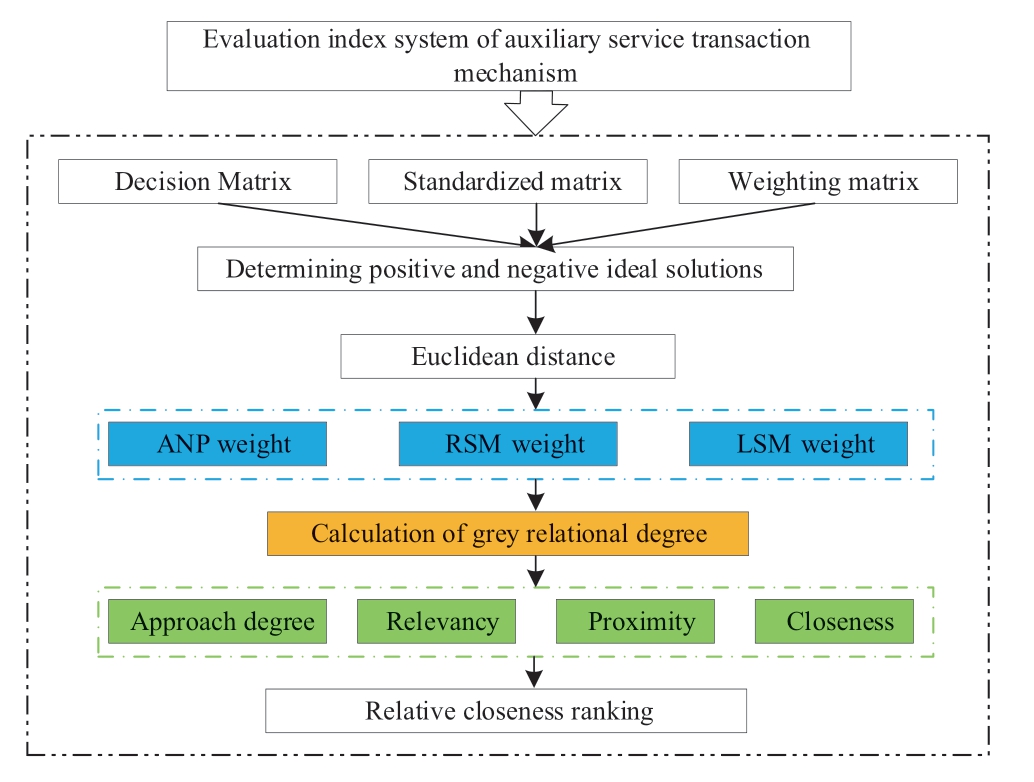
Fig.4 Illustration of the proposed evaluation method of the power auxiliary service transaction mechanism
4 Example analysis
4.1 Basic data
This study analyzed the key factors affecting ancillary service trading mechanism design in the regional power markets and constructed an evaluation index system for assessing auxiliary service trading mechanisms in regional power markets.Based on the basic data of regional power market auxiliary service transactions provided by four regions in China,The purpose is to compare and analyze the operation of the four regional markets and provide evaluation results,as shown in Table 3.
Table 3 Basic data of evaluation indicators for auxiliary service transaction mechanism
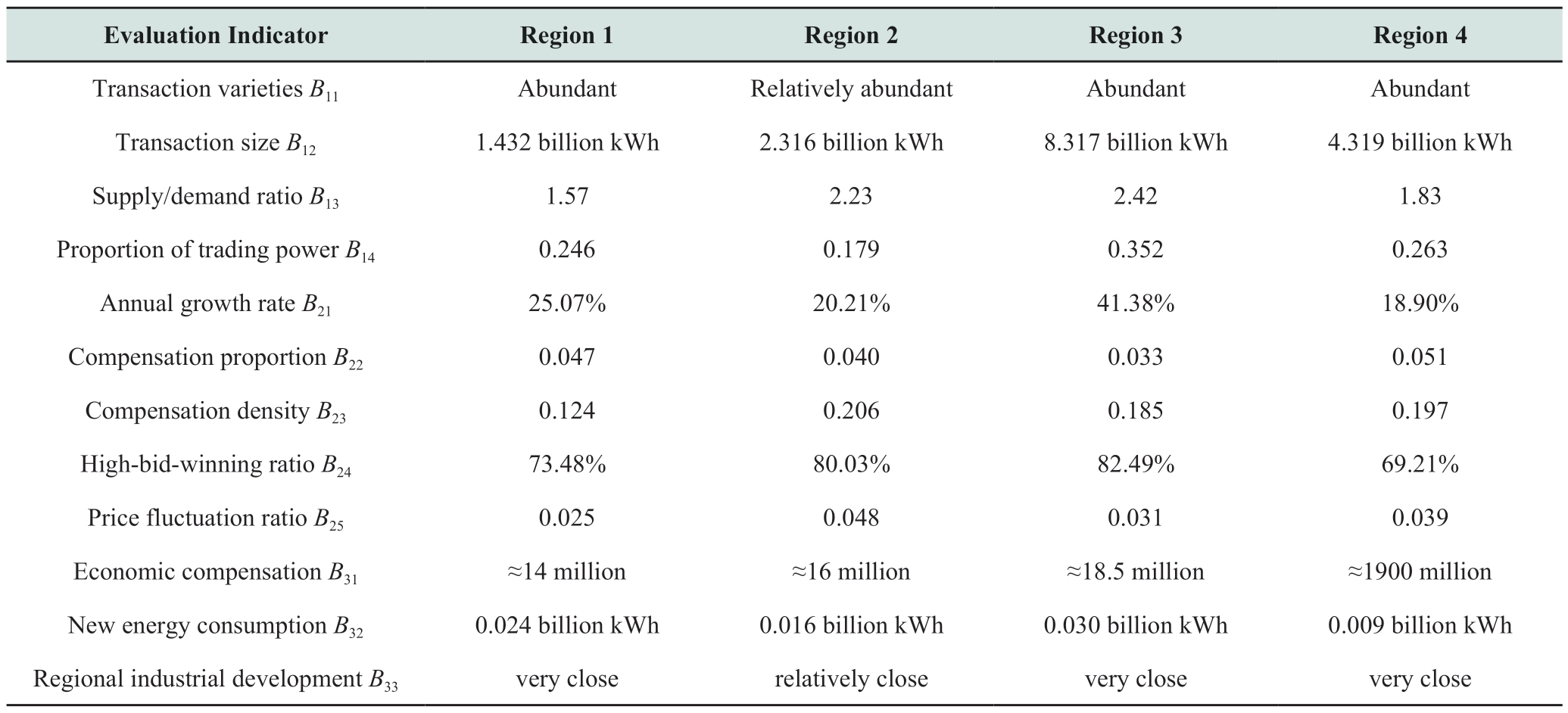
4.2 Pretreatment of evaluation indicators
To verify the effectiveness and applicability of the proposed interval value quantitative estimation and nonlinear dimensionless processing models,basic data on regional power market auxiliary service transactions based on previous research were obtained,and interval value quantitative estimation and nonlinear dimensionless processing methods were applied for data preprocessing.The results are presented in Table 4.
Table 4 Preprocessing results of evaluation indicators for assessing the auxiliary service transaction mechanism
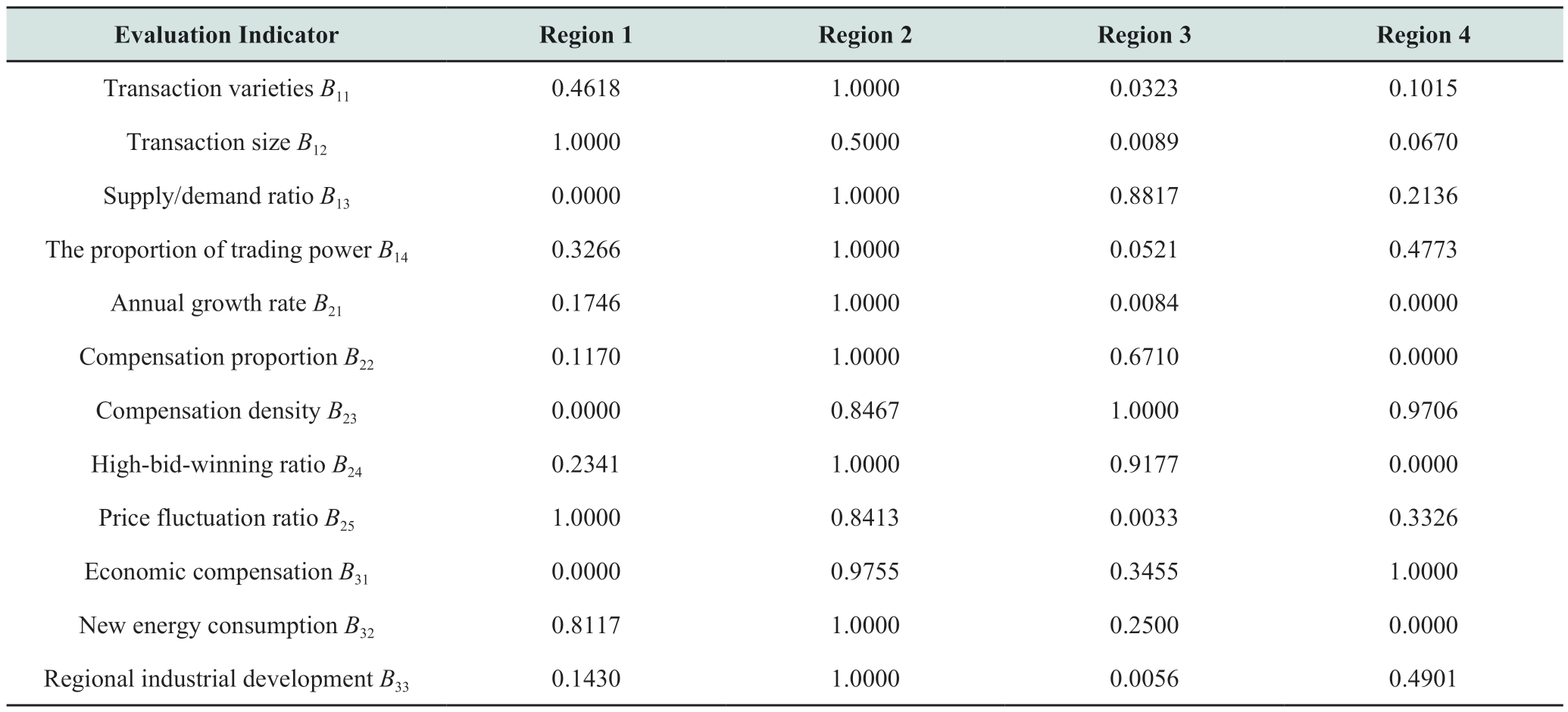
4.3 Optimization of combination weighting
(1) Subjective weighting: the ANP method weight value solution
The basic principle of the ANP method is that the weight of each criterion in the control layer can be obtained by applying the AHP method.The weight of each element in the network layer was determined using the limit hypermatrix.
1) Construction of the weighted hypermatrix
The attribute set of the control layer of the proposed evaluation system was the auxiliary service trading mechanism of the regional power market.The attribute indicators were set as follows:
:Development evaluation of auxiliary service transactions;
:Reasonable compliance with trading mechanism;
:Benefits and welfare of the transaction mechanism.
The network layer has three attribute sets.Theattribute indicators were set as follows:
:Transaction varieties of auxiliary services;
:Transaction scale of regional auxiliary services;
:Supply/demand ratio of the interprovincial auxiliary service market;
:Proportion of auxiliary service option-trading power.
Dominance was determined according to the influence of each attribute index.A judgment matrix was constructed with a scale of 1–9 points,and the ranking vectorof the attribute index was obtained using the following eigenvalue method:

The ranking vectors of all the attribute indicators were used to construct a matrix,and the hypermatrixunder the control layer can be expressed as follows:
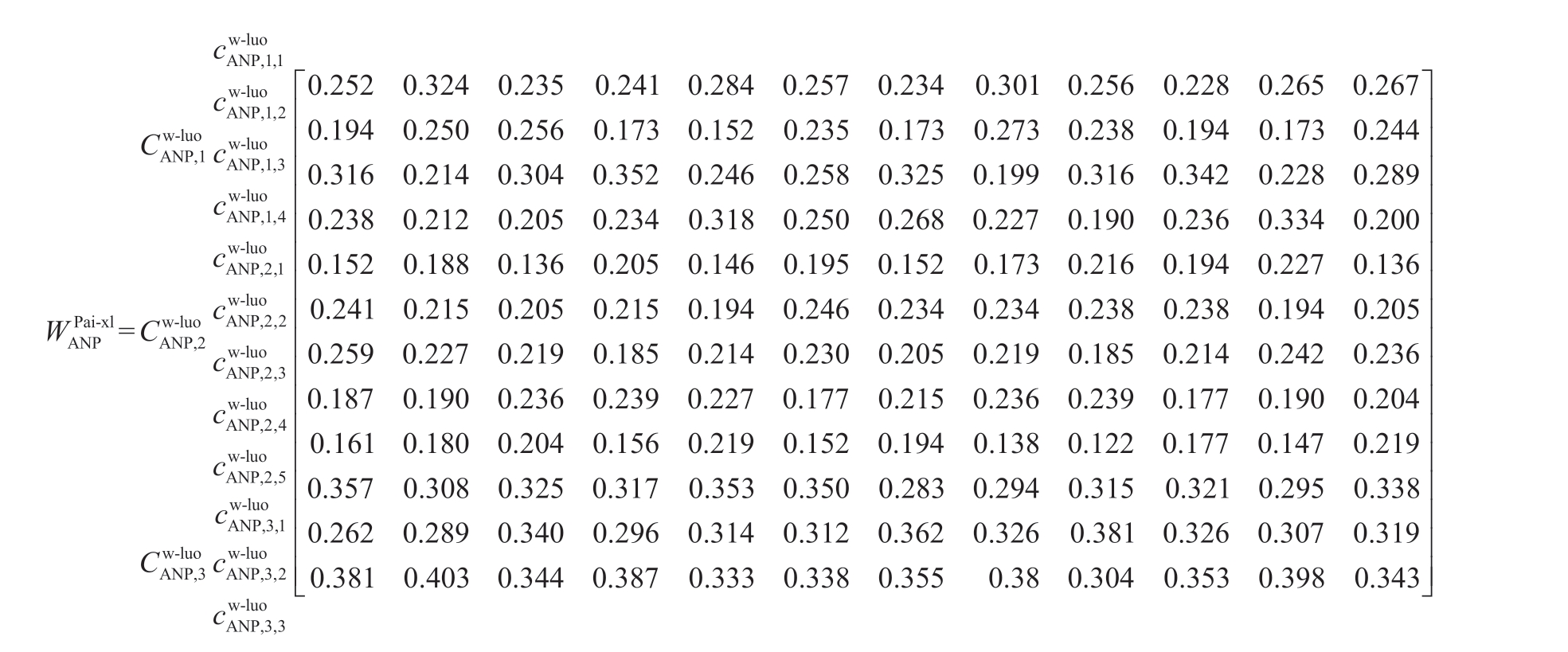
Each attribute of the hypermatrix is also a matrix,and the sum of the elements in each matrix is 1.To simplify the calculation,the hypermatrix can be normalized to obtain a weighted hypermatrix.
2) Relative ordering of limit hypermatrices
The weight values of the attributes of the constructed weighted hypermatrixreflect the first-step dominance of attribute i over attribute j.The relative ranking vector for the limit was obtained by solving the limit of the weighted hypermatrix to obtain the final weight value
,as shown in Table 5.
Table 5 Evaluation index weight value calculation results
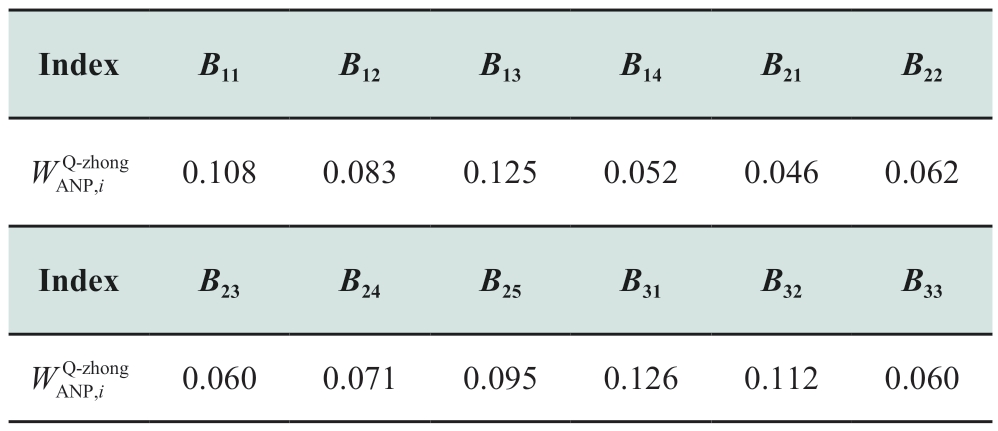
(2) Objective Weighting RSM
The RSM does not require subjective evaluations of knowledge or data.Instead,based on analysis and reasoning of knowledge or data,it searches for knowledge hidden in the system itself to determine the weight value of the evaluation indicators as follows:
1) Determine the interval of discrete attributes
Because the RSM can only handle discrete attribute values based on attribute sets,and some of the index data in the proposed evaluation system are continuous,it was necessary to discretize the continuous data.The data were discretized according to the attribute interval size,and the results are shown in Table 6.
Table 6 Discretization results of rough-set data
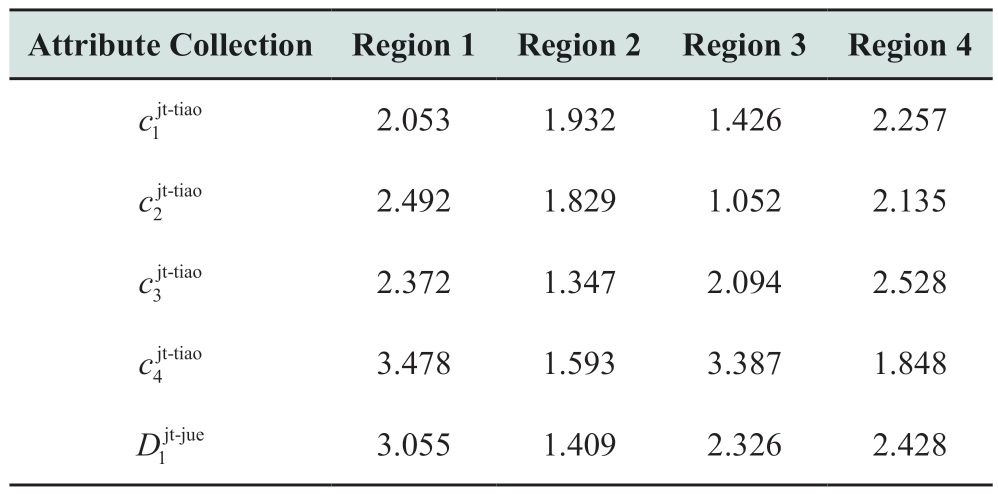
2) Determine the equivalent sets of rough sets
Table 4 shows that the equivalent sets are as follows:
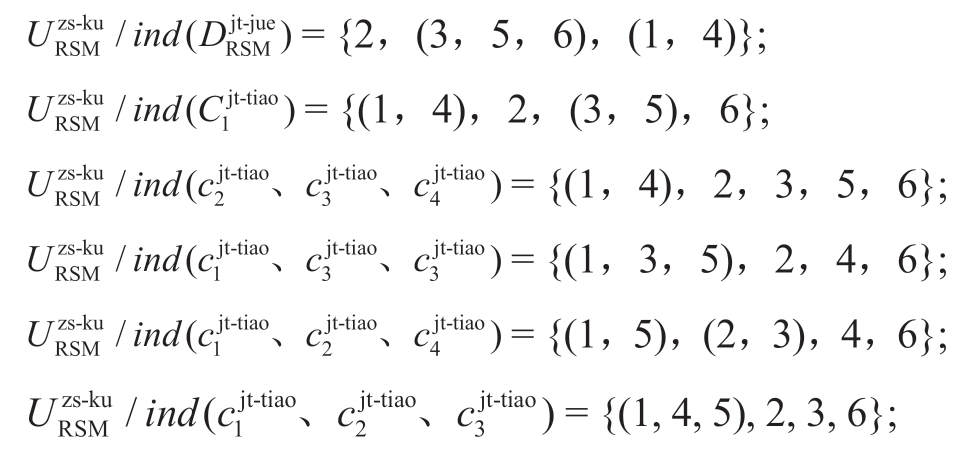
3) Calculate the significance of the condition attributesto the decision attributes
The formula was used to calculate the significance of condition attributes to the decision attributes.The results are as follows:
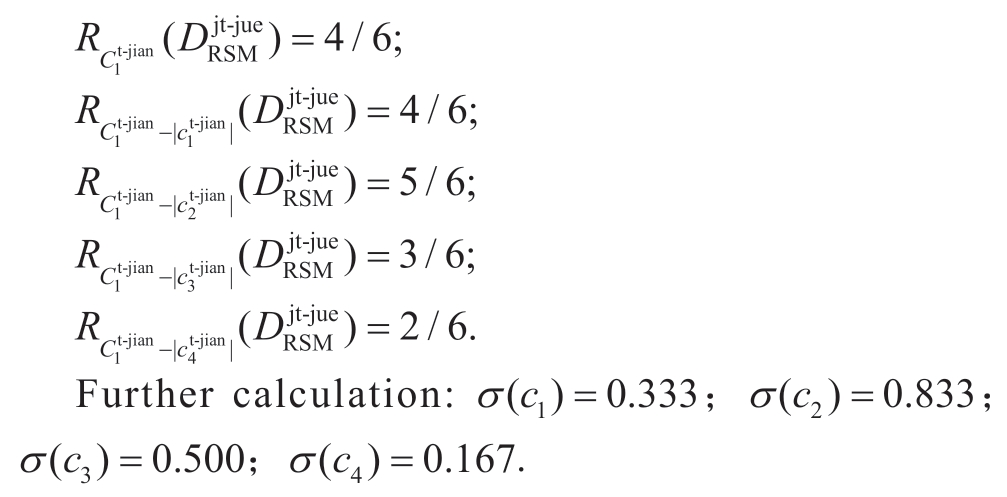
Similarly,the decision attributes can be determined according to the importance of the conditional attributes ofrelative to
relative to
.
4) Determine the weight values of the evaluation index
The index attribute following the index reduction toobtain the index weight value is shown in Table 7.
Table 7 Evaluation index weight value calculation results
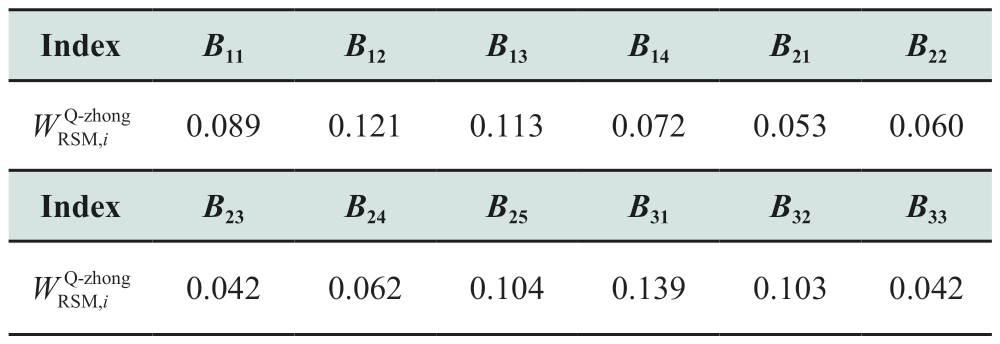
(3) Combination weighting LSM weight value solution
The LSM reduces the uncertainty of the combination coefficient in addition to ensuring full use of the information.The standardized matrix of the four (regional power market) evaluation objects for the 12 indicators in the proposed evaluation system is Z=(Zij)m×n.The evaluation value of the ith evaluation object can be expressed as follows:

The Lagrange function transformation was performed for the constructed LSM combination weighted optimization evaluation model and the following changes were made:

The combination weighting optimization was obtained as follows:
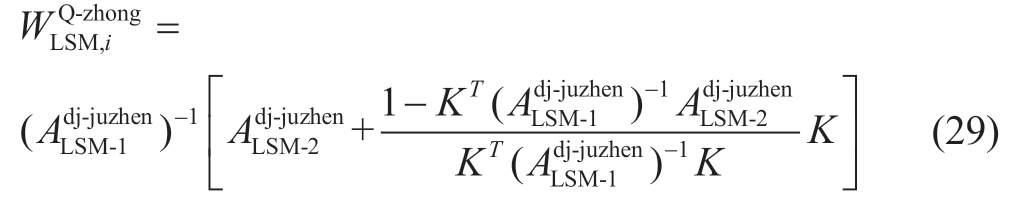
The weight value after the combination weighting optimization is,as shown in Table 8.
Table 8 Evaluation index system of the power auxiliary service transaction mechanism
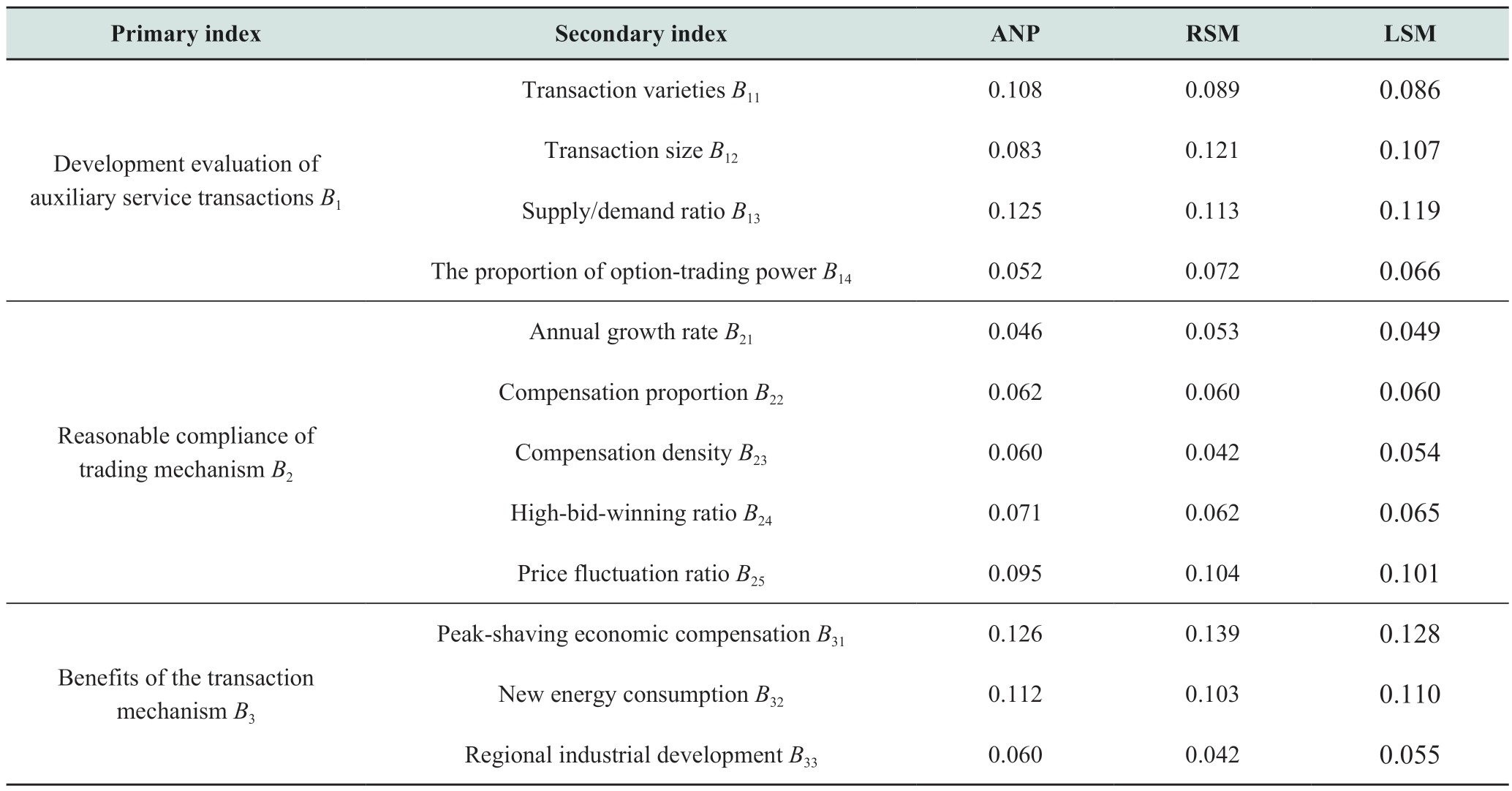
4.4 Evaluation model calculation results
(1) Build the multiattribute decision matrix
As previously described,the example shows four regional power markets (Regions 1–4).The proposed evaluation system includes four secondary indicators and 12 tertiary indicators.The attribute value of the first evaluation indicator representing Region 1 in the table was recorded,and the multiattribute decision-making matrixwas then constructed as follows:
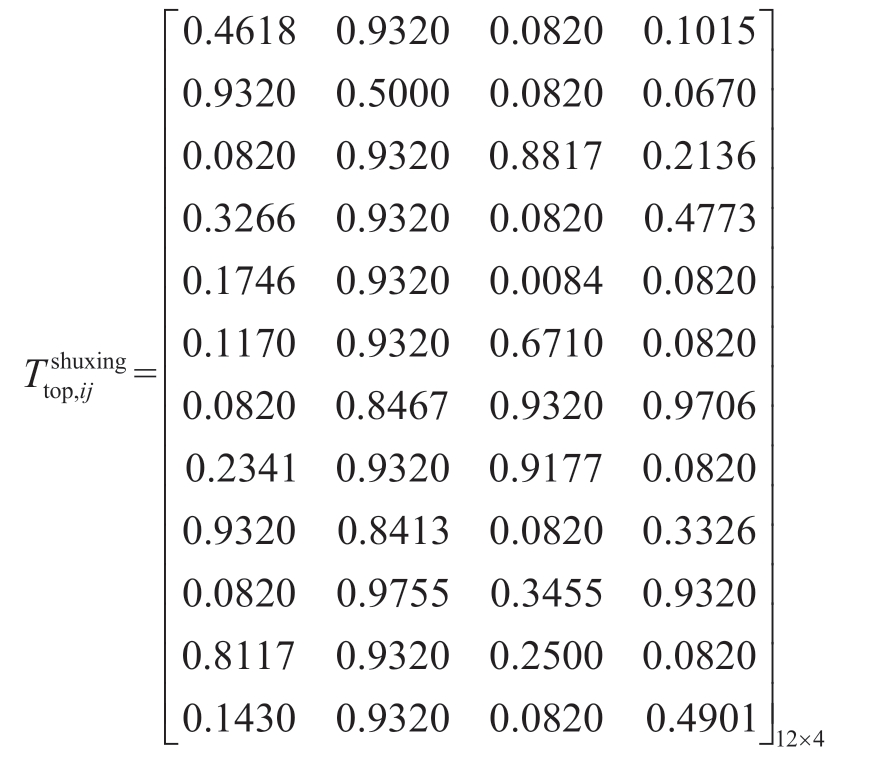
(2) Construct the weighted matrix
The weighted matrix was obtained by multiplying the calculated combination weight and each standardized attribute index in the standardized matrixas follows:
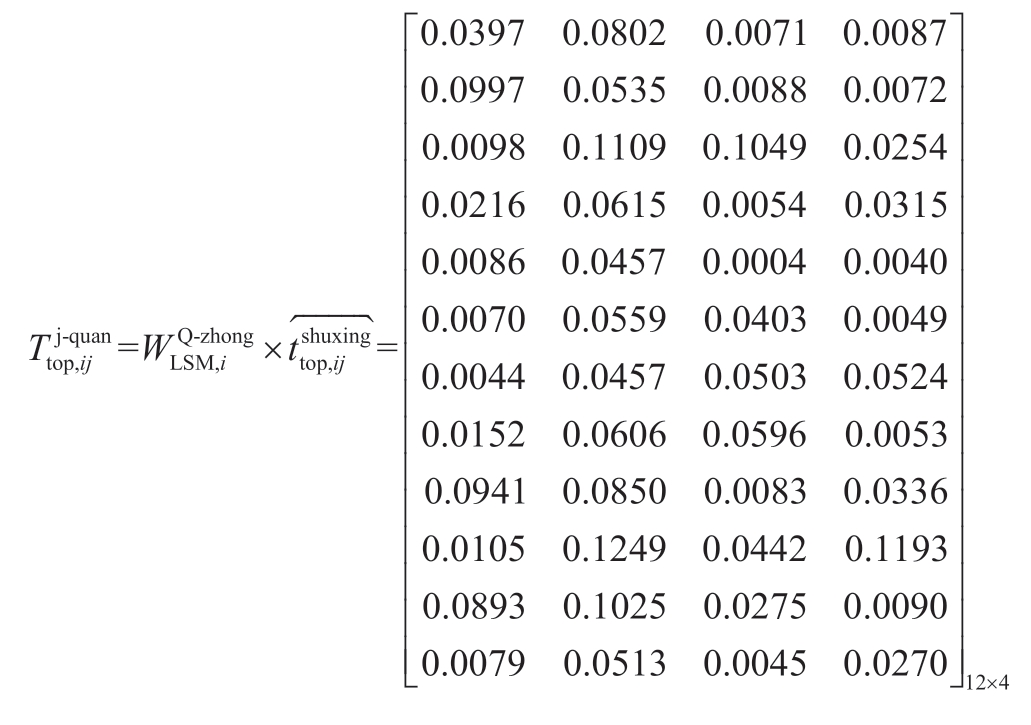
(3) Determine the sum of the positive and negative ideal solutions of the attribute indices
The calculation example was analyzed Taking Region 1 as an example.The positive ideal solutiondetermined based on the weighted matrix is 0.1249 and the negative ideal solution
is 0.0004.
(4) Determine the Euclidean space distance sum for positive and negative ideal solutions
The Euclidean space distances between the evaluation object and the positiveand negative
ideal solutions in Region 1 were calculated to be
and
,respectively.
(5) Determine the sum of the gray relational degree for solving the positive and negative ideal solutions
Based on the calculation,the gray correlation degrees between the evaluation object and the positive and negative ideal solutions areand
,in Region 1,respectively.
(6) Solve the proximity between the evaluation object and the positive and negative ideal solutions
The Region 1 proximities between the evaluation object and the positive and negative ideal solutions areand
,respectively.
(7) Solve the relative closeness
The relative closenessof Region 1 is
.The larger the base value,the better the effect of the regional power market auxiliary service trading mechanism,and the worse the effect of the trading mechanism.
According to the above steps,we obtained a summary of the evaluation results of Regions 2–4,as shown in Table 9.
Table 9 Summary of the evaluation results assessing the auxiliary power service transaction mechanism
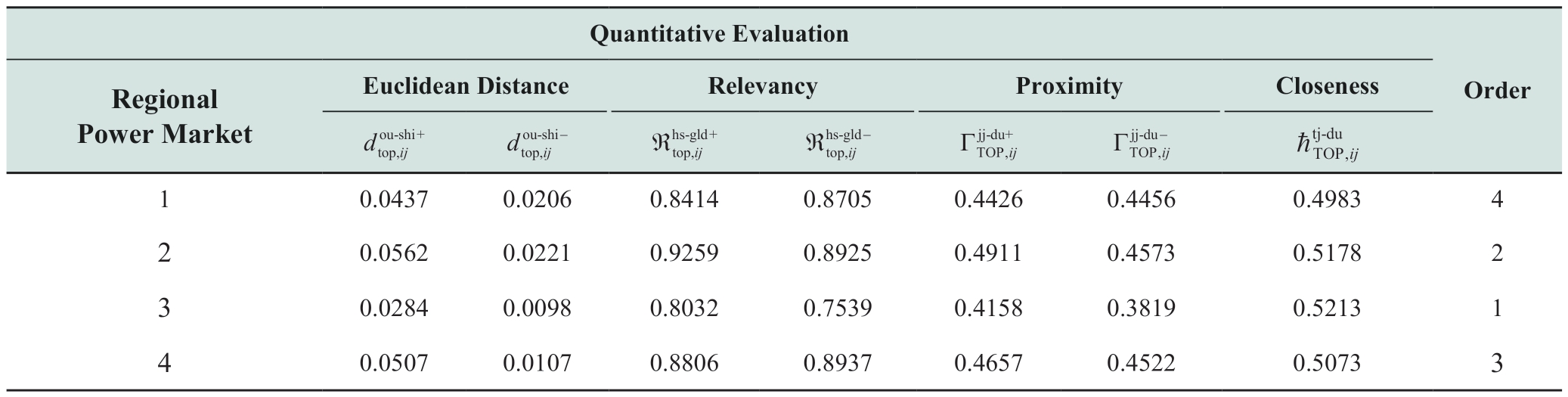
The evaluation results are ranked as follows: Region 3 >Region 2 > Region 4 > Region 1; therefore,the auxiliary service trading mechanism of Region 3 was rated as the best.
5 Conclusions and policy recommendations
Based on theoretical research regarding the evaluation of the power trading mechanism,we proposed an evaluation model for assessing the regional power market auxiliary service trading mechanism based on an ANP–RSM–LSM combined weighting gray correlation TOPSIS method.
(1) The scope of auxiliary services covers the whole process from the power production end to the power user end.Aiming at the problems of wide market scope and multiple subjects,this study deeply analyzed the key factors affecting auxiliary service trading mechanism design in regional power markets and constructed a framework for evaluating auxiliary service trading and trading compliance.The proposed evaluation index examines the benefits and welfare of the trading mechanism.
(2) Continuous data,non-quantitative data,and other types of data are included in the proposed evaluation system.Considering the rationality of subjective and objective indicators and objective evaluation requirements,a comprehensive evaluation model of regional power market auxiliary service trading mechanism was established,including a combined ANP–RSM–LSM weighted optimization model.The gray correlation TOPSIS method was used for comprehensive evaluation.Regional power markets of four provinces in China were selected for empirical analysis to elicit suggestions for future market construction.
(3) Because the rules,products,and mechanisms of the auxiliary service market differ in different regions,and the influencing factors involved are also complex,limited to the current circumstances of the construction of the power market,this study did not propose an auxiliary service market mechanism that is applicable to the national unified power market.In future research,we will continue to explore the trading mechanism of the power market and expand our contributions to the construction of a unified power market.
(4) The rationale for building a unified auxiliary service market is to stimulate the motivation of market participants,improve unit performance,and optimize resource allocation.In the early stage of the market,there are still considerable human factors interfering with resource allocation.To realize congenial connections within a unified market and regional markets,it is essential to clarify the relationship between regional markets and the unified market.Furthermore,the guidance of market incentives should be prioritized,considering the reasonable and fair income of all participants in the design of the market mechanism to fully leverage the role of the market.
Acknowledgements
This research was supported by the Beijing Power Exchange Center (Study on the Medium and Long Term Time Division Transaction Mode and Balance Mechanism of Electric Power) and supported by the National Natural Science Foundation of China(No.72171082).
Declaration of Competing Interest
We declare that we have no conflict of interest.
References
[1] Li L X,Cao X L (2022) Comprehensive effectiveness assessment of energy storage incentive mechanisms for PV-ESS projects based on compound real options.Energy,239: 121902
[2] Yang S X,Wang X F,Ning W Q,et al.(2021) An optimization model for charging and discharging battery-exchange buses:Consider carbon emission quota and peak-shaving auxiliary service market.Sustainable Cities and Society,68: 102780
[3] Dey B,Raj S,Mahapatra S,et al.(2022) Optimal scheduling of distributed energy resources in microgrid systems based on electricity market pricing strategies by a novel hybrid optimization technique.International Journal of Electrical Power& Energy Systems,134: 107419
[4] Jiang Y C,Hou J X,Lin Z Z,et al.(2019) Optimal bidding strategy for a power producer under monthly pre-listing balancing mechanism in actual sequential energy dual-market in China.IEEE Access,7: 70986-70998
[5] Afshar K,Ghiasvand F S,Bigdeli N (2018) Optimal bidding strategy of wind power producers in pay-as-bid power markets.Renewable Energy,127: 575-586
[6] Shang J C,Zhang Z F,Han G (2005) Study on transaction mechanism and model of regional layered power market.Automation of Electric Power Systems,29(4): 6-10
[7] Albadi E,Saadany I (2008) A summary of demand response in power markets.Electric Power Systems Research,78: 1989-1996
[8] Jiang D B,Zhang Y,Jin Z X,et al.(2006) The concept of giving consideration to market efficiency and economic fairness in the unified power market.Power Grid Technology,06: 59-62 (in Chinese)
[9] Xue C,Ren J,Ma X W,et al.(2021) Market mechanism and practice of peak shaving auxiliary services in the northwest for high proportion of new energy consumption.China Power,54(11): 19-28 (in Chinese)
[10] Liu J P,Shen W,Hu G,et al.(2018) Research on the Design of China’s Unified Power Market Direct Trading Platform.Communication Power Technology,35 (01): 134-136 (in Chinese)
[11] Li C B,Kang C Q,Xia Q,et al.(2004) Study on trading mechanism of regional power market.Power System Technology,28(7): 34-39
[12] Brunekreeft G (2015) Network unbundling and flawed coordination: Experience from the electricity sector.Utilities Policy,34: 11-18
[13] Jafarzadeh S,Fadali M S,Livani H (2016) Stability analysis of electricity markets using TSK fuzzy modeling.IEEE Transactions on Power Systems,31(2): 1161-1169
[14] Rajabi Mashhadi H,Rahimiyan M (2011) Measurement of power supplier’s market power using a proposed fuzzy estimator.IEEE Transactions on Power Systems,26(4): 1836-1844
[15] Zhao W M,Zhou B R,Li X L,et al.(2019) Comprehensive evaluation index system and evaluation method of power market.China Southern Power Grid Technology,13 (08): 74-80 (in Chinese)
[16] Shi J H (2020) Research on evaluation indexes and methods of Guangdong power market.Guangzhou: South China University of Technology(in Chinese)
[17] Galán J E,Pollitt M G (2014) Inefficiency persistence and heterogeneity in Colombian electricity utilities.Energy Economics,46(1403):31-44
[18] Whitaker R (2007) Validation examples of the analytic hierarchy process and analytic network process.Mathematical and Computer Modelling,46(7-8): 840-859
[19] Chen D G,Yang Y Y (2014) Attribute reduction for heterogeneous data based on the combination of classical and fuzzy rough set models.IEEE Transactions on Fuzzy Systems,22(5): 1325-1334
[20] Chavent G (1979) Identification of distributed parameter systems:About the output least square method,its implementation,and identifiability.IFAC Proceedings Volumes,12(8): 85-97
[21] Wang B Q,Dong J M,Guan Q F,et al.(2020) Study on the risk assessment system of pumped storage power plants based on the grey correlation TOPSIS method.Southern Energy Construction,7 (S2): 56-61 (in Chinese)
[22] Yang X L,Niu D X,Sun L J,et al.(2021) Participation of electric vehicles in auxiliary service market to promote renewable energy power consumption: Case study on deep peak load regulation of auxiliary thermal power by electric vehicles.Energy Science &Engineering,9(9): 1465-1476
[23] Zeng M,Liu H,Cao Y W,et al.(2020) Research on the business model and development strategy of energy storage power station based on the perspective of policy environment-also analyzing the policy effects of industrial development,price compensation and market transaction support.Price Theory and Practice,09:127-131 (in Chinese)
[24] Xu F,Ge Z Q,Wu X,et al.(2019) Market mechanism and clearing model of interprovincial peak regulation ancillary service for regional power grid.Automation of Electric Power Systems,43 (16): 109-1115
Full-length article
Received: 10 December 2022/ Accepted: 15 February 2023/ Published:25 April 2023
Genzhu Li
lgz6869532@163.com
Le Liu
119992104@126.com
Xueyu Chen
ccchenxy@jl.sgcc.com.cn
Dexin Li
1163600676@qq.com
Chang Liu
1975988911@qq.com
Dunnan Liu
liudunnan@163.com
2096-5117/© 2023 Global Energy Interconnection Development and Cooperation Organization.Production and hosting by Elsevier B.V.on behalf of KeAi Communications Co.,Ltd.This is an open access article under the CC BY-NC-ND license (http: //creativecommons.org/licenses/by-nc-nd/4.0/).
Biographies
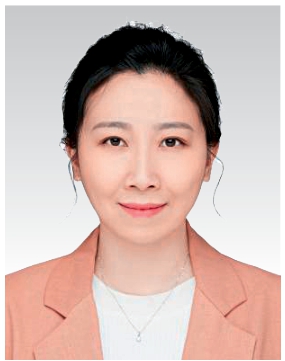
Le Liu received the Ph.D.degree at Dalian University of Technology,Dalian.She is working in Jilin Power Exchange Center Co.,Ltd.,Changchun.Her research interests include electricity market,electricity trading,energy internet,etc.
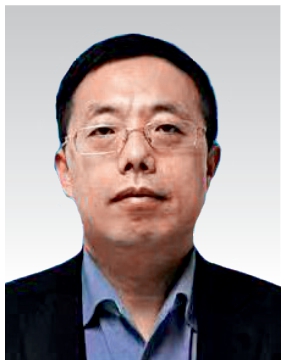
Xueyu Chen received the master degree in Northeast Electric Power University,Jilin.He works in Jilin Power Exchange Center Co.,Ltd.,Changchun.His research interests include electricity market,clean energy system,etc.
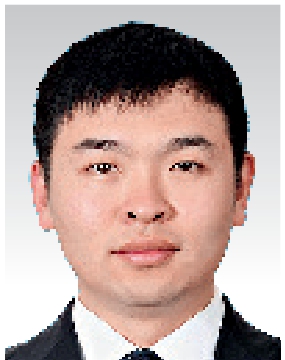
Dexin Li received his master degree at North China Electric Power University.He is working in Jilin Electric Power Research Institute Co.Ltd.,Changchun.His research interests include electricity market,electricity trading,energy internet,etc.
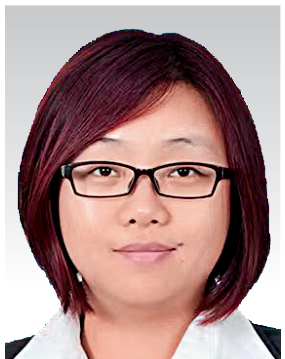
Chang Liu received the master degree at Yokohama National University,Yokohama.She is working in Jilin Electric Power Research Institute Co.Ltd.,Changchun.Her research interests include electricity trading,low-carbon economy,etc.
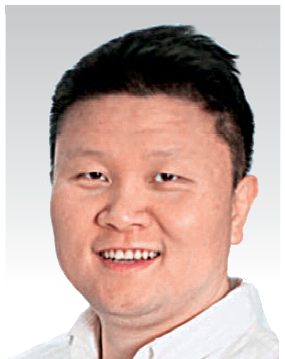
Dunnan Liu is a professor with the School of Economics and Management,North China Electric Power University,Beijing.His research interests include electricity market,renewable energy resource integration,etc.
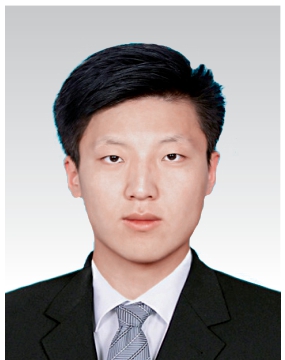
Genzhu Li received his master degree in Industrial Engineering from North China Electric Power University in 2021,and he is pursuing his Ph.D.degree in business administration at North China Electric Power University.His research interests include electricity market and energy internet.
(Editor Dawei Wang)