0 Introduction
With continuous development of natural gas and electric power systems,their connection is becoming increasingly close [1,2],manifested by gradual deep coupling of the power distribution system (PDS) and gas distribution system (GDS) on the user side.Construction of a fully coordinated power-gas integrated energy system (PGIES)improves energy consumption efficiency on the user side and increases the reliability of the overall energy supply through multi-energy complementarity [3].
In an integrated energy system (IES) composed of multiple subsystems,energy coupling causes any fault,energy supply blockage,or shutdown in one subsystem to affect the energy flow distribution optimization of other subsystems [4].In recent years,large-scale energy outages caused by natural disasters,improper operation,and malicious attacks have occurred.In August 2017,six gasfired generators in Taiwan,China were disconnected from the grid owing to misoperation,leading to a significant blackout that ultimately affected approximately 60% of user electricity consumption [5].In February 2021,extremely cold weather in Texas produced significant ice blockage in natural gas pipelines,which led to gas-supply interruption and no electricity generation from gas-fired generators,leading to a system-wide blackout [6].The advantages of multi-energy complementation must be used to restore the global energy supply as soon as possible when the energy supply is interrupted.
With the large-scale grid connection of gas power generation,gas supply blockages in natural gas systems greatly affect safe and reliable power supply [7].When the gas supply in a GDS is limited,distribution of the gas source to the gas load,and whether to feed the gasfired generator to produce electricity in the PDS should be determined dynamically according to actual conditions.Similarly,changes in gas demand owing to power supply blockage in the PDS require readjustment of the gas flow in the GDS.Thus,the supply restoration of the PGIES after an energy shutdown requires coordinated decisionmaking.In reference [8],the authors considers the interdependence between loads,provides power,water and natural gas to key users in the required proportion,and refines the distribution of sources.It was found that the available power from the power storage system should be used first for load restoration; if there is still a need for power load restoration,natural gas from the gas storage system should be converted into electric energy to increase the power supply reliability of the electric power system in reference [9].At present,most energy supply restoration decisions of the PGIES ignore the difference in the time constants of electric energy flow and gas energy flow and do not consider the delay characteristics [10,11] or transient characteristics of the gas system [12].Using a fixed optimization step size [13],the restoration decision of the PGIES is simplified,which reduces the guidance required for the restoration operation of an actual IES.Thus,it is necessary to consider the transmission speed difference between power energy and natural gas,and on this basis,develop a strategy for energy supply restoration to make full use of the limited sources in the system.
As a complement to fixed power and gas sources,mobile emergency power sources (MEPSs) and mobile emergency gas sources (MEGSs) can be flexibly connected to the system,and can supply energy quickly after energy outage and ensure reliable supply of the system.Most studies have focused on the power-supply restoration decision-making of MEPSs [14-20]; few studies have considered both MEPSs and MEGSs in IES restoration decision-making.In reference[14],a robust optimization restoration model for an isolated distribution network with wind power,PV,and MEPS was established,considering the output uncertainty factors of wind power and PV generators in the PDS.Reference [15]outlined the restoration optimization objective following the demand for system outage restoration and developed a multi-objective restoration scheme optimization model considering the auxiliary function of the MEPS without considering the influence of the MEPS transportation path on the restoration scheme.Reference [16] realized flexible scheduling of MEPS through a two-stage framework,and enhances system restoration.References [17,18] suggested that in blackouts caused by extreme disasters,electric vehicles can be connected to the distribution network through charging piles and be used as energy storage devices to quickly restore important loads.In reference[19],a real-time dispatching strategy was proposed to transport natural gas,which enhances the reliability of the system.In reference [20],MEPSs and MEGSs were used to transport electricity and natural gas for preventive control,which alleviated the supply pressure on power lines and gas pipelines.The dispatching decisions for mobile emergency resources can improve the toughness of a power-gas IES.Considering the dispatching decisions for the MEPS and MEGS,energy restoration of the power-gas IES requires further study.
Through gas-fired generators (GFGs) and power-togas (P2G) devices,the PGIES realizes a bidirectional flow of energy between the PDS and GDS.With the high investment and operation costs of P2G equipment,the gasto-power integrated energy system (G2PIES) is commonly used [21].In this study,a power-supply restoration decision-making method for a mobile emergency source,including a G2P integrated energy system (MG2PIES),was investigated.The composition of the MG2PIES was studied; the coupling characteristics of the PDS and GDS were analyzed,and the impact of the transient process caused by the restoration operation of GDS on the PDS was analyzed.On this basis,PDS,GDS,and mobile emergency source models were established,and an optimization model of MG2PIES energy-supply restoration was established considering the restoration income and cost.According to the model,a coordinated restoration optimization decision method was proposed,including system status recognition,dispatching optimization of both the MEPS and MEGS,G2P gas-flow optimization,and parallel intra-partition restoration scheme optimization for both the PDS and GDS.A numerical example is presented to verify the effectiveness of the proposed coordinated energy-supply restoration optimization method for the MG2PIES.
1 Composition and restoration of MG2PIES
1.1 System composition
The system composition and basic structure of the MG2PIES are shown in Fig.1.The electric power of the PDS source nodes comes from power injection of adjacent power systems at the same or higher level of voltage,and the fixed or mobile power sources within the PDS.MEPSs include vehicle-mounted mobile power storage systems and mobile emergency generators [22].Similarly,the gas supply of the GDS source nodes comes from gas injection of adjacent gas systems at the same or higher level of pressure,and the fixed or mobile gas sources within the GDS.The GDS has gas wells and tanks with fixed spatial positions,and vehicle-mounted mobile gas storage systems with adjustable spatial positions.A GDS is usually equipped with a pressure regulator to maintain constant pressure of the gas source node; its flow can be adjusted according to the nominal capacity [23].
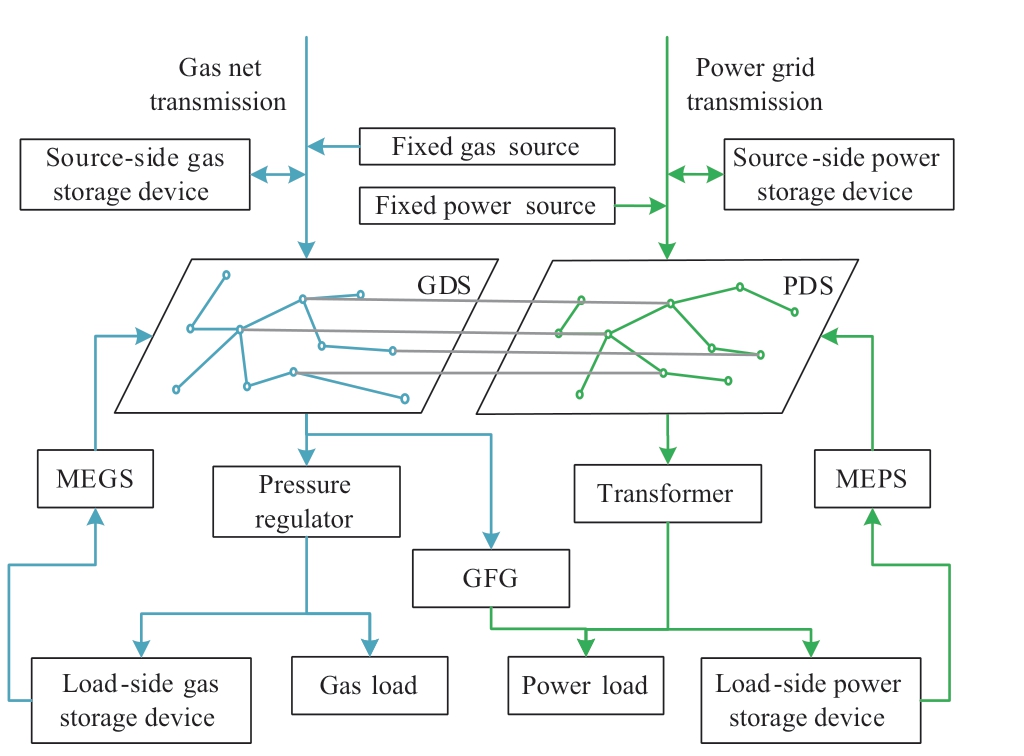
Fig.1 Basic structure of MG2PIES
Considering the grid connection conditions,the MEPS should be connected to the grid at the MEPS connection points reserved in the grid; multiple MEPSs can be connected to the same connection point in parallel.The MEGS connection point in the GDS can only access one MEGS at a time; if natural gas is exhausted,another MEGS can be used to provide a continuous gas supply [24].MEPS and MEGS usually have fixed storage locations and are transported to the corresponding connection points for energy supply through the transportation network.The transportation speed can theoretically reach 100 km/h; the actual speed depends on the real-time road and traffic conditions.
1.2 Energy coupling and coordinated restoration of MG2PIES
As an entire energy system,the MG2PIES should create a global distribution scheme of energy supplies and restoration sources according to the need for the load to be restored in the restoration process after energy supply interruption.The allocation and coordination of the two energy forms of power and gas in the power grid,gas network,and transportation network are relatively complicated.Considering that the PDS and GDS have relatively independent data communication and dispatching control systems,decoupling iteration and coordination optimization are currently feasible restoration decisionmaking methods [25,26].If the operation time is ignored,the transmission speed of power energy in the PDS is much faster than the transport speed of the MEPS in the traffic network.The priority of using a fixed power source to restore power supply can accelerate the speed of restoration and obtain greater restoration benefits; thus,in this study,MEPSs can only supply loads that cannot obtain power from fixed electric power sources.However,the transmission speed of natural gas is slow owing to the influences of pipeline pressure,inner diameter,temperature,and other factors [27,28].The transmission speed of natural gas in a GDS is close to the transport speed of an MEGS in a traffic network on a time scale.This study considers the real-time conditions of the traffic network to make a unified optimization decision for the gas supply of a fixed gas source in the GDS and MEGS.The restoration decision of the MG2PIES should fully consider the coupling characteristics at the source,network,and load sides,coordinate distribution of available energy of fixed and mobile power and gas sources to the loads to be restored,and optimize the capacity and path of energy transmission in the power,gas,and transportation networks.Considering the time scale difference between different energy systems,the restoration decision of the MG2PIES is preliminarily decoupled as follows: (1) the dispatching control of the PDS should consider the transient process of the GDS;(2) the dispatching decision of the MEPS should consider the distribution of available power of fixed power sources in the PDS within the expected transportation time interval;(3) the scheduling control in the GDS should only consider the influence of the steady-state results of the PDS,ignoring the transient process of the PDS; (4) the restoration optimization scheduling in the GDS must consider both fixed gas sources and MEGSs.
2 Optimization model of energy system restoration
2.1 Objective function
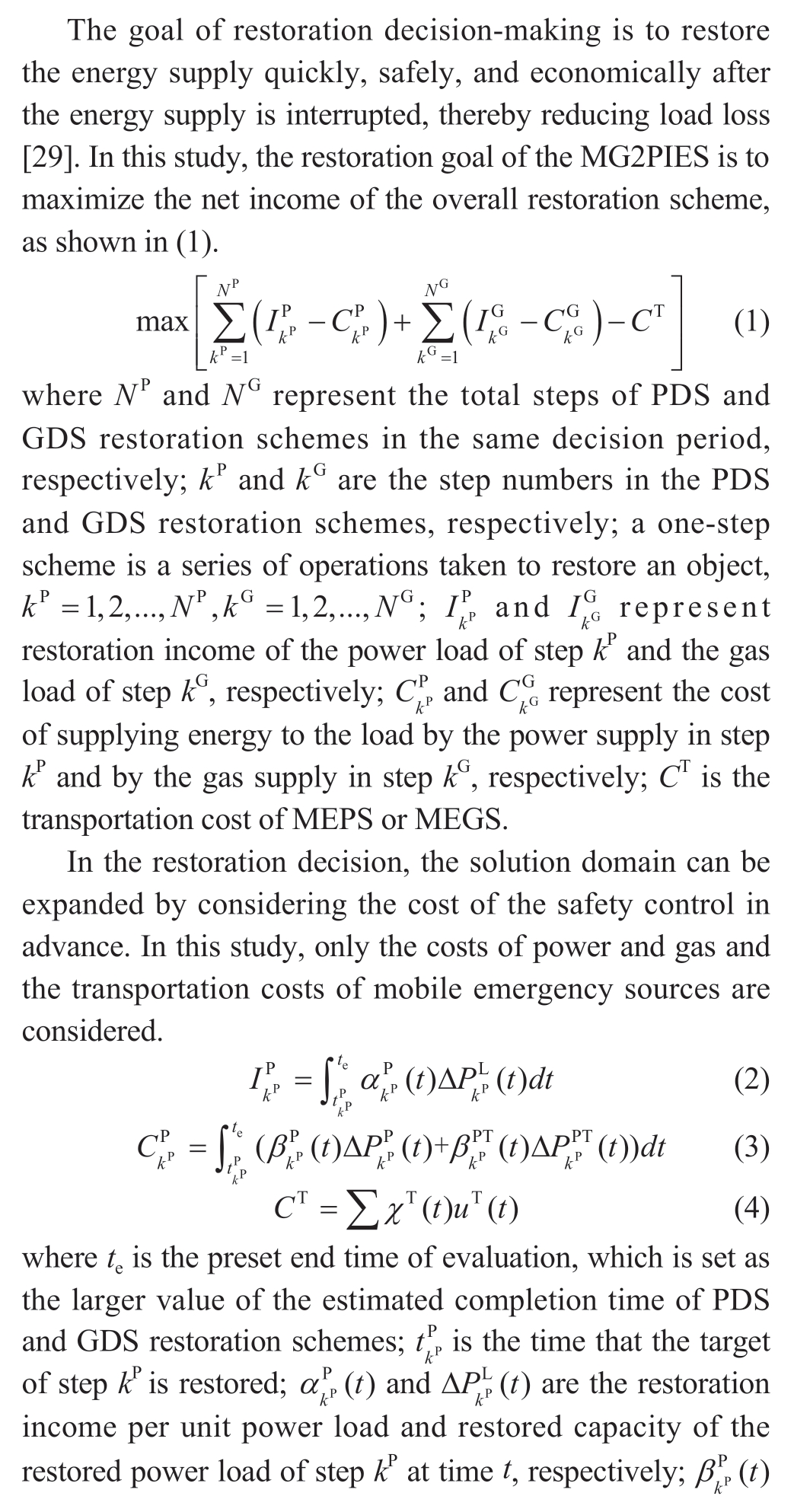
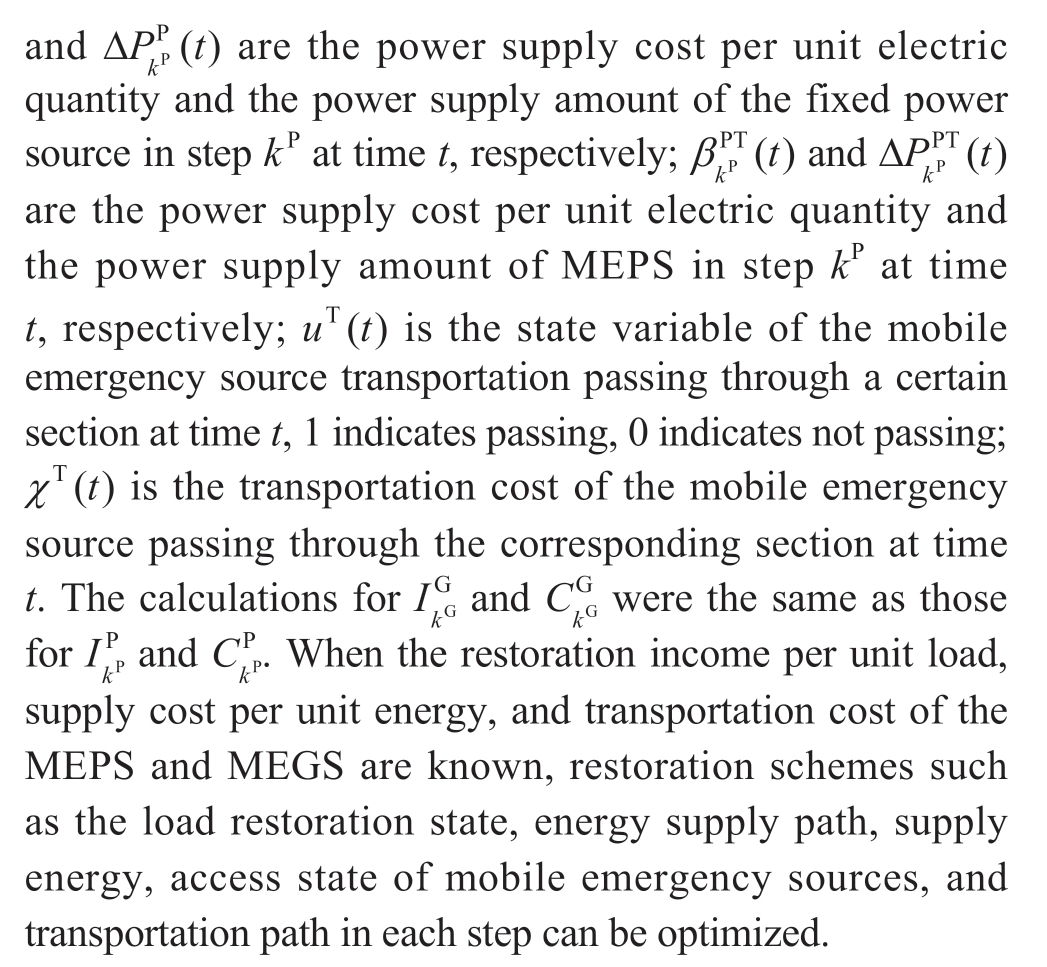
2.2 Constraints
2.2.1 PDS constraints
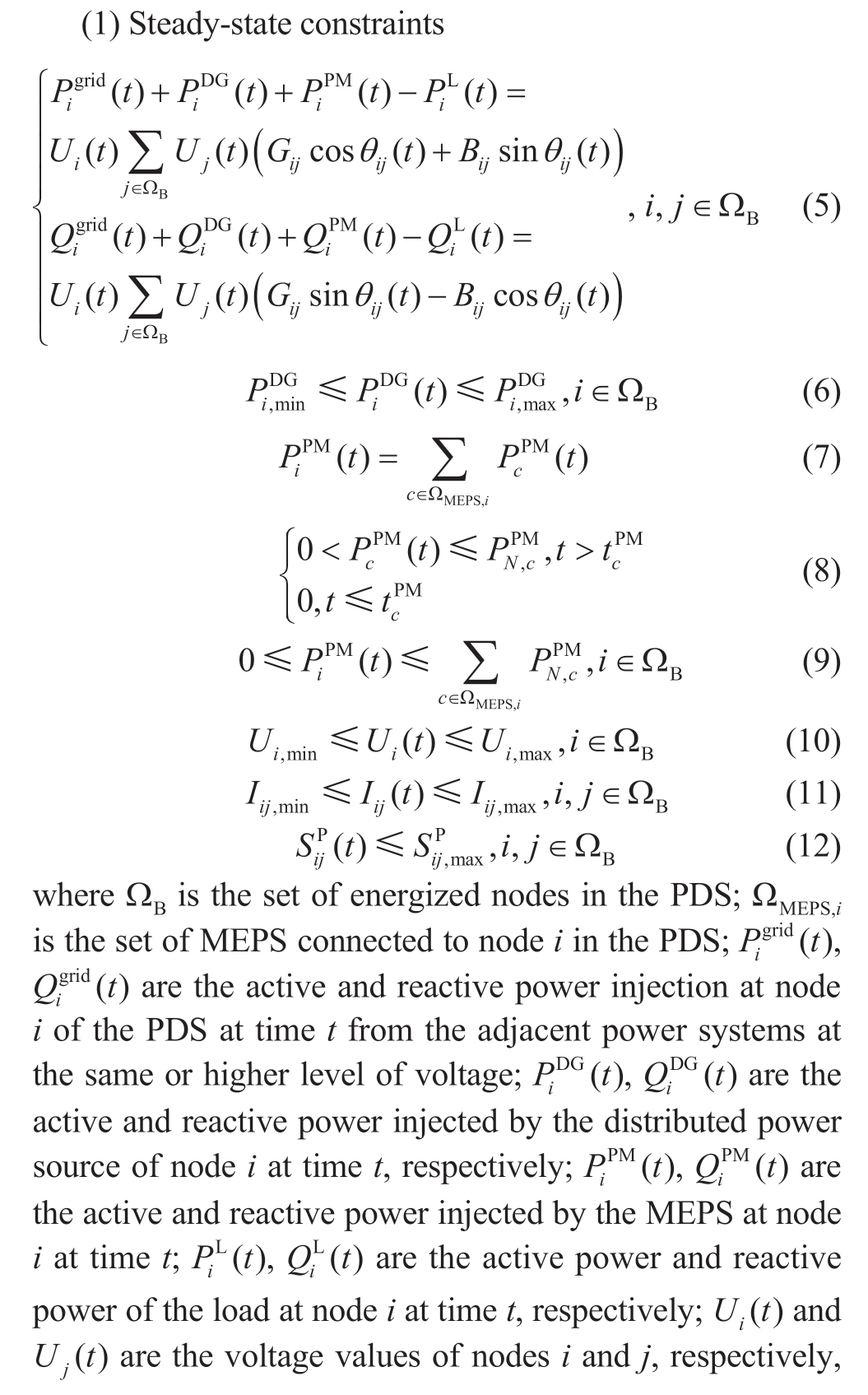
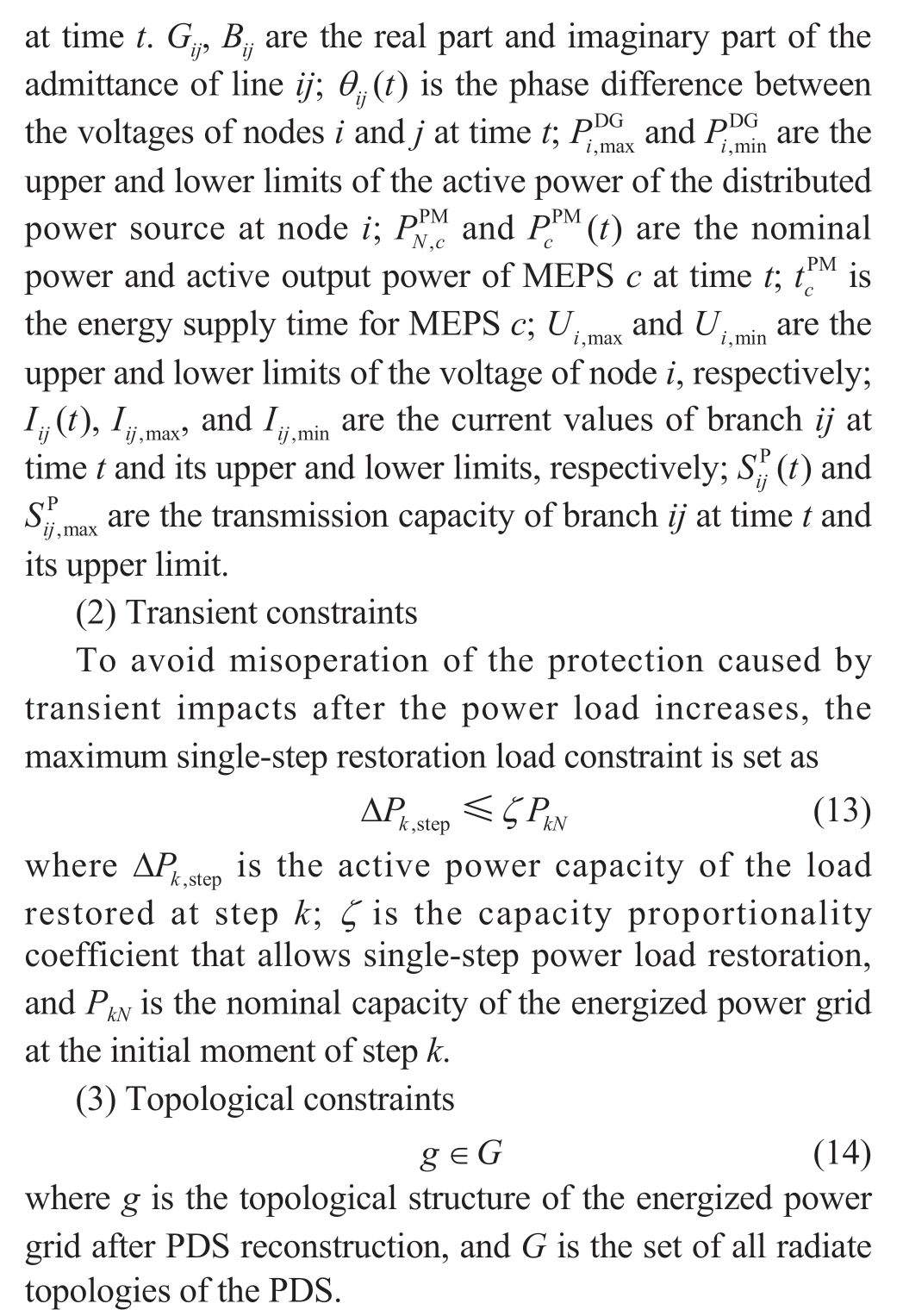
2.2.2 GDS constraints
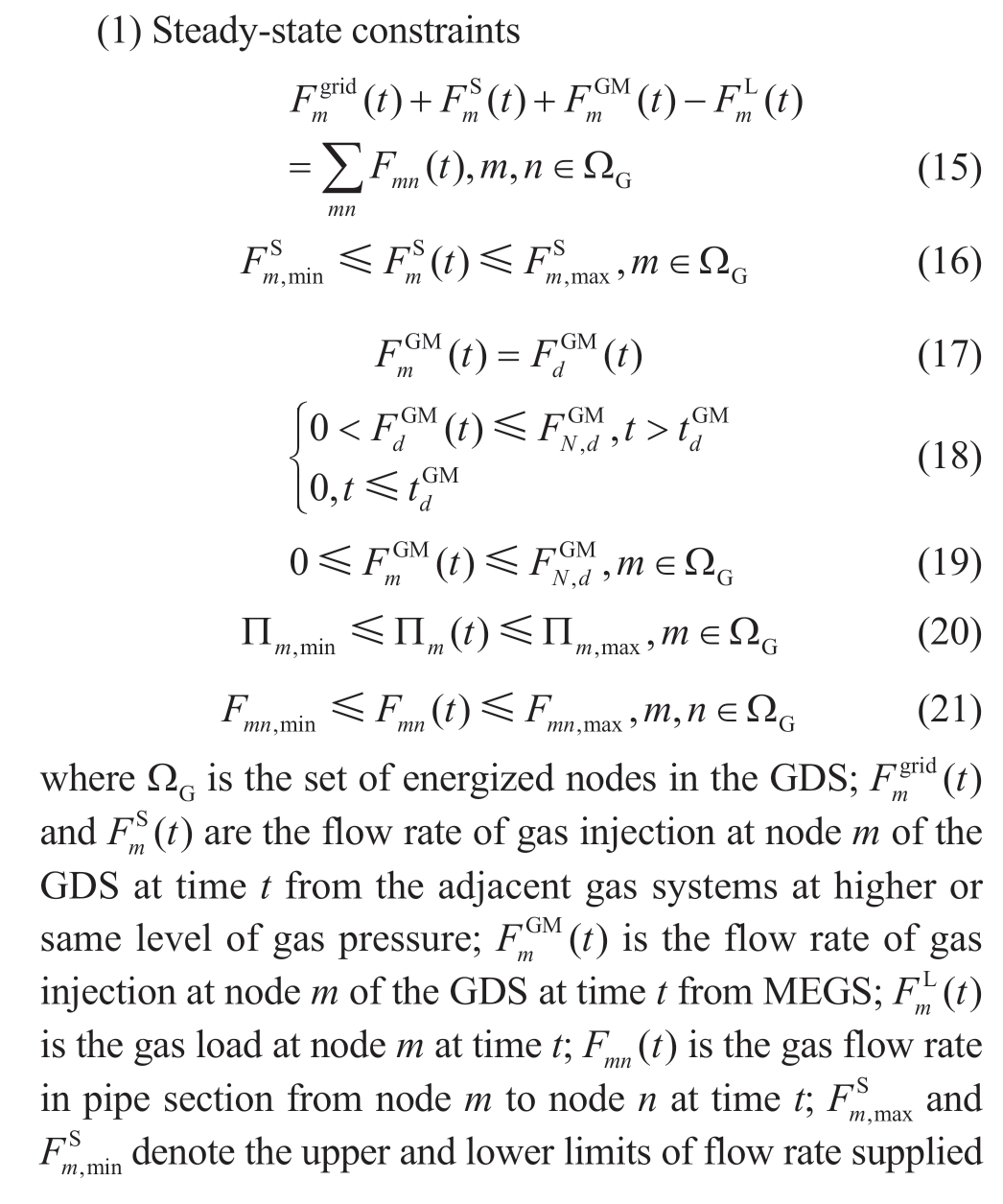
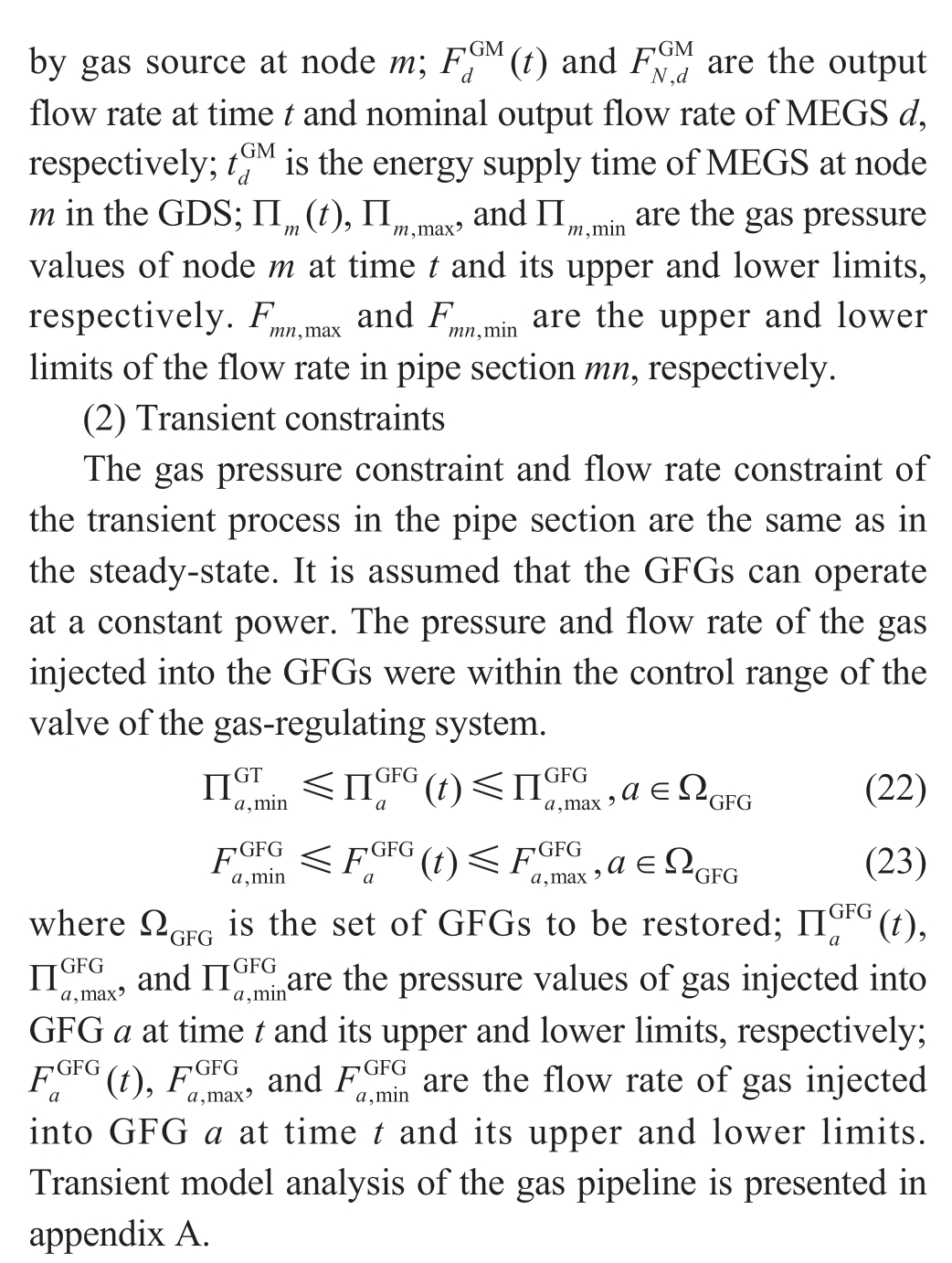
2.2.3 Constraints of mobile emergency sources
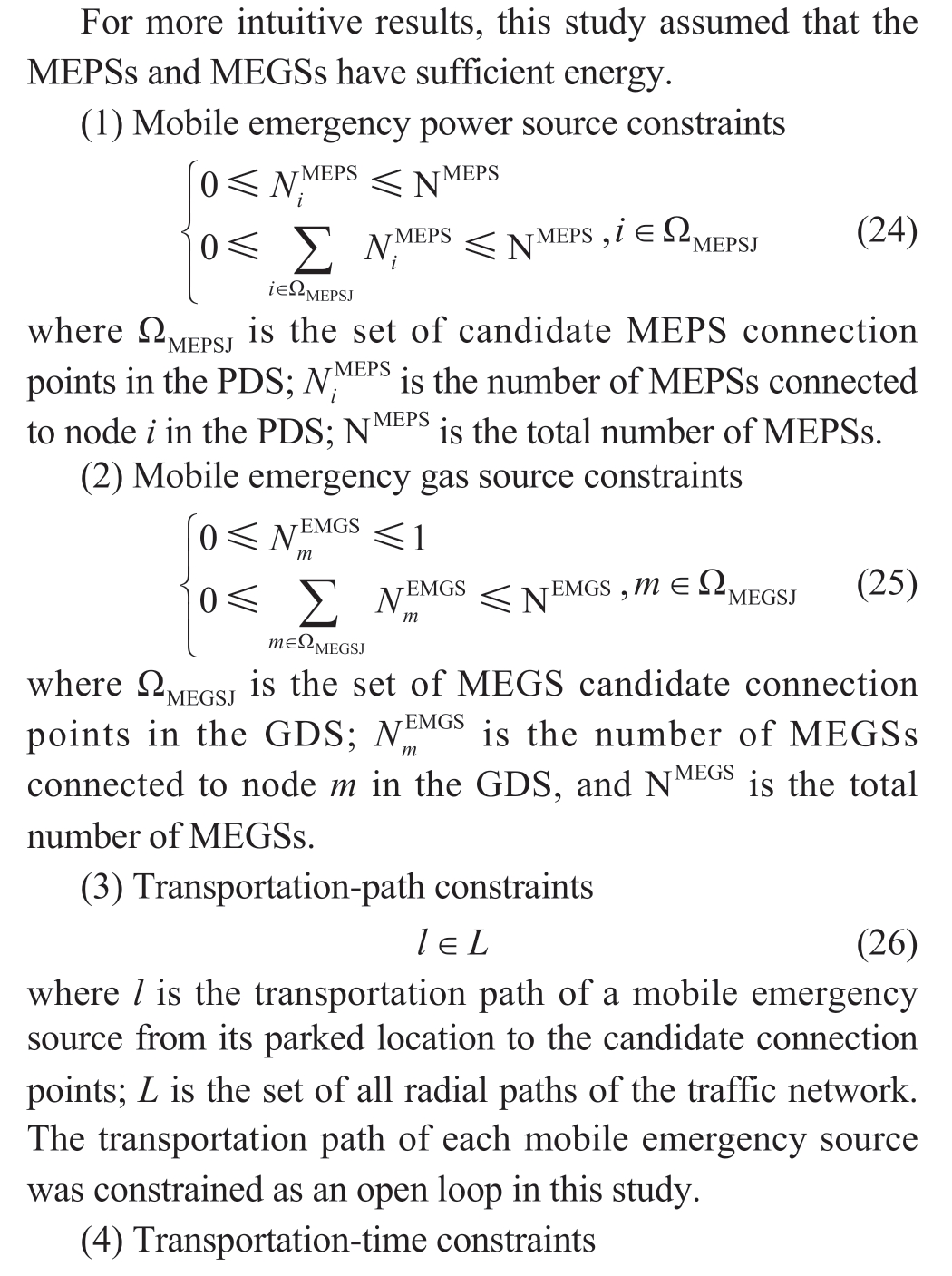
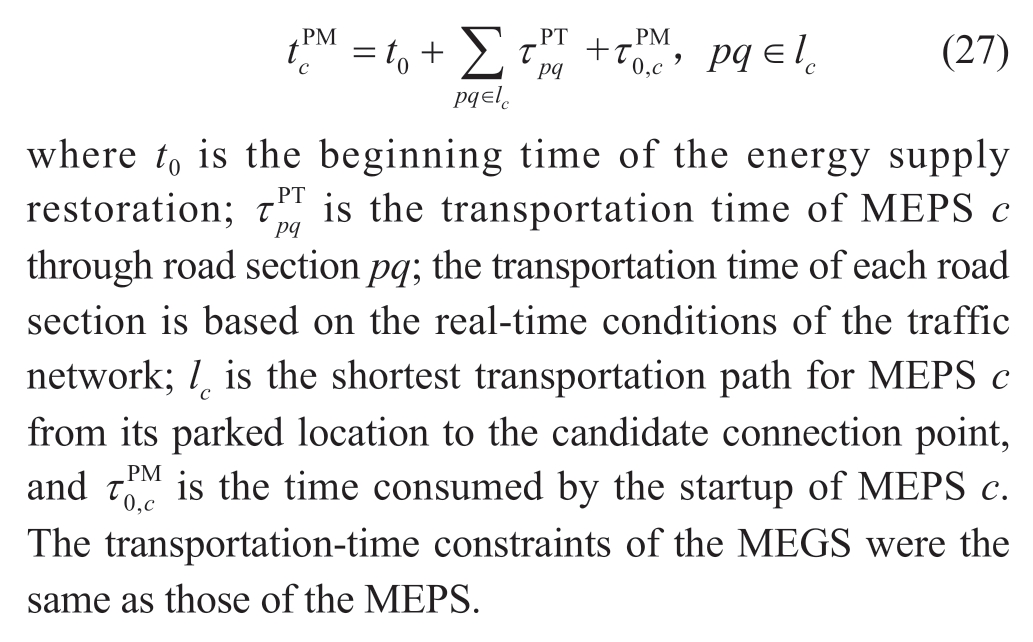
3 Coordination and optimization method of energy supply restoration
Due to the slow transmission speed of natural gas,in order to avoid a long transient process,independent parallel restoration of small-scale GDSs formed by gas sources is considered in this study.The optimal decision-making process for the energy supply restoration of the MG2PIES was established to solve the model shown in equation (1),as shown in Fig.2.
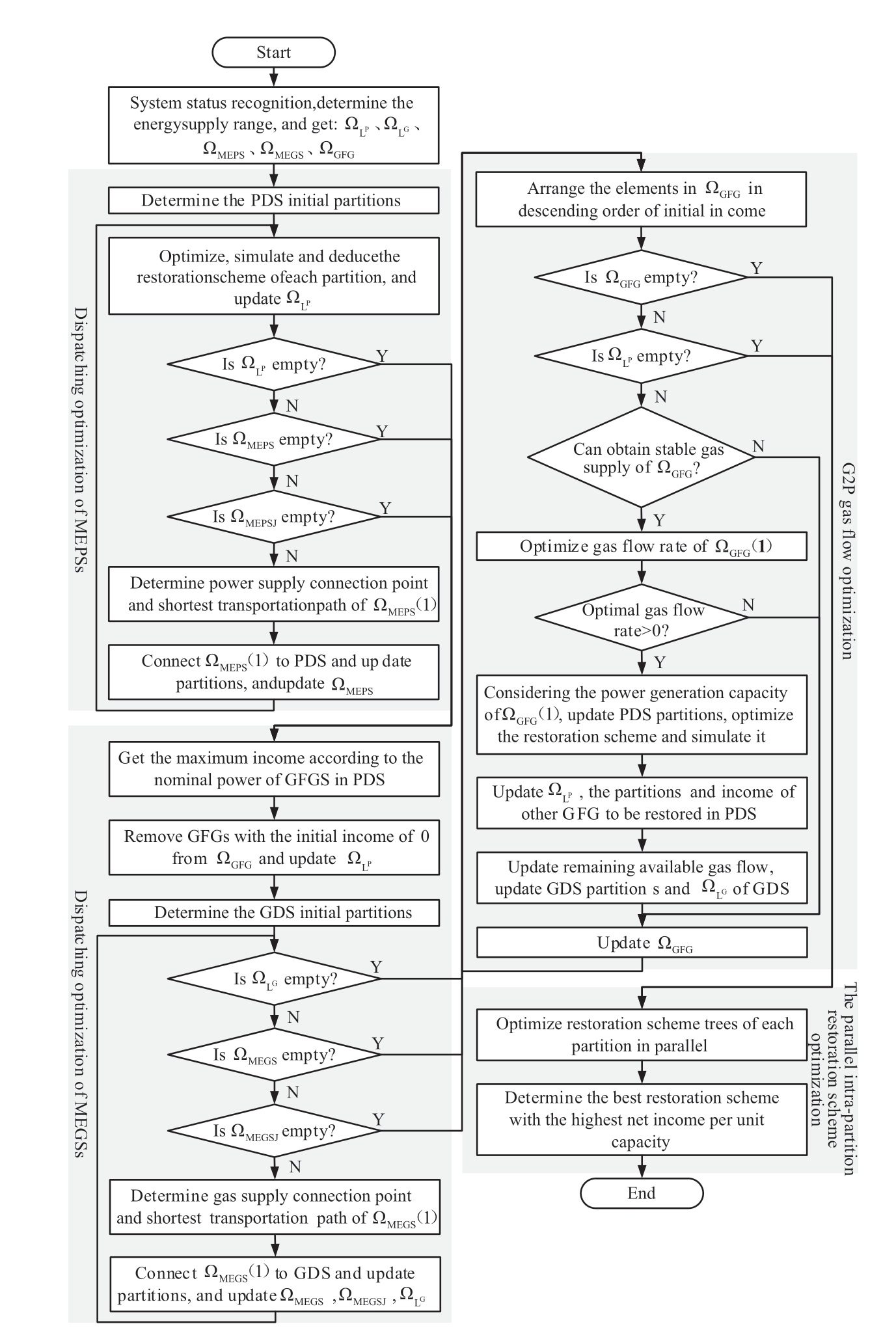
Fig.2 Optimization decision-making process of MG2PIES energy supply restoration
First,system status recognition is carried out to identify potential restorable regions that refer to outage regions where there is at least one restorable path between the node of the region and any energized node in the MG2PIES.Inside the potential restorable region,the status of the loads to be restored,energy supply sources,branches,and switches are analyzed to identify ΩLP and ΩLG,the set of power loads to be restored,and the set of gas loads to be restored,respectively.
Second,mobile emergency source dispatch optimization was performed.In this process,the restoration partitions of each fixed source in the PDS are first divided and the restoration scheme is simulated until the fixed power sources are no longer available.Subsequently,the MEPS available capacity,transportation path,and delivery time of each candidate connection point were determined to maximize the income of electric power load restoration according to the MEPS nominal power and real-time traffic conditions.On this basis,the maximum income that can be obtained in the PDS of each GFG to be restored was calculated according to the nominal power of the GFGs and taken as the estimated gas-load restoration profit of the GFG in the GDS.The MEGS available capacity,transportation path,and delivery time of each candidate connection point were determined to maximize the gas-load restoration income according to the nominal flow of the MEGS and real-time traffic conditions.
Third,G2P gas flow optimization was carried out to optimize the gas flow rate GFG to be restored from the GDS,and the PDS and GDS partitions were updated.
Finally,parallel intra-partition restoration scheme optimization was performed to obtain the restoration amount and path of the load to be restored for each partition in PDS parallel partitions and GDS parallel partitions.
3.1 System status recognition
In the MG2PIES,the stable energy supply systems and power and gas sources during their restoration process are referred to as energy supply systems in this study.The available capacity of each energy supply system was analyzed,corresponding to the available power and electricity in the PDS and the available gas and flow rate in the GDS.The available power and gas flow rate in the PDS and GDS can be determined using the predicted maximum residual output power and flow rate of the power and gas distribution networks,respectively.Furthermore,the power supply range of the power distribution network is determined based on the available power,voltage level,and other factors.The gas supply range of the gas distribution network is determined based on the available flow rate and gas pressure level.
3.2 Mobile emergency source dispatching optimization
Considering that there are multiple candidate connection points for the MEPS and MEGS in the PDS and GDS,respectively,it is necessary to optimize the access points and capacity of mobile emergency sources.
(1) Dispatching optimization of MEPSs
The following steps are taken for dispatching optimization of MEPSs:
Step 1: Each energized power grid with available power determines the PDS initial partition.Considering the voltage overlimit problem,based on the power supply range of each initial partition in the PDS,each node in set ΩLP is uniquely divided into the nearest partition that can send power to it [30]to determine the parallel restoration partitions in the PDS.
Step 2: According to reference [31],calculate the restoration income of the restoration targets in each partition by considering the available power.Each partition prioritizes the supply of power to the restoration target with a high restoration income,and the restoration scheme of each partition is optimized,simulated,and deduced.Then,update ΩLP and determine whether ΩLP is an empty set.If it is empty,terminate the process; otherwise,proceed to Step 3.
Step 3: Determine whether sets ΩMEPSJ and ΩMEPS are empty,where ΩMEPS is the set of MEPSs;MEPS c∈ΩMEPS(c =1,2,...,NMEPS),if either ΩMEPSJ or ΩMEPS is an empty set,end the process; otherwise,arrange the elements in set ΩMEPS in descending order of nominal power and proceed to Step 4.
Step 4: Assume that the first element ΩMEPS(1) of set ΩMEPS is connected to one of the candidate connection points in set ΩMEPSJ.After ΩMEPS(1) is accessed,update the restoration partitions of the PDS and calculate the load restoration income and the shortest transportation path according to (28).All candidate connection points in set ΩMEPSJ are traversed to determine the maximum load restoration income.If the maximum load restoration income is less than or equal to 0,delete ΩMEPS(1) from set ΩMEPS,and proceed to Step 3; otherwise,the candidate connection point that can obtain the maximum load restoration income is determined as the optimal connection point of ΩMEPS(1) ,and proceed to Step 5.

where IM is the maximum net income of load restoration after the mobile emergency source is connected to the candidate connection point; βM(0 <βM ≤ 1) is the restoration income conversion coefficient of the candidate connection points for mobile emergency sources; IM,J and CM,J represent the restoration income and cost of mobile emergency sources supplying energy to the load to be restored at the candidate connection points,respectively.
Step 5: Connect ΩMEPS(1) to the PDS,update the partitions of the PDS,delete ΩMEPS(1) from ΩMEPS,and proceed to Step 2.
(2) Dispatching optimization of MEGSs
Considering that each candidate connection point in the GDS can access only one MEGS at most,the following steps were taken to optimize the dispatching of MEGSs.
Step 1: For each GFG in set ΩGFG,determine the initial partition of the GFG according to its connection in the PDS.The power supply range is determined according to the nominal power of the GFG.Each node in set ΩLP is uniquely divided into the nearest partition of the GFG that can send power to it.The maximum income that the GFG nominal power can receive in its initial partition is taken as its initial income in the PDS; the corresponding gas demand of the GFG is regarded as the gas load need to be supplied in the GDS.Remove GFGs with an initial income of 0 from set ΩGFG,and update set ΩLP.
Step 2: Each operating gas supply system with available gas flow determines the GDS initial partition.Considering node gas pressure limits,based on the gas supply range of each initial partition in the GDS,each node in set ΩLG is uniquely divided into the nearest partition that can send gas to it.
Step 3: Determine whether sets ΩMEGSJ and ΩMEGS are empty,where ΩMEGS is the set of MEGSs;MEGS d∈ΩMEGS(d=1,2,...,N M EGS); if either ΩMEGSJ or ΩMEGS is an empty set,end the process; otherwise,arrange the elements in set ΩMEGS in descending order of nominal flow and proceed to Step 4.
Step 4: Assume that the first element ΩMEGS (1) of set ΩMEGS is connected to one of the candidate connection points in set ΩMEGSJ.After ΩMEGS (1) is accessed,update the restoration partitions of the GDS and calculate the load restoration income and the shortest transportation path according to (28).All candidate connection points in set ΩMEGSJ are traversed to determine the maximum load restoration income.If the maximum load restoration income is less than or equal to 0,delete ΩMEGS (1) from set ΩMEGS,and proceed to Step 3; otherwise,the candidate connection point that can obtain the maximum load restoration income is determined as the optimal connection point of ΩMEGS (1),and proceed to Step 5.
Step 5: Connect ΩMEGS (1) to the GDS,update the GDS partitions,delete ΩMEGS (1) from ΩMEGS,and delete the corresponding candidate connection points from ΩMEGSJ.Determine whether set ΩLG is empty; if so,terminate the process; otherwise,proceed to Step 3.
3.3 G2P gas flow optimization
During the PDS power supply restoration process,whether the GDS supports gas flow from FGFG to the PDS through GFG conversion depends on the comparison of the conversion gas maximum net incomes earned from the PDS and GDS during the assessment period.As shown in Fig.3,are the FGFG maximum net income curves obtained from the PDS and GDS,respectively.When the total available flow of the GDS is fixed,the gas load supplies ∆FGFG in the GDS from F1 to F1',and increases the gas supply ∆FGFG to the GFG,through which the gas is converted into electricity in the PDS from F2 to F2',such that the restored income of the GDS reduces
from I1 to I1',and the restored income of the PDS increases
from I2 to I2'.As long as
,the total available flow of the GDS can obtain a higher income.Considering the efficiency and loss of G2P conversion,the G2P flow optimization decision is considered only when the load to be restored in the PDS exceeds its available power.
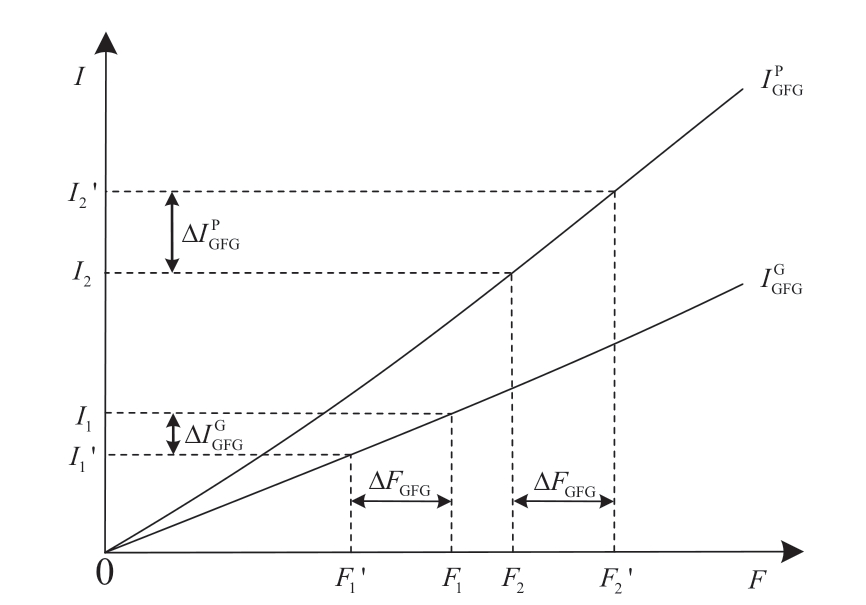
Fig.3 Diagram of G2P gas flow optimization
The objective function for G2P flow optimization is established as

whereis the difference between the FGFG maximum net income obtained from the PDS and that obtained from the GDS.If
,the GDS does not consider supplying gas to the GFG for electricity generation.
The following steps are proposed to make the G2P flow optimization decision.
Step 1: Arrange the elements in set ΩGFG in descending order of the initial income of the GFG.Determine whether ΩGFG is an empty set; if ΩGFG is empty,terminate the process; otherwise,proceed to Step 2.
Step 2: Determine whether the PDS still has nodes to be restored.If ΩLP is an empty set,terminate the process;otherwise,proceed to Step 3.
Step 3: If a GFG in set ΩGFG cannot obtain stable gas supply; that is,the available flow rate supplied by the gas distribution network in the partition with the GFG is less than the lower limit of its nominal operating flow,the GFG is removed from ΩGFG.Determine whether ΩGFG is an empty set; if it is empty,terminate the process; otherwise,proceed to Step 4.
Step 4: Identify the path HGFG(1) by which ΩGFG (1) can obtain the gas supply from the gas supply system with the shortest time delay.The maximum available flow rate of ΩGFG (1) is ,where ΩGFG (1) is the first element of ΩGFG,
is the available flow rate of the gas supply system,and
is the nominal flow rate of ΩGFG (1).
Step5:Assume that the maximum net incomes of the gas flow rate obtained by ΩGFG (1) in its PDS partition and GDS partition are ,respectively.Optimize the gas flow rate FGFG(1) obtained by ΩGFG (1) based on (29).If FGFG(1) =0,delete ΩGFG (1) from set ΩGFG and proceed to Step 1; otherwise,determine the gas supply system plan to supply gaswith a flow rate of FGFG(1) to ΩGFG (1),and proceedto Step6.
Step 6: Considering the power generation capacity of ΩGFG (1) after obtaining the gas flow rate FGFG(1),update the restoration partition of the PDS,optimize the restoration scheme of the PDS,and simulate it.Update ΩLP,and update the restoration partition and initial income of the other GFGs to be restored in the PDS.Update the remaining available gas flow,restoration partitions,and ΩLG of the GDS.Delete ΩGFG (1) from ΩGFG and proceed to Step 1.
3.4 Parallel intra-partition restoration scheme optimization of PDS and GDS
The PDS and GDS partitions generated in the previous steps are optimized using the breadth deduction method suggested in reference [30].The restoration scheme trees of each partition were simultaneously optimized in parallel.
In the presented optimization strategy,there is a combination explosion problem; it is necessary to balance the optimization effect and the calculation burden.To solve this problem,the number of scenarios can be reduced by screening the restoration targets,limiting the breadth and depth of the tree,and limiting the number of schemes for the same restoration target (refer to reference [30] for specific methods).
4 Simulation results and analysis
4.1 System description
In this study,an MG2PIES was established,as shown in Fig.4,including an 81-node medium-voltage PDS and an 89-node medium-pressure GDS [30].Residential areas are shaded in blue,and the white areas between residential areas are traffic sections.In the PDS,black-start units DG1-DG4 were located at nodes P9,P65,P16,and P72,with nominal capacities of 15,15,6,and 6 MW,respectively;the generation cost was 500 yuan/MWh.DG3 and DG4 were GFGs,and the upper and lower limits of the operating gas flow were2,000and 200m3/h,respectively.The parameters are presented in Table B1 in Appendix B.Node P81 is the secondary-side bus of the step-down transformer.Assuming that all power loads are controllable,the load demand of each node in the PDS is 1 MW.The power load importance is divided into grades 1–5; the corresponding unit restoration income of the load is 10,5,2,1,and 0.1 thousand yuan/MWh,respectively,(refer to Table B2 in Appendix B for information).In the GDS,the gas distribution station and gas wells S1 and S2 are connected to nodes G81,G84,and G87,respectively,with available flow rates of 4,500,3,000,and3,000 m3/h,nominalgaspressures of0.3,0.2,and 0.2MPa,andgascosts of4,5,and 6yuan/m3,respectively.C1 between nodes G85 and G86,C2 between nodes G88 and G89,and C3 between nodes G82 and G83 are compressors with a compression ratio of 1.2.Assume that the length of each pipe section between two nodes is 2 km and the pipe diameter is 100 mm.Assuming that the gas loads of nodes G1–G80 are all controllable,the load flow demand of each load is 250 m3/h.The gas load importance is divided into grades 1–5; the corresponding restoration unit income of each load is 200,100,40,20,and 10 yuan/m3,respectively(see Table B3 in Appendix B for information).
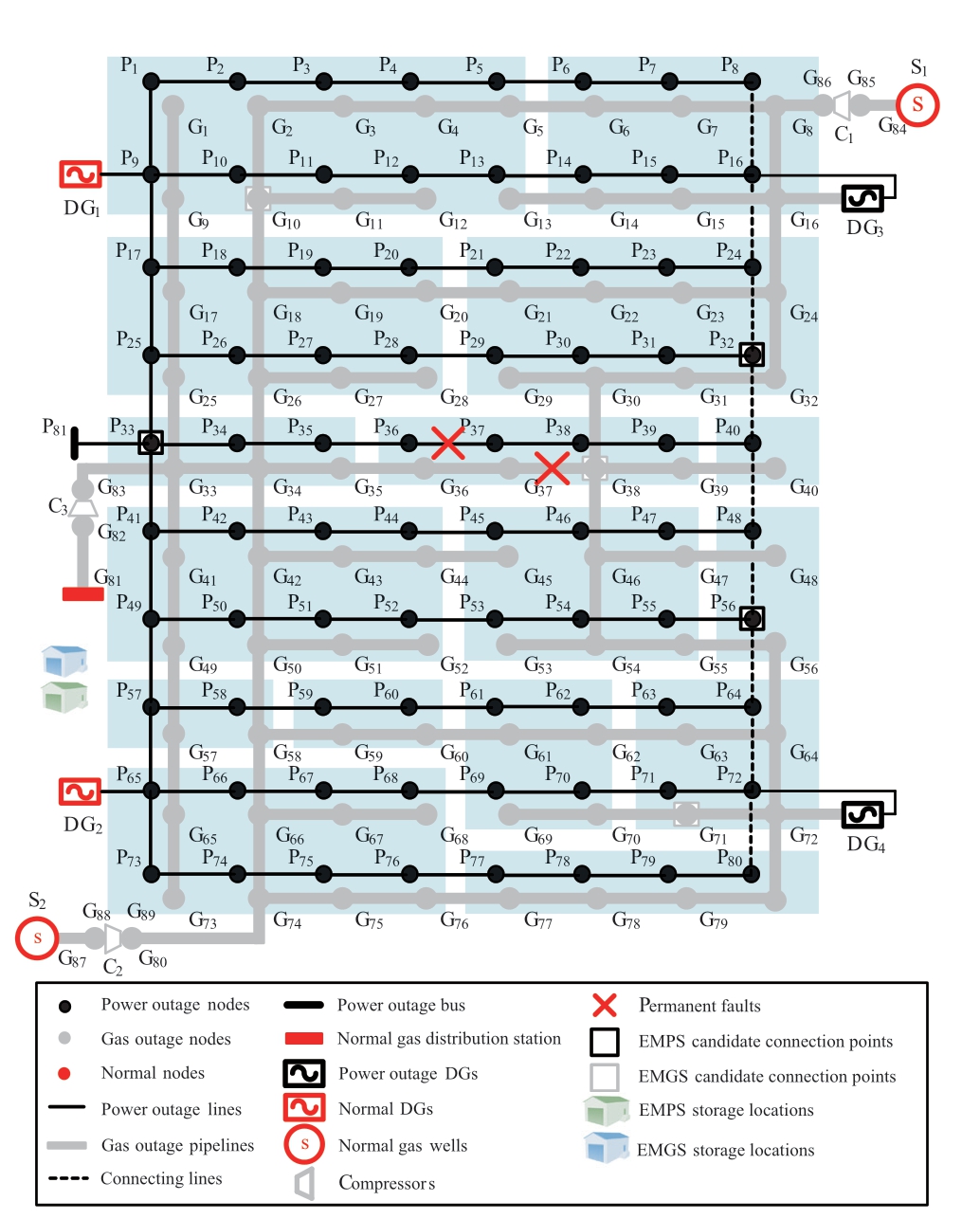
Fig.4 81–89 node MG2PIES
Table B1 Parameters of units
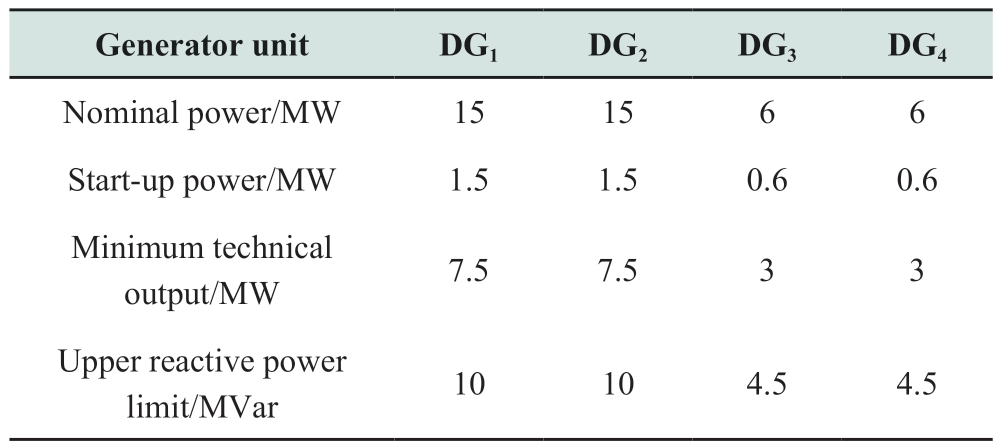
continue
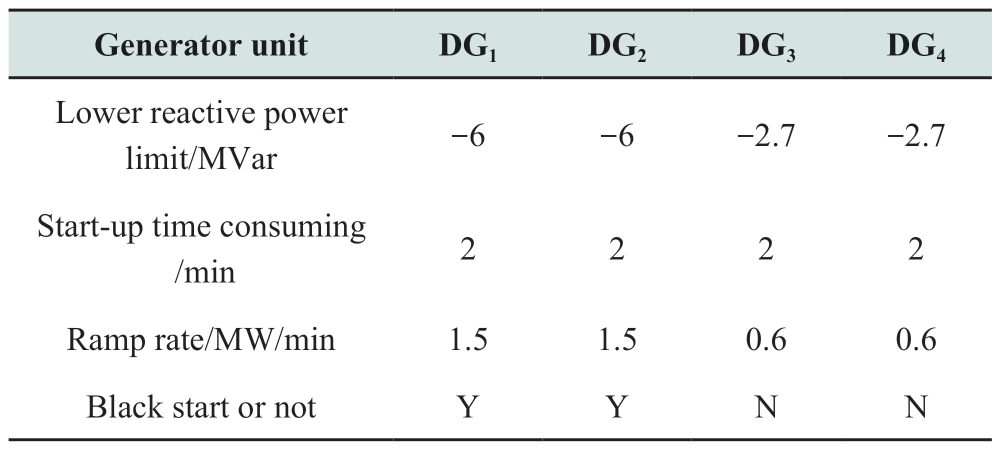
Table B2 Power loads importance information
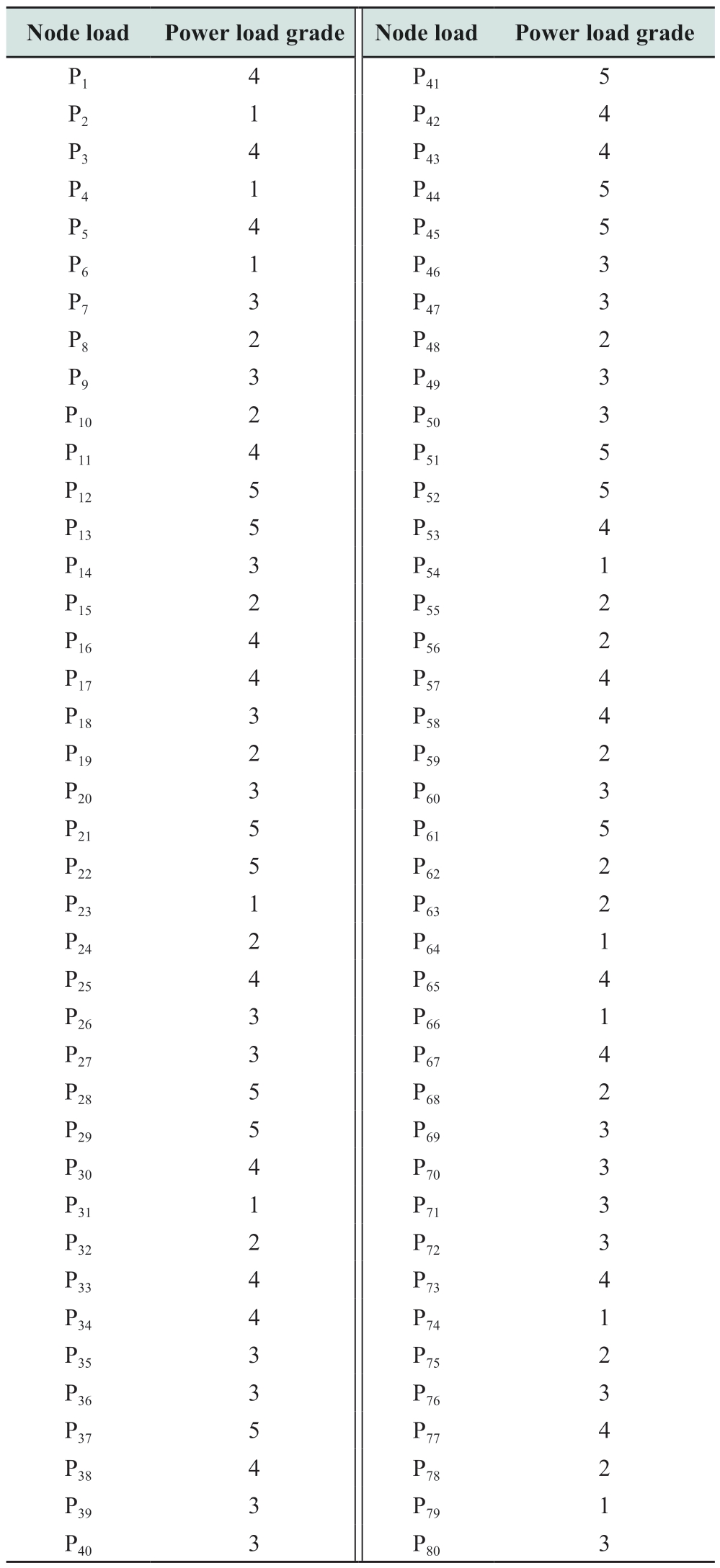
Table B3 Gas loads importance information
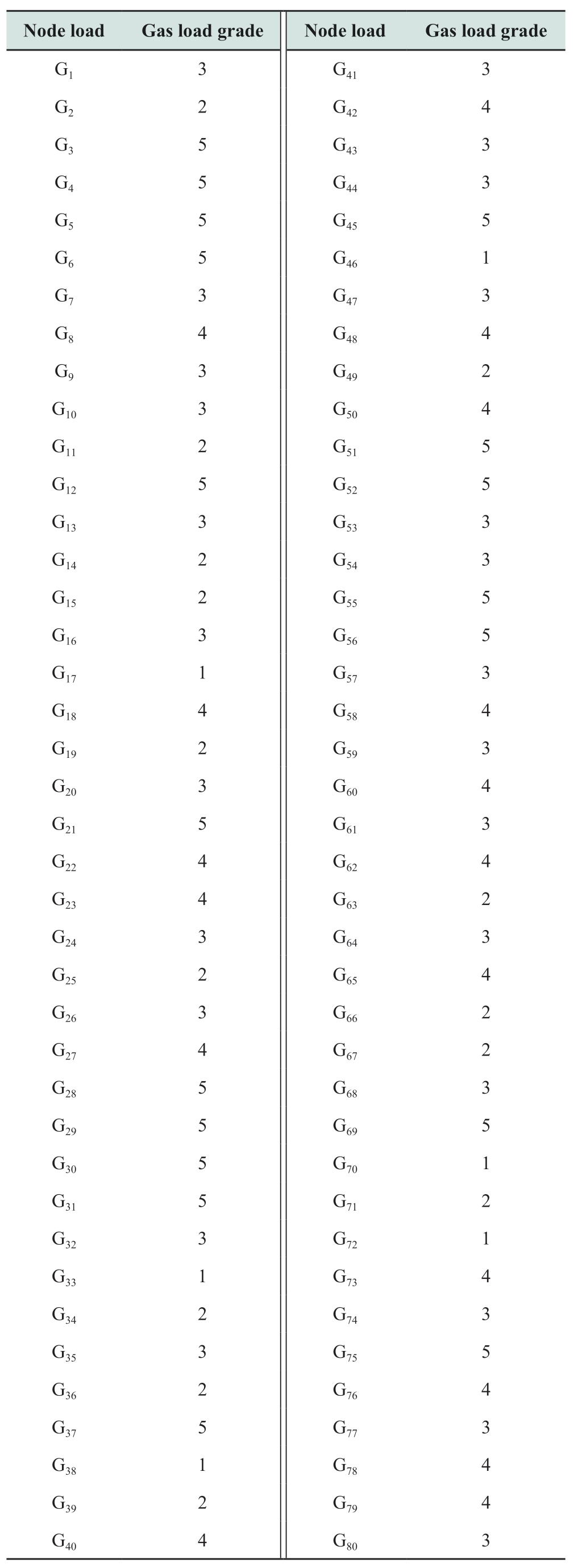
We assumed two identical MEPSs in this area.The nominal power of each MEPS was 6 MW,the start-up time was 2 min,and the power supply cost was 600 yuan/MWh.There were two identical MEGSs; the nominal flow rate of each MEGS was 1,500 m3/h; the nominal gas pressure was 0.2 MPa; the start-up time was 2 min,and the gas supply cost was 6 yuan/m3.The MEPS and MEGS parks were adjacent to each other and the transportation cost was 800 yuan/km.Because traffic conditions change dynamically,the congestion level of the road section was set to 1 for unblocked traffic and 2 for congested traffic; the speed of the MEPSs and MEGSs passing through unblocked sections was approximately 60 km/h,and the speed of passing through congested sections was approximately 30 km/h.The road section parameters are presented in Table B4 in Appendix B.The transportation path was determined according to the shortest transportation time.
Table B4 Road section parameters
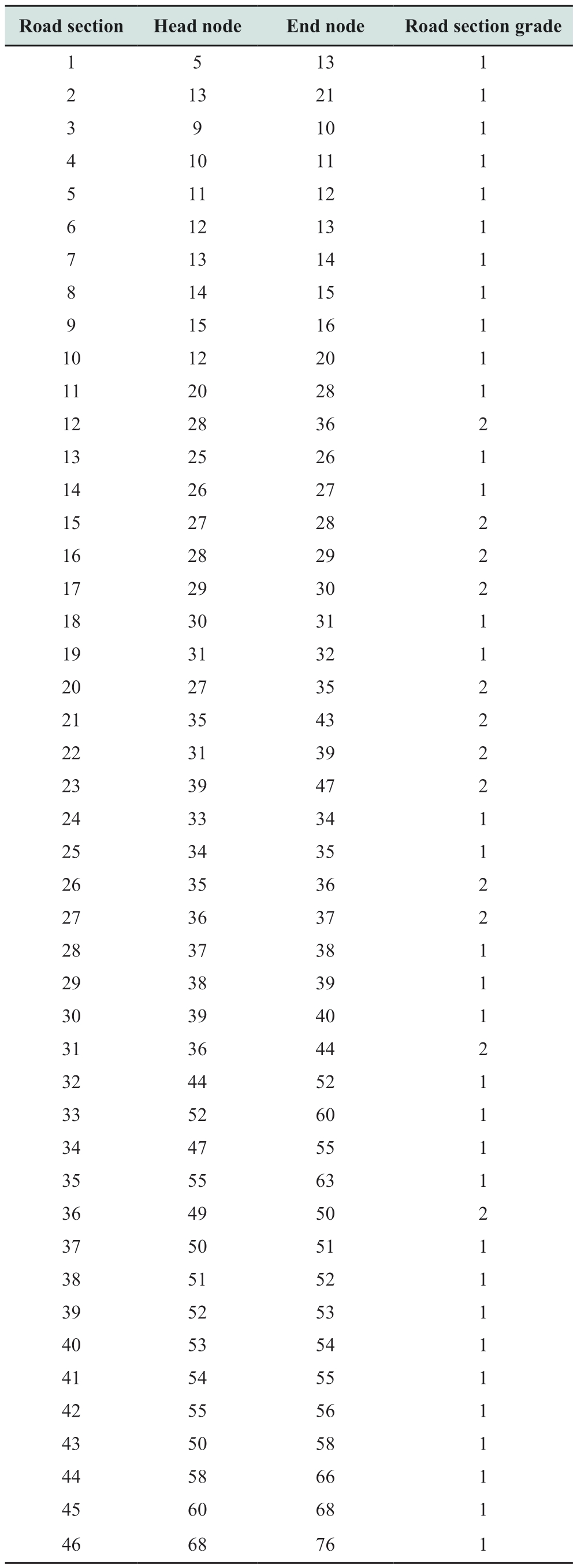
continue
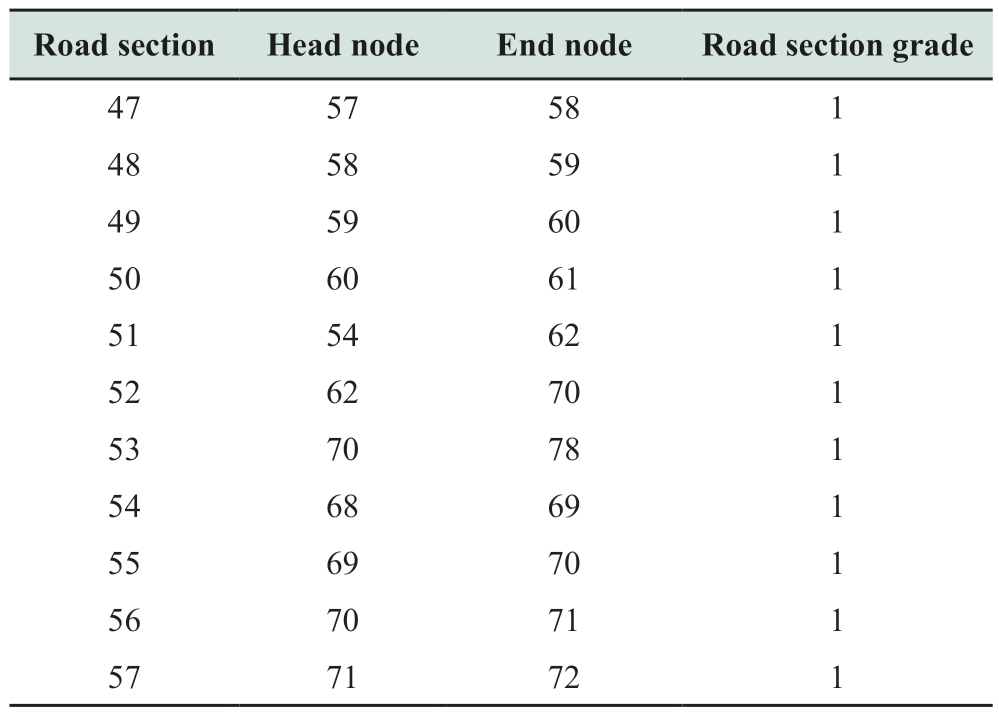
Suppose that the load supply of the MG2PIES is interrupted at 00:00:00,at the beginning of restoration.The line between nodes P36 and P37 in the PDS,and the pipeline between nodes G37 and G38 in the GDS are permanently faulty.Due to loss in the energy transmission process,βM was set to 0.95.The end time of the MG2PIES restoration decision was set to 03:00:00.
4.2 Optimization of restoration scheme of MG2PIES
At 00:00:00,suppose that DG1 and DG2 in the PDS start successfully,the gas distribution station and gas wells S1 and S2 in the GDS return to normal,and the entire system starts to restore.Because the GDS has a large number of gas loads to be restored,whether DG3 and DG4 start depends on the optimization of their gas flow.The optimization process for the restoration scheme of the MG2PIES is described as follows:
(1) MEPS and MEGS dispatching optimization
Determine the partitions for DG1 and DG2 in the PDS,denoted as Partition 1 and Partition 2,and optimize the restoration scheme of Partitions 1 and 2,respectively,as shown in Fig.5.Fig.5 was obtained by expanding the spatially overlapping PDS and GDS in Fig.4 with their coupling links DG3 and DG4 as the axis; the connection relation of the components in the system in Figs.4 and 5 remained unchanged.After simulated implementation of the restoration schemes of Partitions 1 and 2,there are still nodes to be partitioned in the PDS.Thus,the MEPSs are considered to restore the power supply in the PDS.
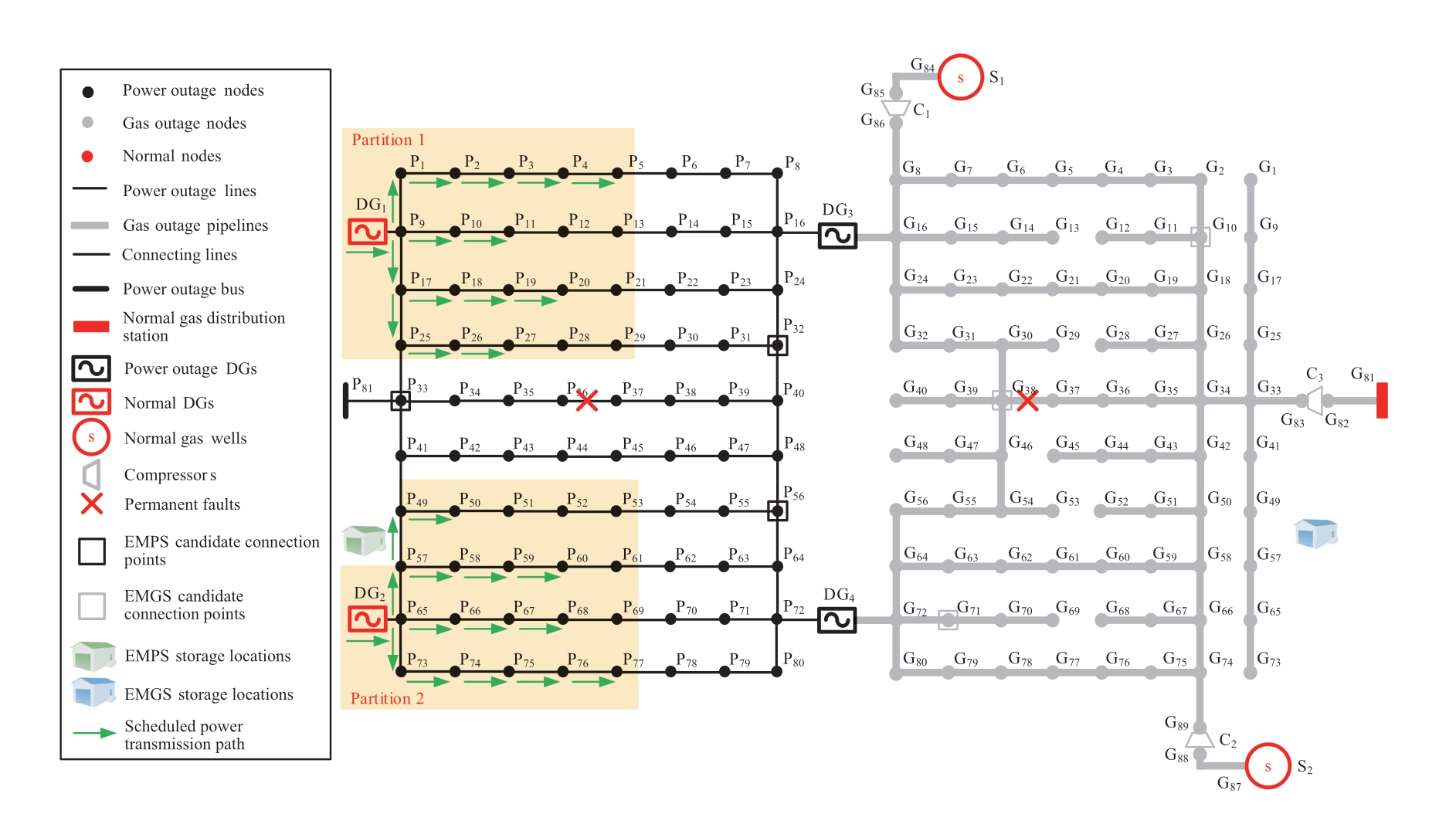
Fig.5 Restoration scheme for Partition 1 and Partition 2
The available power of the MEPS candidate connection points P32,P33,and P56 is taken as the nominal power of the MEPS (6 MW).The partitions of each candidate connection point are determined (Partitions 3–5),as shown in Fig.B1 in Appendix B,with restoration incomes of 791.78,172.63,and 1,003.27 thousand yuan,respectively.Accessing the MEPS to connection point P56 obtains the maximum restoration income.Thus,P56 is first chosen for MEPS access to form Partition 3,and the restoration scheme of Partition 3 is optimized for the available power of the MEPS.After the simulation and deduction,the PDS nodes to be partitioned are updated.Using the same method,the access location of the second MEPS is optimized as P32,forming Partition 4; the restoration scheme within the partition is simulated and deduced; the results are shown in Fig.6.
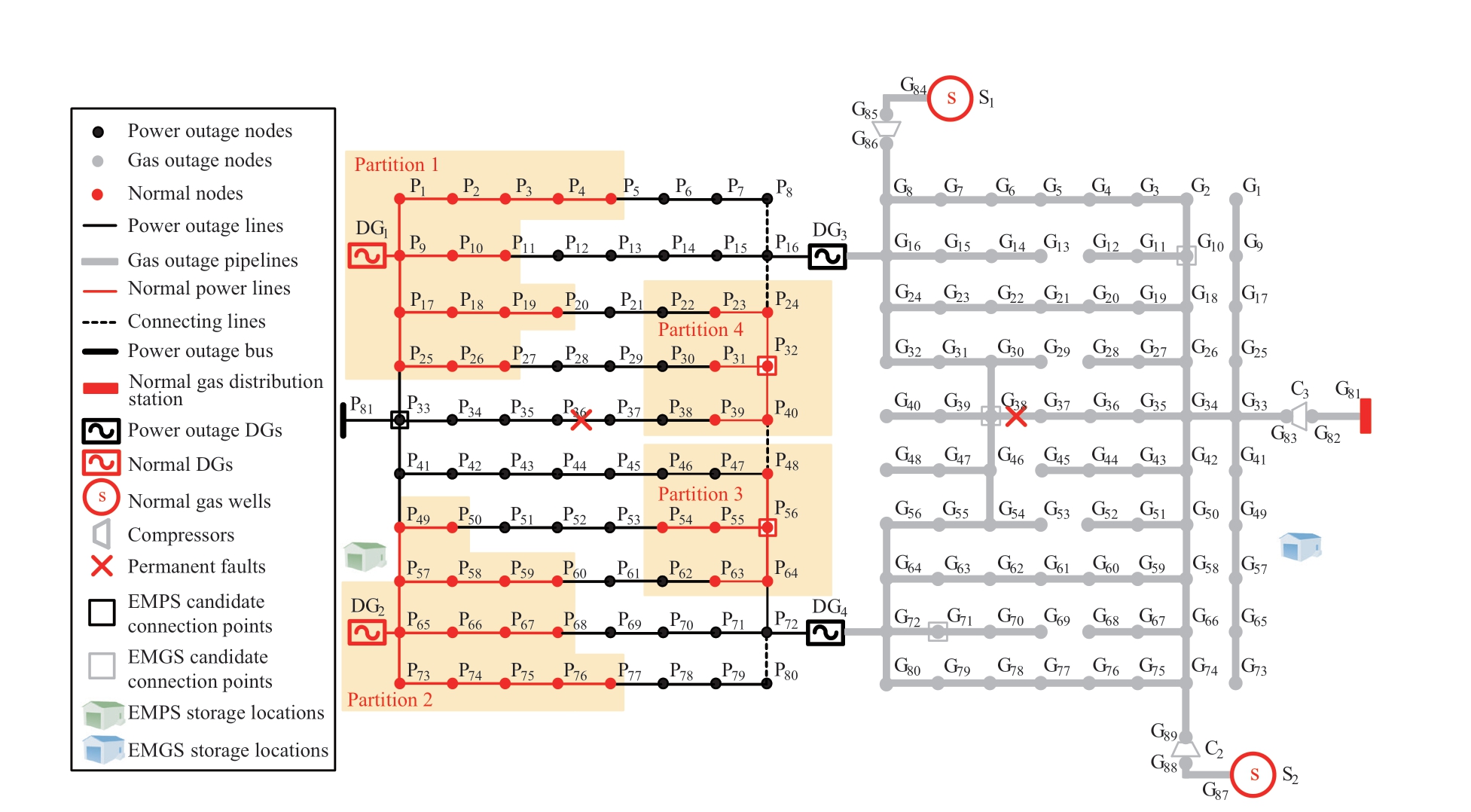
Fig.6 Restoration scheme simulation and deduction for Partitions 1–4
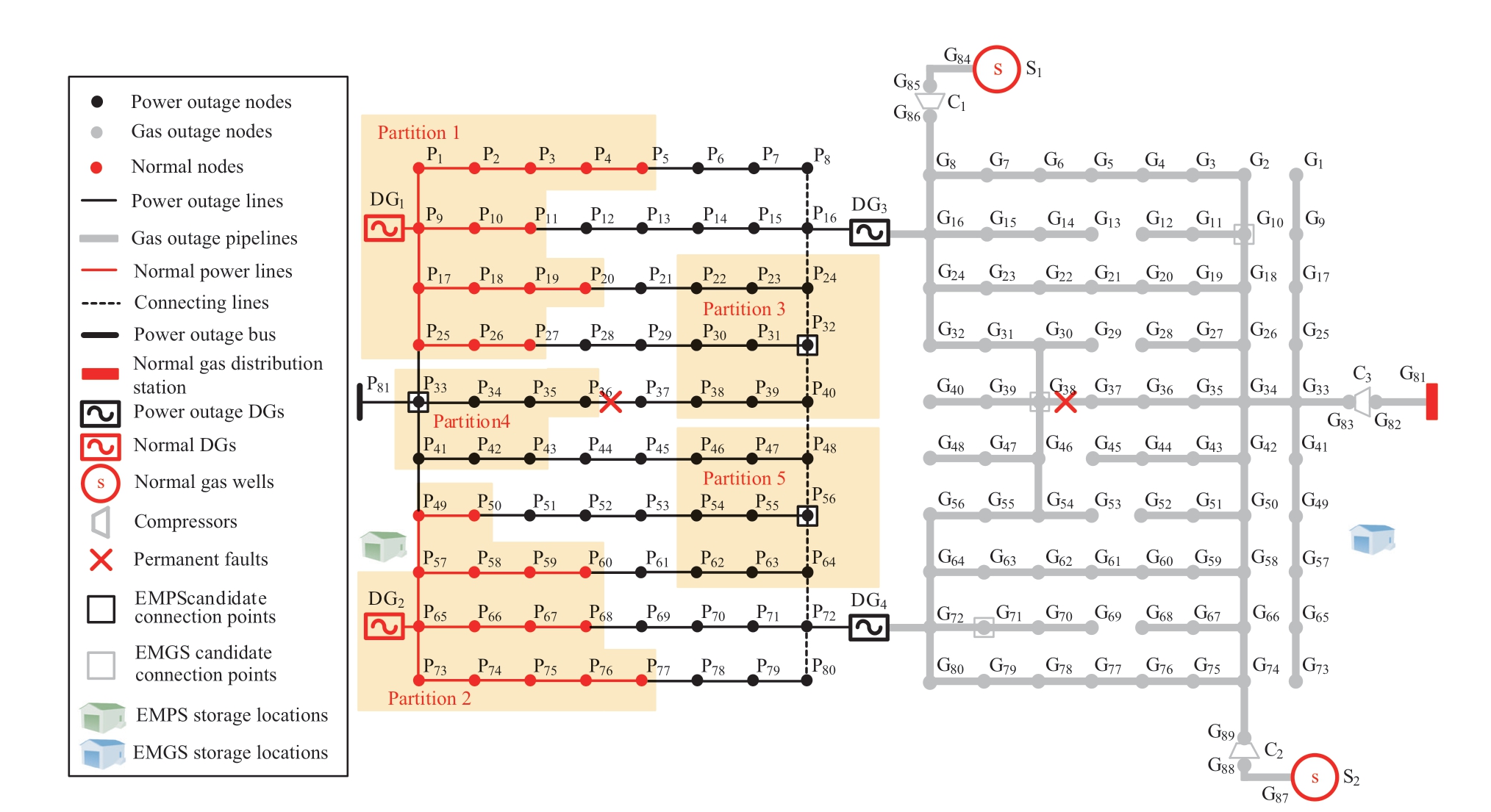
Fig.B1 Partitions of MEPS candidate connection points
As there are still nodes to be partitioned in the PDS,the possibility of restoring the power supply through the GFG was considered.The nodes to be restored in the PDS are uniquely divided into the nearest partitions supplied by DG3 or DG4; the nominal power of the GFG is the available power of the partition.Partitions of DG3 and DG4 are shown in Fig.B2 of Appendix B.The total power demand and the corresponding income of the loads to be restored in each GFG partition in the PDS were calculated.The initial restoration incomes of DG3 and DG4 were 670.73 and 526.91 thousand yuan,respectively,with corresponding estimated unit restoration incomes of 166.667 and 153.333 yuan/m3,respectively.The gain in such income depends on whether the GFG can obtain a gas supply from the GDS.
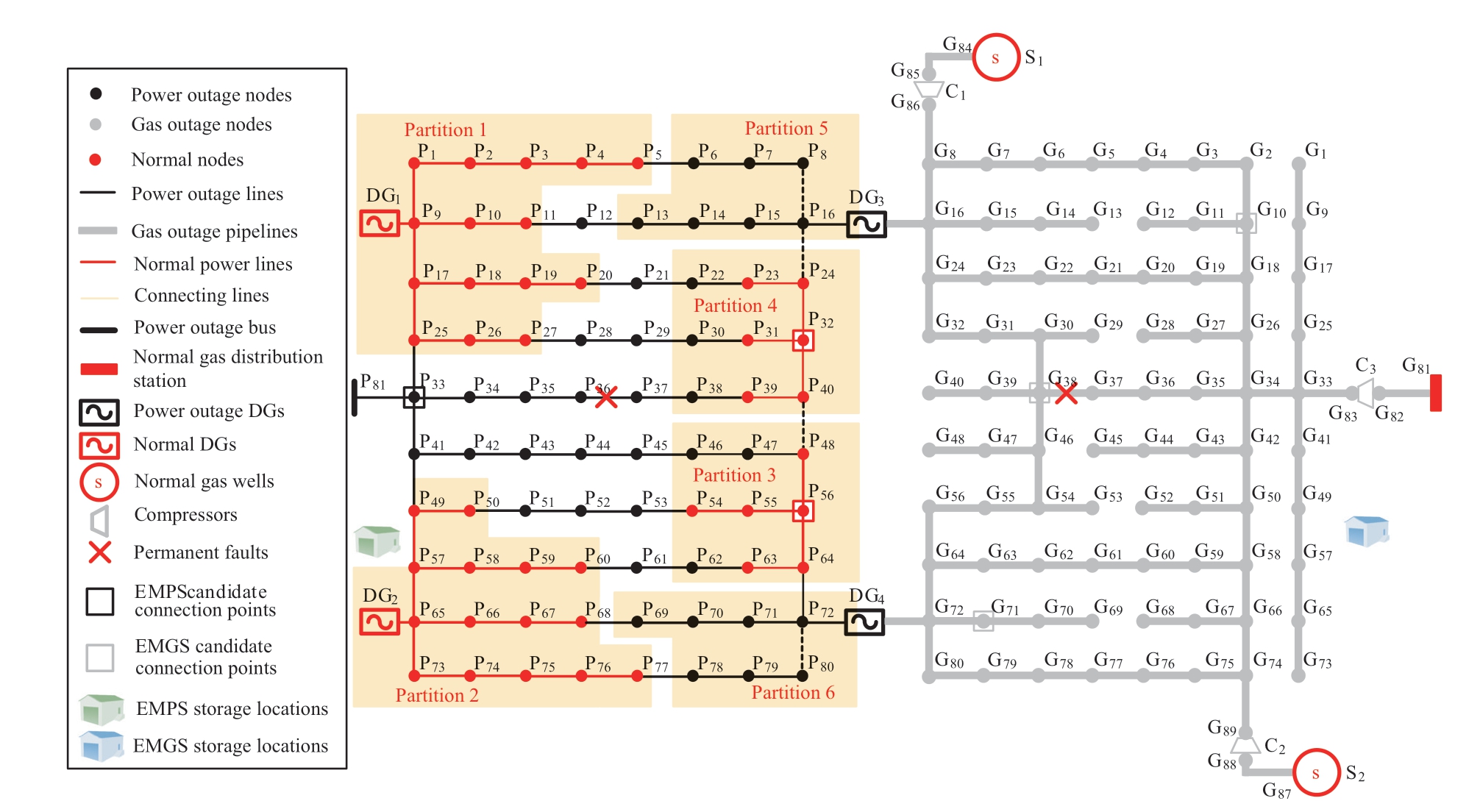
Fig.B2 Partitions of DG3 and DG4
Determine Partitions 7–9 for gas distribution stations S1 and S2 and optimize the restoration schemes of these partitions.The available gas flow of MEGS candidate connection points G10,G38,and G71 in the GDS is taken as the nominal gas flow of the MEGS (1,500 m3/h).The partitions of each candidate connection point (Partitions 10–12) are determined,as shown in Fig.B3 in Appendix B,with restoration incomes of 226.76,345.19,and 580.82 thousand yuan,respectively.Accessing the MEGS at connection point G71 yields the maximum restoration income.Thus,G71 is first chosen for MEGS access to form Partition 10 in Fig.7,and the restoration scheme of Partition 10 is optimized for the available power of the MEGS.After the simulation and deduction,the GDS nodes to be partitioned were updated.Using the same method,the access location of the second MEGS is optimized as G38,forming Partition 11 in Fig.7.The restoration scheme within the partition is simulated and deduced; the results are shown in Fig.7.
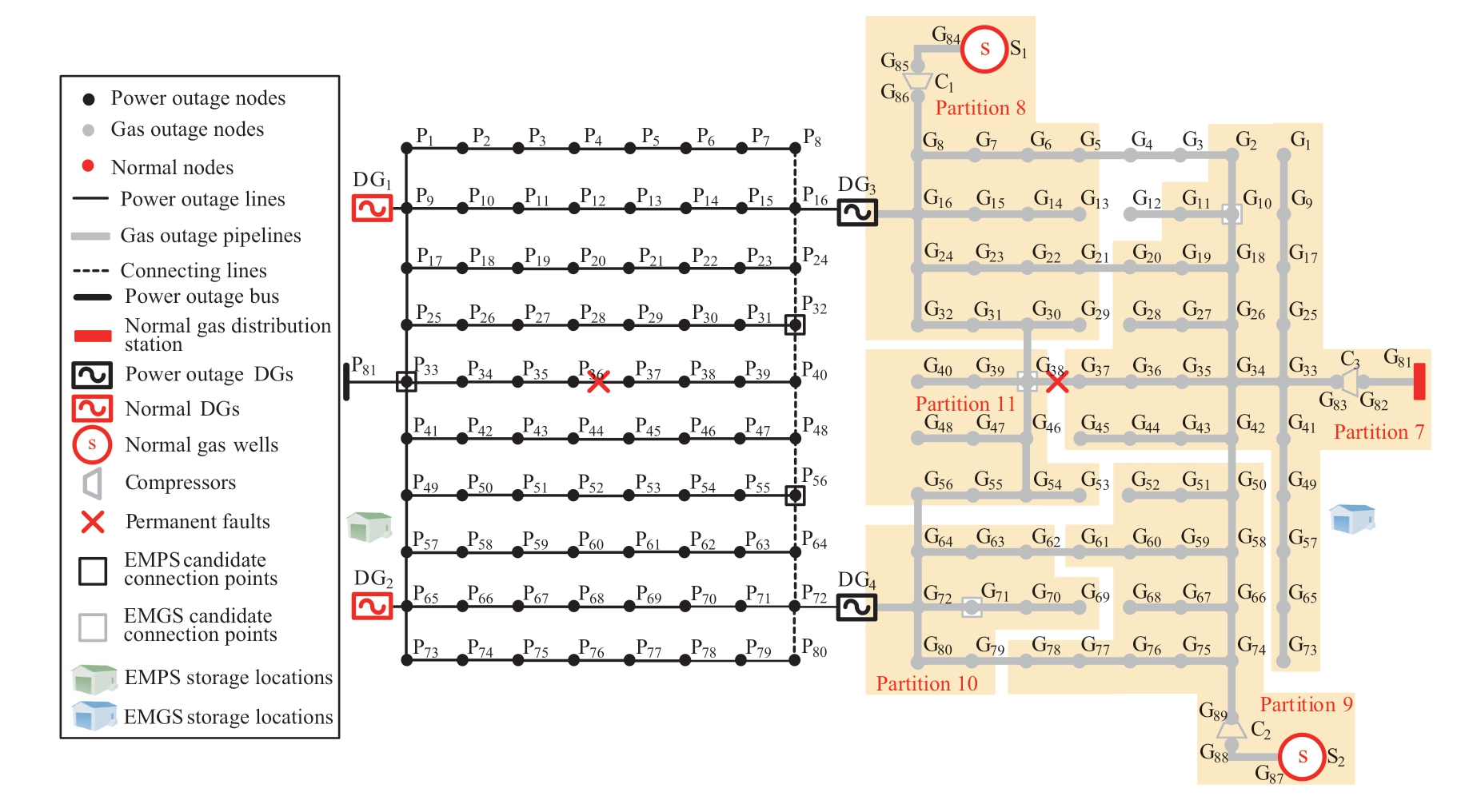
Fig.7 Update of GDS partitions
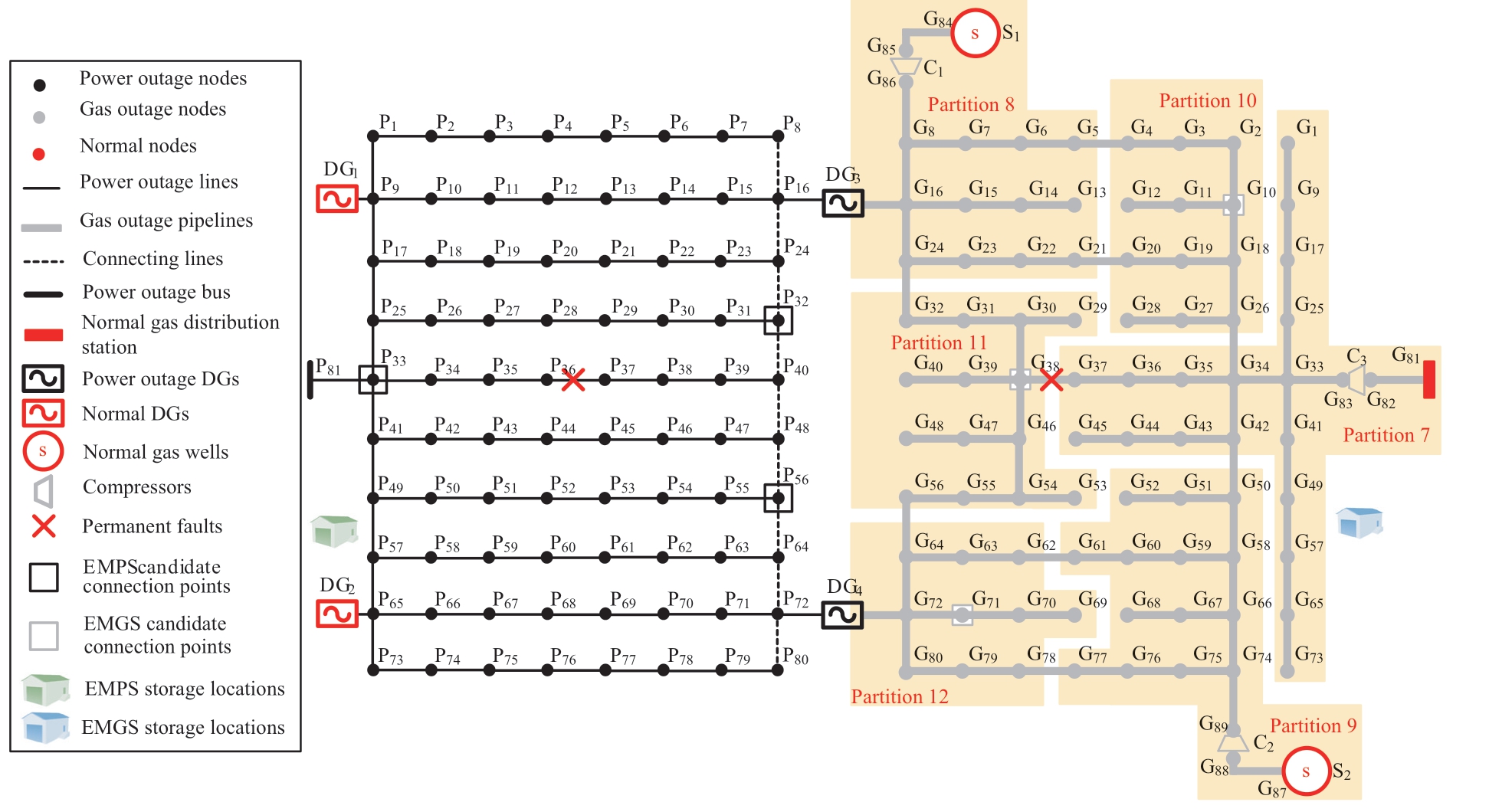
Fig.B3 Partitions of MEGS candidate connection points
(2) G2P gas flow optimization
According to the analysis,DG3 and DG4 obtain gas from S1 and G71 in the GDS,respectively,and form nonempty partitions in the PDS,which indicates that DG3 and DG4 can restore more power loads through G2P conversion.It is necessary to determine the optimal gas flow rate to obtain DG3 and DG4.According to the method described in Section 3.3,the optimal gas flow rate of DG3 with a higher initial income was first determined.According to the calculation,when S1 in the GDS supplies 1,000 m3/h of gas flow to DG3,4 MW of electric power can be converted through DG3,and the DG3 partition in the PDS receives the maximum restoration net income (592.77 thousand yuan).With the same gas flow in the GDS,the maximum restoration net income obtained by the S1 partition is 173.89 thousand yuan.At this time,the difference in the restoration net incomes of the PDS and GDS reaches its maximum positive value; thus,a gas supply flow rate of 1,000 m3/h is the optimal gas flow rate for DG3,and the optimal gas supply path is S1-G84-G85-G86-G8-G16-DG3.After simulating and deducing the restoration scheme for the DG3 partitioning in the PDS,the power loads at P6,P7,P8,and P15 were restored.
Similar to DG3,the optimal gas flow rate for DG4 was 500 m3/h,and the optimal gas supply path was G71-G72-DG4.After simulating and deducing the restoration scheme for the DG4 partitioning in the PDS,the power loads at P78 and P79 were restored.
Based on mobile emergency source dispatching optimization and G2P gas flow optimization,the partitions of the PDS and GDS are shown in Fig.B4 of Appendix B.In the PDS,priority is given to DG1 and DG2 to determine Partitions 1 and 2,respectively.The partitions for candidate connection points P32 and P56 are connected with the MEPSs as Partition 3 and Partition 4,respectively.DG3 and DG4 are divided into Partitions 5 and 6,respectively,according to the remaining nodes to be partitioned.In the GDS,the fixed gas sources connected to G81,S1,and S2,and candidate connection points G38 and G71 connected with the MEGS,are uniformly divided into Partitions 7–11,respectively.
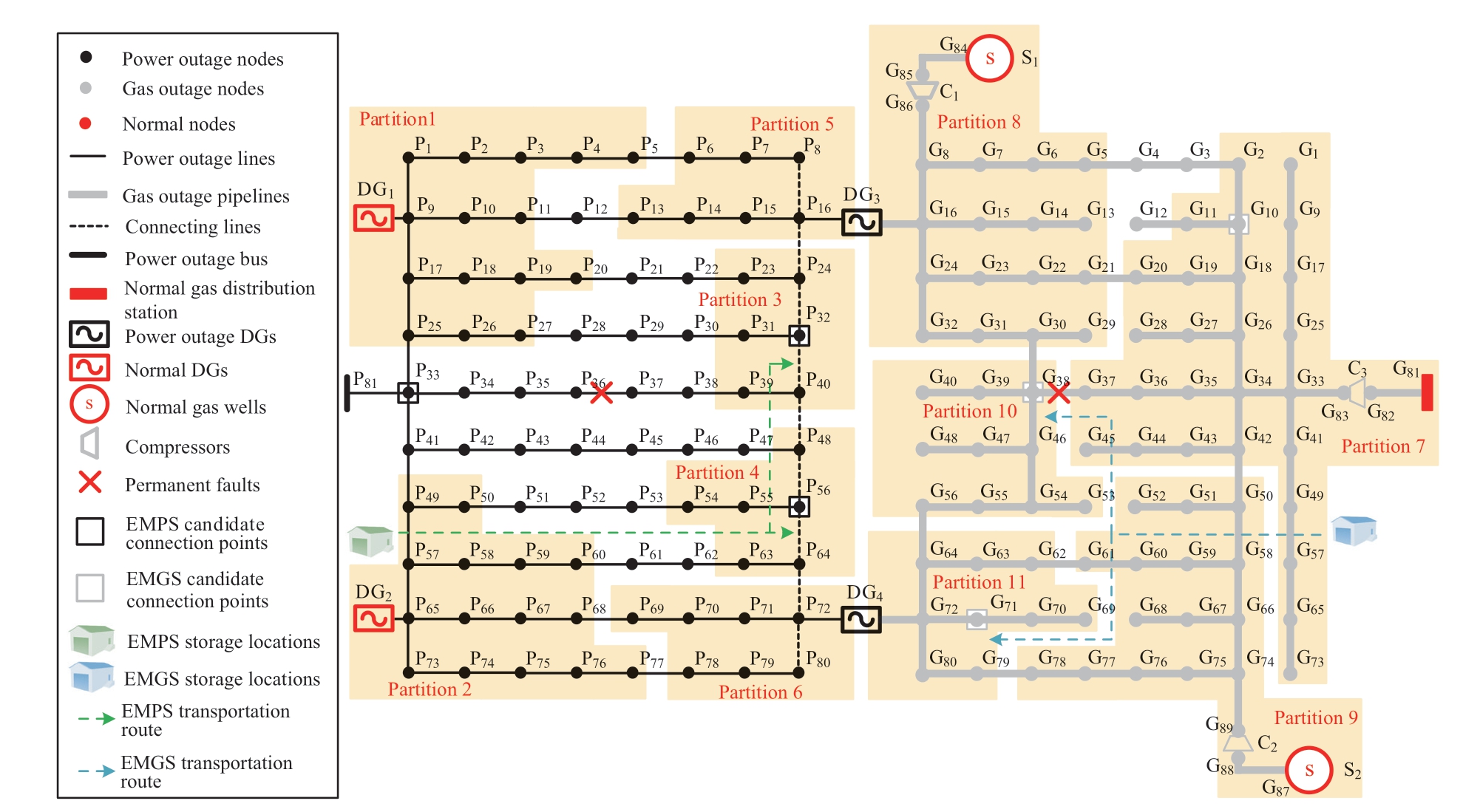
Fig.B4 Partitions of PDS and GDS
(3) Parallel intra-partition restoration scheme optimization of PDS and GDS
The restoration schemes for PDS and GDS partitions are shown in Fig.8; the optimal restoration schemes are shown in Table B5 of Appendix B.Eleven partitions execute their respective restoration schemes in parallel.At 00:28:31,the PDS restoration scheme is complete; the GDS restoration scheme is finished at 00:54:46.During the entire restoration process,the total restoration net income of the MG2PIES was 7.44 million yuan.Fig.B5 in Appendix B shows the restored system topology structure of the MG2PIES.
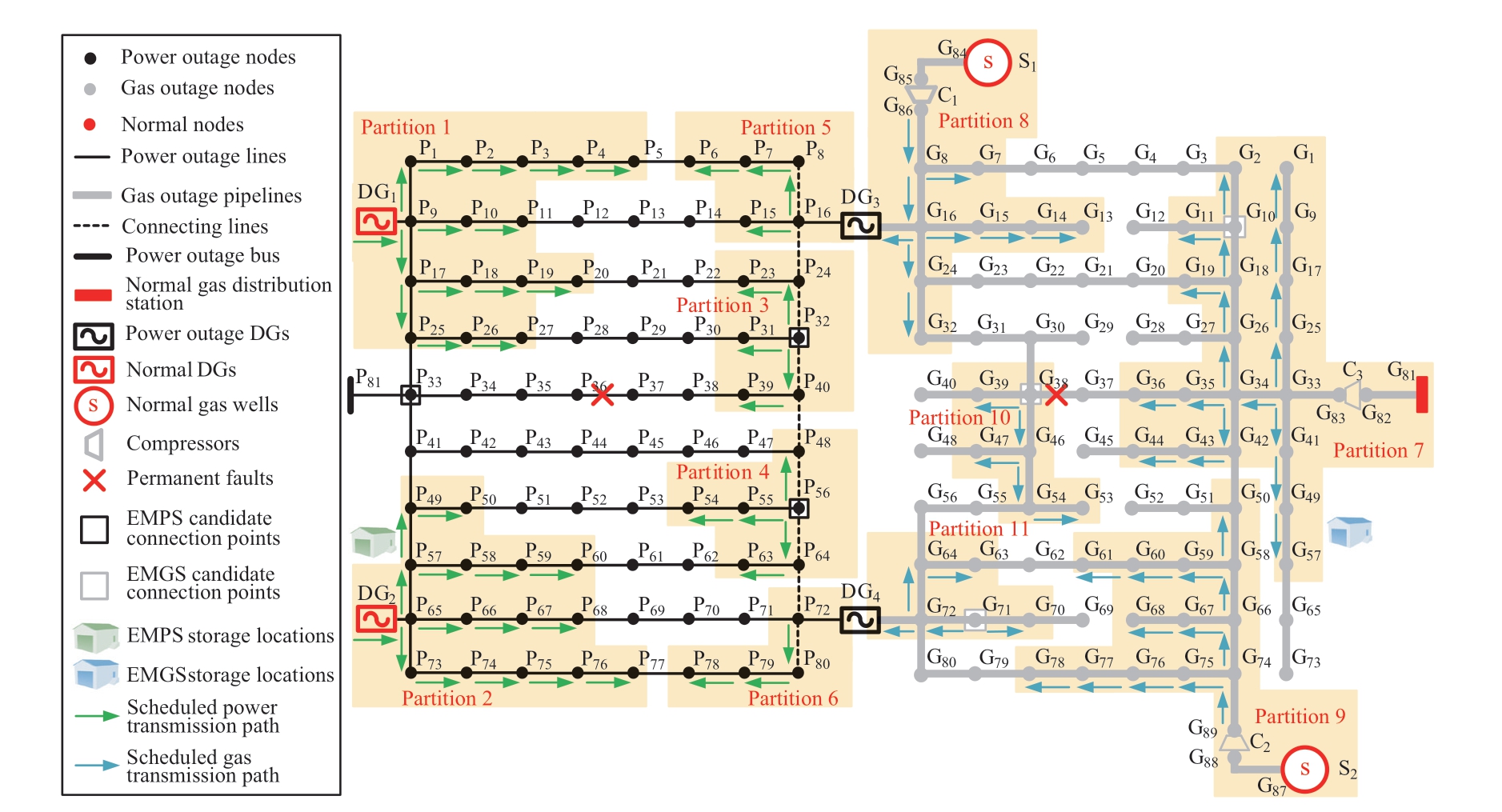
Fig.8 Restoration scheme for PDS and GDS partitions
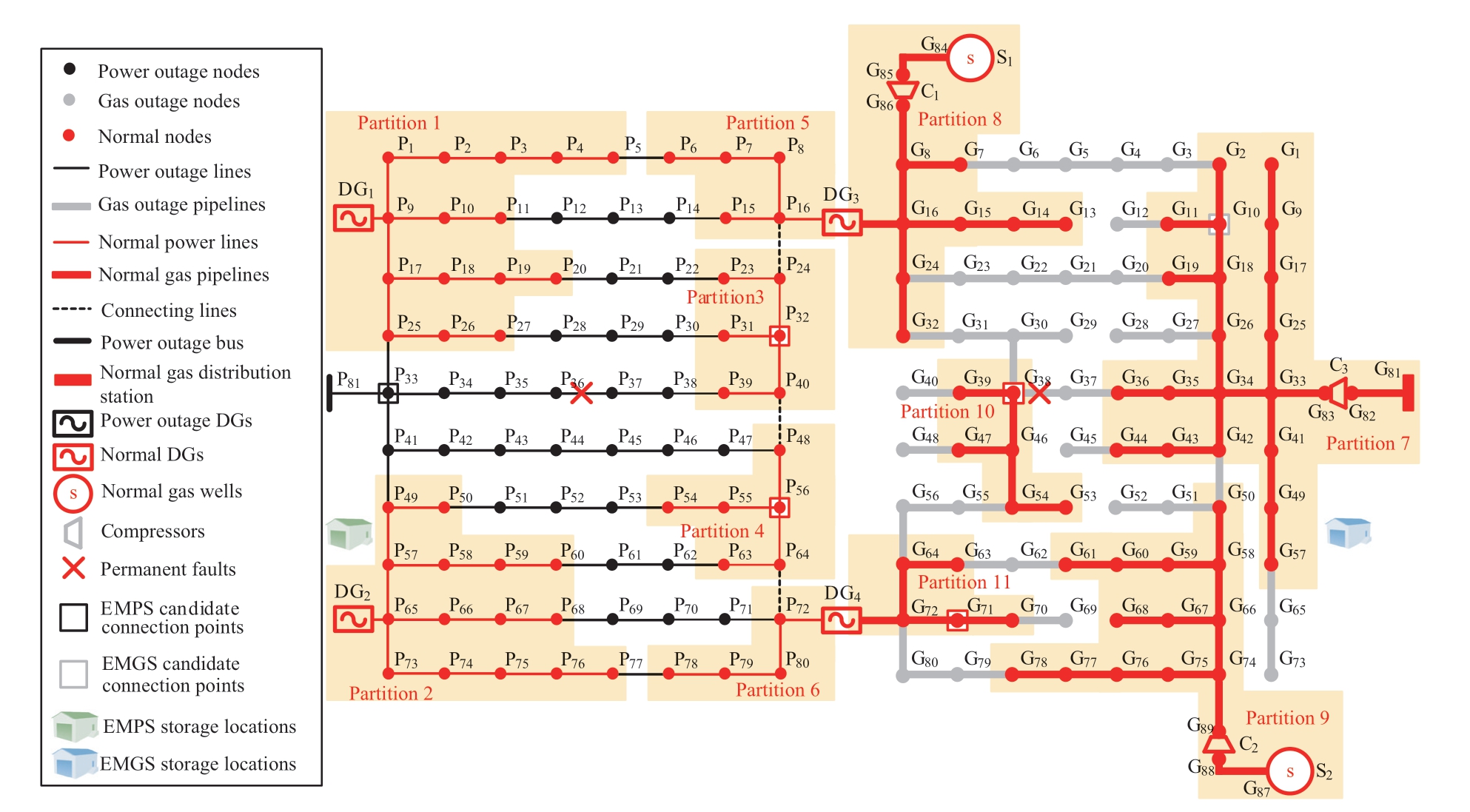
Fig.B5 Restored system topology structure of MG2PIES
Table B5 Optimal restoration schemes
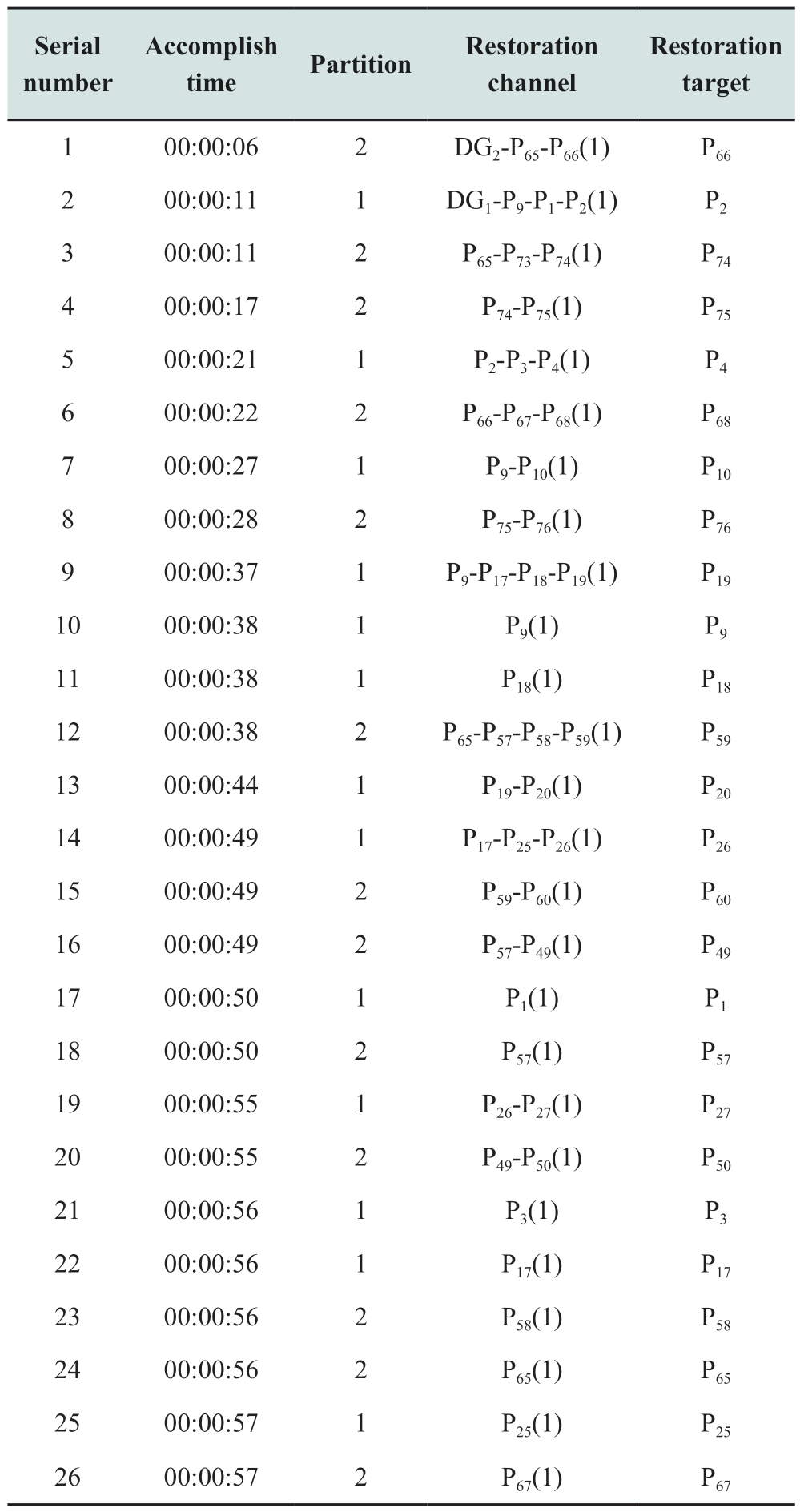
continue
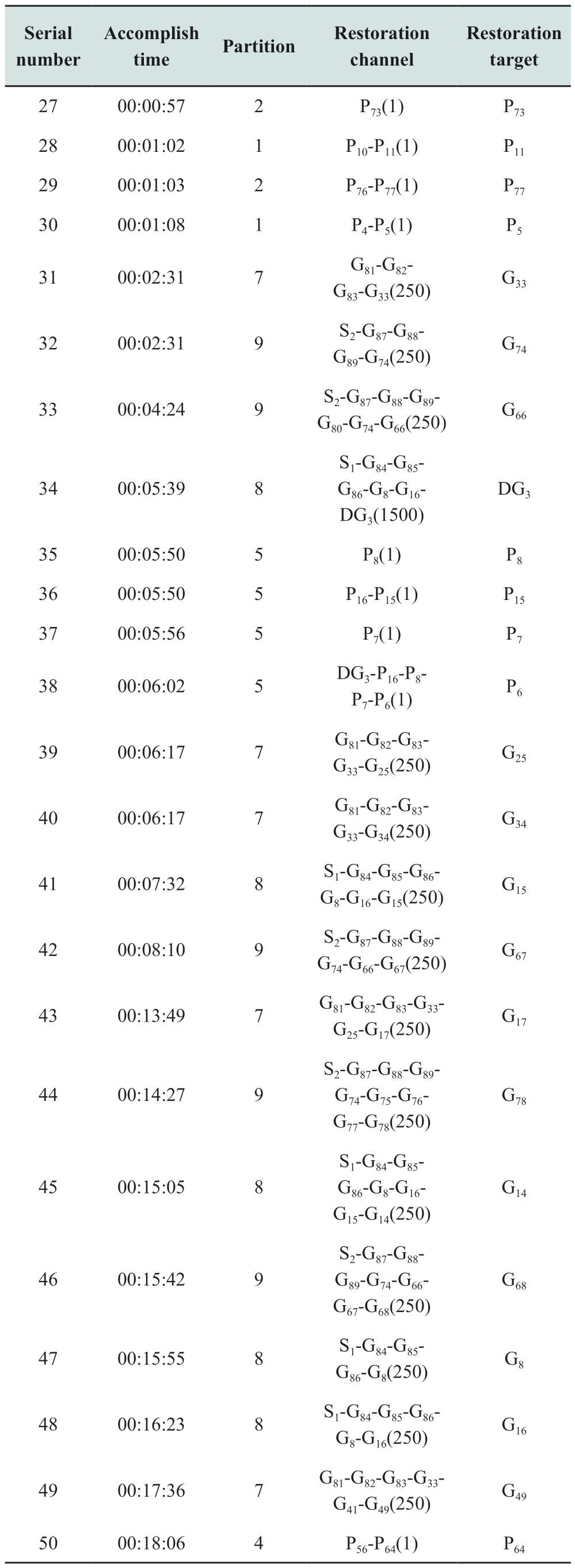
continue
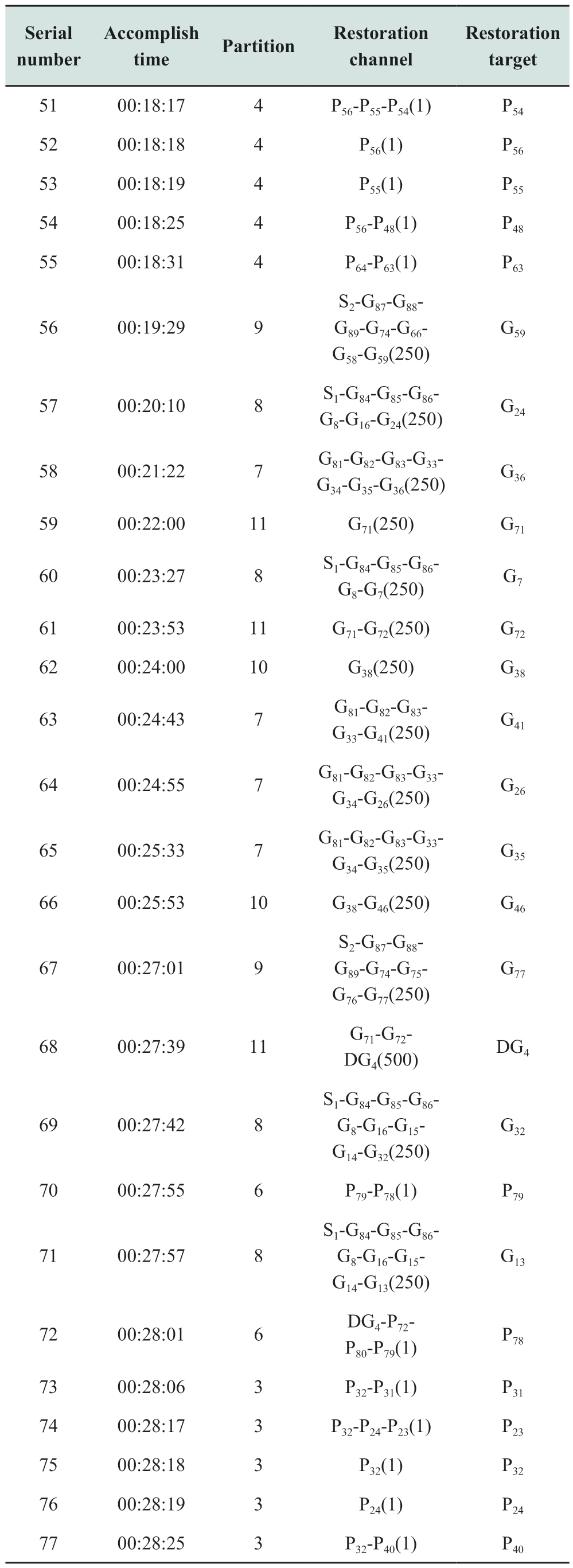
continue
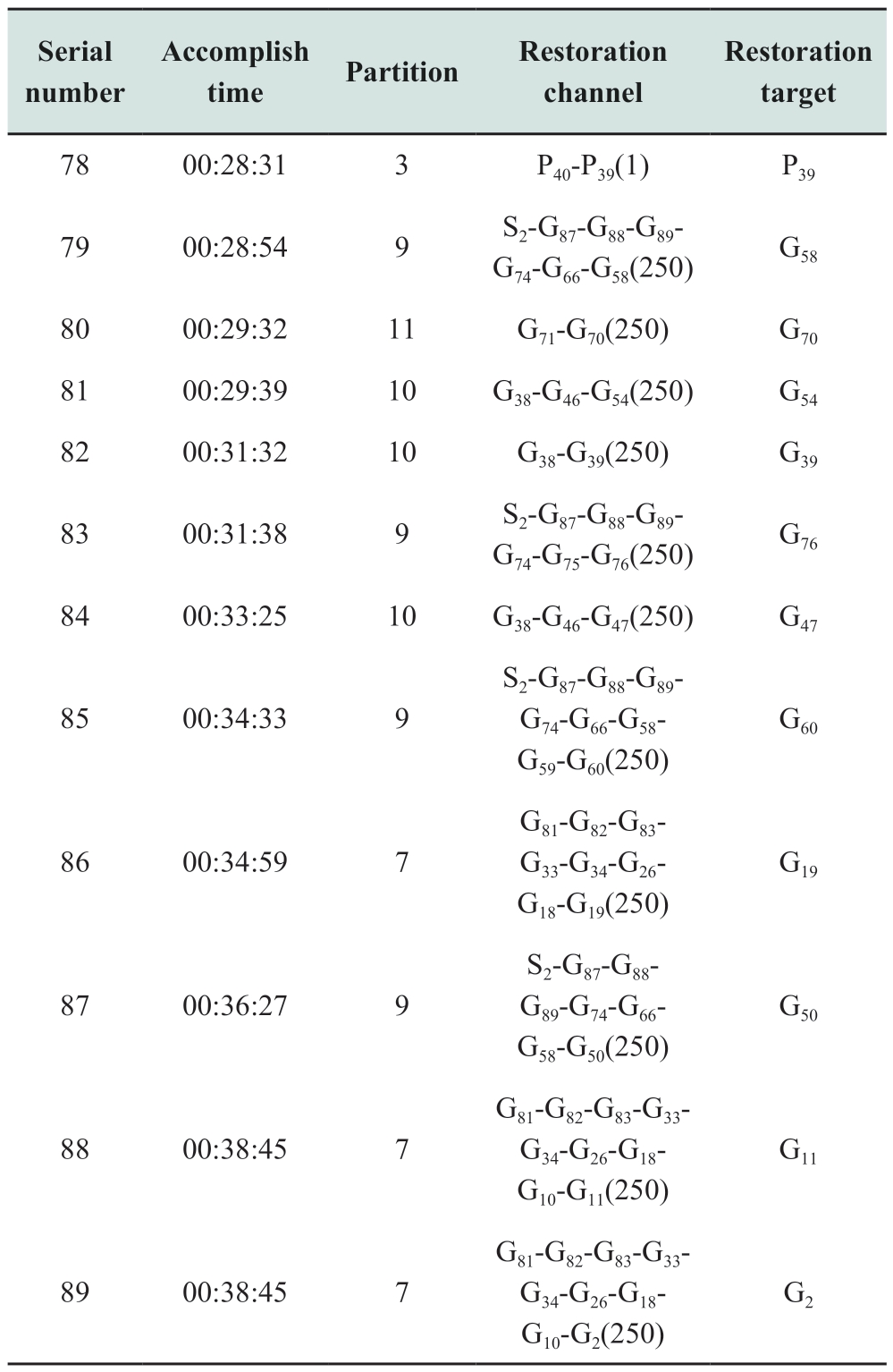
continue
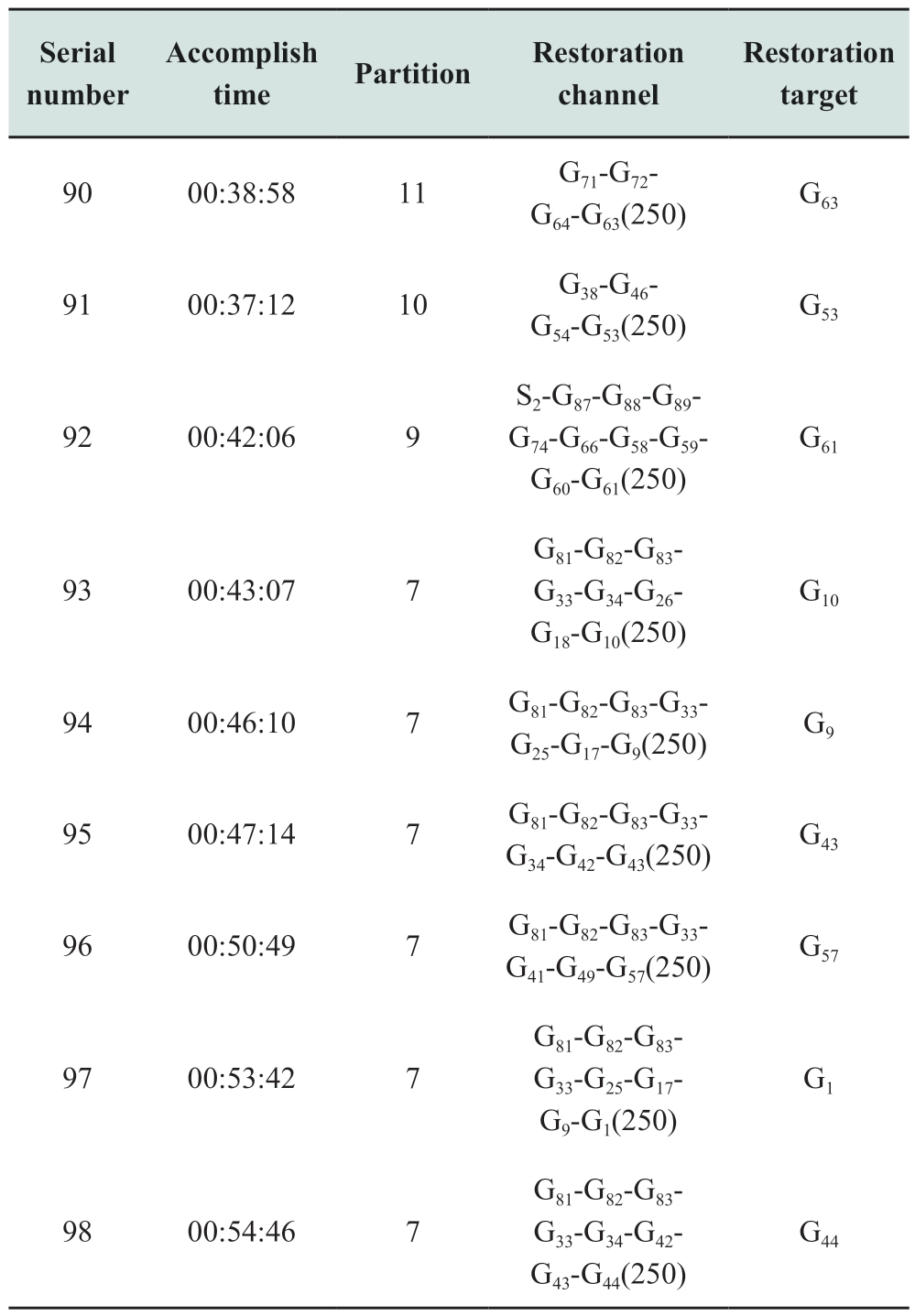
(Note: The power (gas flow) consumed by the corresponding nodes in this step scheme is in brackets,in MW(m3/h).)
4.3 Scenario contraction
With the initial energy shutdown conditions described in Section 4.1,three scenarios were considered to verify the effectiveness of the coordinated restoration optimization proposed in this study.
Scenario 1: Considering MEPSs and MEGSs participating in energy supply restoration and the G2P flow optimization decision,the restoration optimization decision is made completely according to the proposed method.
Scenario 2: Based on Scenario 1,except MEPSs and MEGSs are not considered for energy supply restoration.
Scenario 3: Based on Scenario 1,except the G2P flow optimization decision is not considered,meaning that the gas supply for DG3 and DG4 from the GDP is not permitted.
The restoration cost and income of the MG2PIES in each scenario are shown in Figs.9 and 10,respectively.
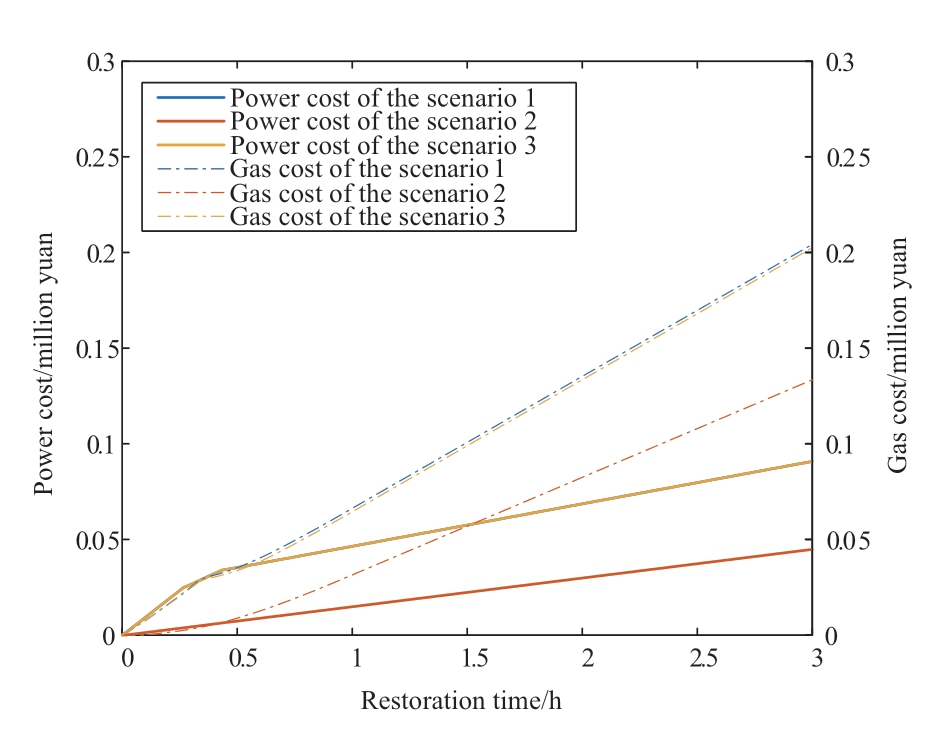
Fig.9 Restoration cost comparison for different scenarios
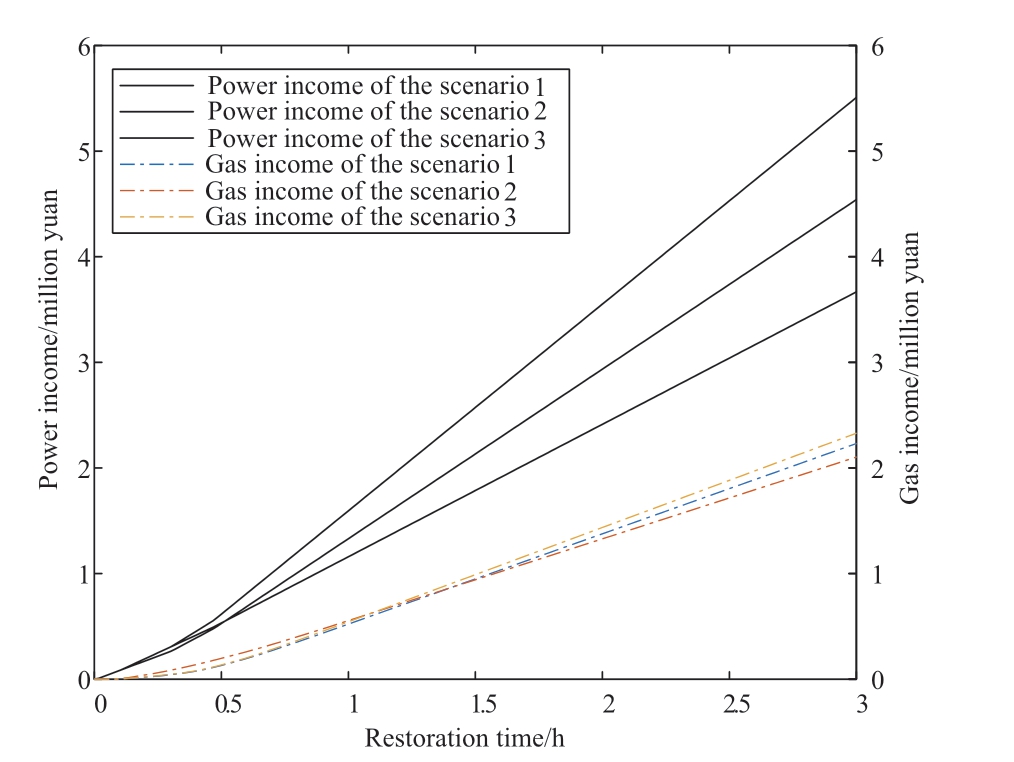
Fig.10 Comparison of restoration income in different scenarios
The restoration cost includes the energy supply and transportation costs of mobile emergency sources.Scenario 2 included fixed power and gas sources.Due to the fast transmission speed of power,it takes only a few minutes to complete distribution of available power from power sources in a power distribution system; thus,its cost increases evenly.The transmission speed of natural gas is slow; tens or dozens of minutes are required to complete distribution of the available flow of gas sources in the gas distribution system.The growth rate of the gas cost increases with an increase in gas flow supply from gas sources until distribution of available gas flow from gas sources is completed.Thus,the restoration cost curve in Scenario 2 increases slowly at first,and then rapidly.In Scenarios 1 and 3,MEPSs and MEGSs were added to participate in restoration.A high transportation cost was considered before the MEPSs and MEGSs were connected and a low energy supply cost was considered after they were connected.Thus,the restoration cost curve for Scenarios 1 and 3 first increased and then slowed.After the MEPSs and MEGSs were connected,the cost curve was similar to that for Scenario 2.
Restoration income is the income derived from the advanced energy supply to the load to be restored.Scenario 2 included fixed power and air sources.Before allocation of available capacity was completed,the restored income increased rapidly from zero with an increase in available capacity.After allocation of available capacity was completed,no additional load was restored,and the uniform growth in restoration income reflected the income accumulation of the early restored load over time.
In Scenarios 1 and 3,MEPSs and MEGSs were added to participate in restoration.The only difference between Scenarios 3 and 2 is that there is no income before the MEPSs and MEGSs are connected,and the curves after they are connected are similar to those of fixed power and gas sources,respectively.In Scenario 1,owing to the G2P gas flow optimization,part of the gas flow is converted into power energy to supply power to the power load to be restored.Thus,the restoration income of the power load increases rapidly,and the corresponding restoration income of the gas load increases to a lesser extent,with the net income of the entire system being higher.
The total restoration net income and restored load of the MG2PIES in each scenario are shown in Figs.11 and 12,respectively.
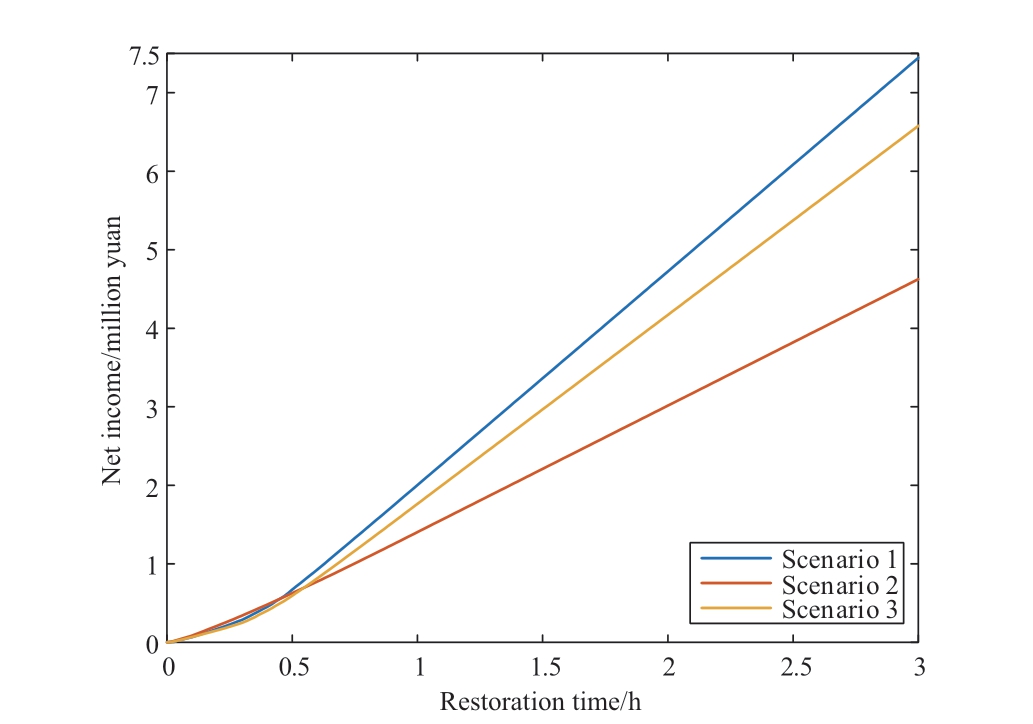
Fig.11 Net income comparison of different scenarios
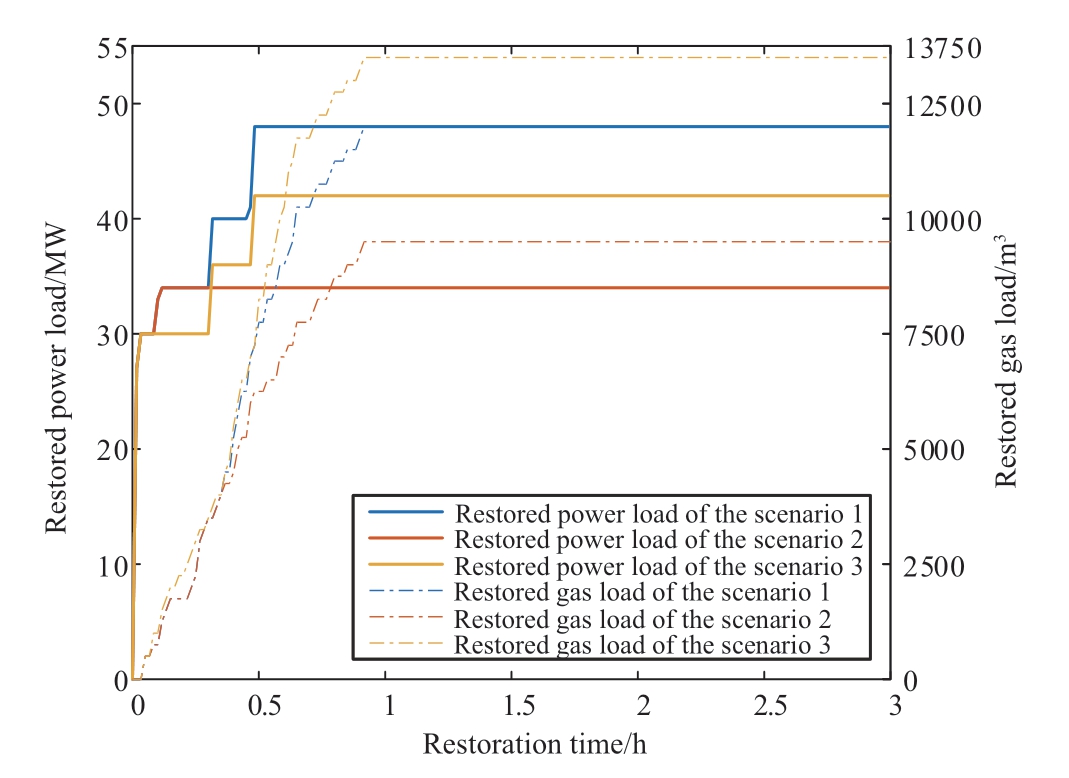
Fig.12 Load restoration comparison of different scenarios
In Fig.11: (1) participation of the MEPS and MEGS in restoration increased the available capacity of the system and increased the number of parallel partitions to improve the opportunity for the load to be restored to obtain the available capacity and reduce the amount of time needed.Thus,at 03:00:00,the net income of Scenario 1 increased by 37.8% compared with that of Scenario 2.(2) Due to the transportation costs of the MEPS and MEGS,the net income of Scenario 2,without considering mobile emergency sources before 00:28:00,was higher than that of the other two scenarios.However,in the long-term,the net income of Scenario 2 is much lower than that of the other two scenarios after 00:28:00 because there is no support for the available capacity of mobile emergency sources.(3) The total available capacity in Scenario 1 is equal to that in Scenario 3.However,because Scenario 1 has an optimized G2P flow rate (optimal distribution of gas flow in the PDS and GDS for maximum restoration income at 03:00:00),the net income of Scenario 1 increased by 11.6% compared with Scenario 3.Because it takes time to supply gas to the GFG,Scenarios 1 and 3 have the same net income until 00:05:39.
In Fig.12: (1) In the first 20 min,the power and gas load restoration in Scenarios 1 and 2 was the same.Later,with the involvement of the MEPS and MEGS in Scenario 1,the total power and gas load restoration in Scenario 1 was greater than that in Scenario 2.(2) The power load restoration in Scenario 2 was completed before 00:06:02,and in Scenarios 1 and 3 was completed before 00:28:31.The restoration was greater than that in Scenario 2.Although energy supply restoration involving mobile emergency sources improved the load restoration,the restoration speed was slow due to the time spent in transportation.(3) In scenarios 1,2,and 3,gas loads were restored at 00:54:46.Because the speed of the gas flow was of a similar order of magnitude to the speed of mobile emergency source transportation,the impact of the transportation of mobile emergency sources was not determined.The gas load restoration time in each scenario was subjected to the restoration process of a largecapacity gas distribution station.(4) In each scenario,the restoration speed of the power load was significantly higher than that of the gas load.Newly available power from power sources can be fully allocated to the load within a few minutes,whereas the available flow of gas sources can be fully allocated in ten or dozens of minutes,reflecting the time scale difference between the power and gas supply.(5) The total available capacity in Scenario 1 is the same as that in Scenario 3.The G2P flow optimization in Scenario 1 allocates gas flow to more important power loads,resulting in a higher global income; thus,compared with Scenario 3,less gas load is restored in Scenario 1,and more power load is restored.
The results indicate that,considering the scheduling optimization decision of the mobile emergency source proposed in this study,more critical loads can be restored,improving the net income of the overall restoration scheme of the MG2PIES.
5 Conclusion
In this study,the composition of the MG2PIES and the coupling characteristics of the PDS and GDS was investigated.On this basis,a coordinated energy supply restoration optimization model of an MG2PIES with mobile emergency sources was established with the goal of maximizing net income,and an optimization decisionmaking framework and method were proposed.The coordinated restoration optimization decision of the MG2PIES energy supply restoration includes system status recognition,mobile emergency source dispatching optimization,G2P gas flow optimization,and parallel intra-partition restoration scheme optimization of the PDS and GDS.The examples demonstrate that the decisionmaking method in this study can fully coordinate the energy supply sources and the loads to be restored by the GDS and PDS,and significantly improve the net income of system restoration,utilization rate of energy supply sources,and load restoration rate.
Appendix A
Transient model analysis of gas pipeline
Assuming that the gas transportation process in a pipeline is a constant temperature process,the transient model of the gas pipeline can be represented in the form of a partial differential equation.
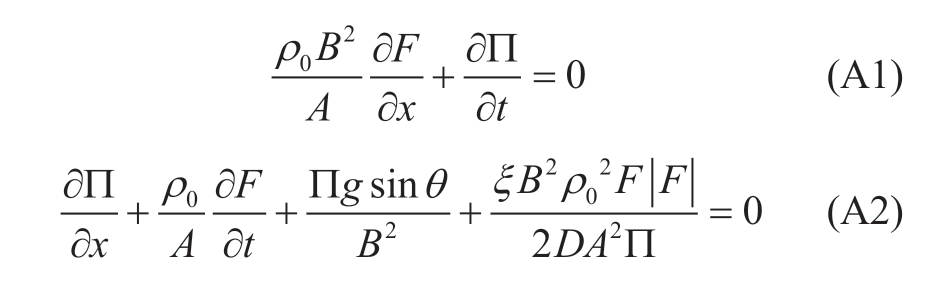
where ρ0 is the density of gas in the standard state,B is the gas wave velocity,A is the pipeline cross-sectional area,F is the gas flow rate,which has positive and negative values.This study considers F to be positive when the gas enters the node and considers F to be negative when the gas leaves the node.x is the pipe space distance,Π is the gas pressure,t is the time,g is the acceleration due to gravity,θ is the inclination angle between the pipeline and horizontal plane,ξ is the hydraulic friction coefficient,D is the inner diameter of the pipeline.
In this study,the characteristic line method was used to approximate the partial differential equation.The x-t grid of the characteristic line method is shown in Fig.A1,where ∆x is the space step,∆t is the time step,and ∆x = B∆ t.
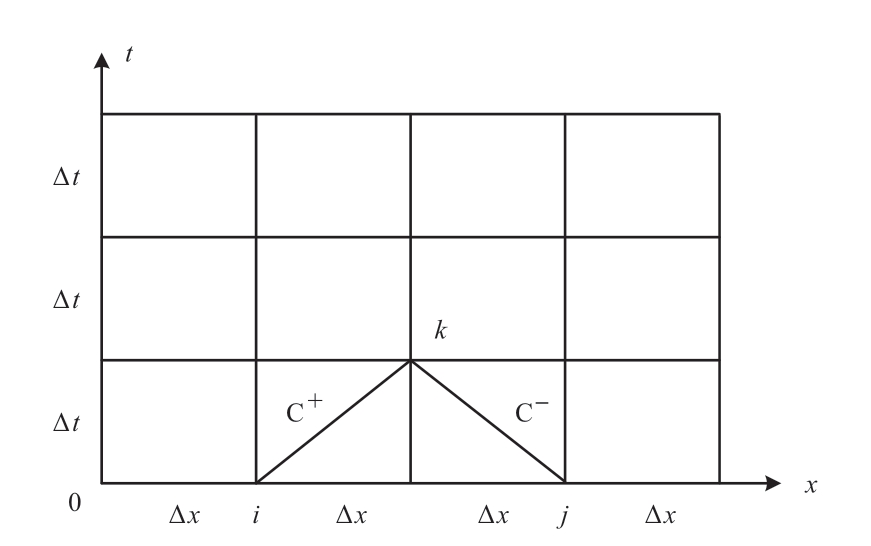
Fig.A1 x-t grid of characteristic line method
From (A1) and (A2),the equation of the characteristic line in an ordinary differential form can be obtained as
In the direction of C+,immediately:

In the direction of C−,immediately:

Integrating (A3) and (A4) along the characteristic line yields
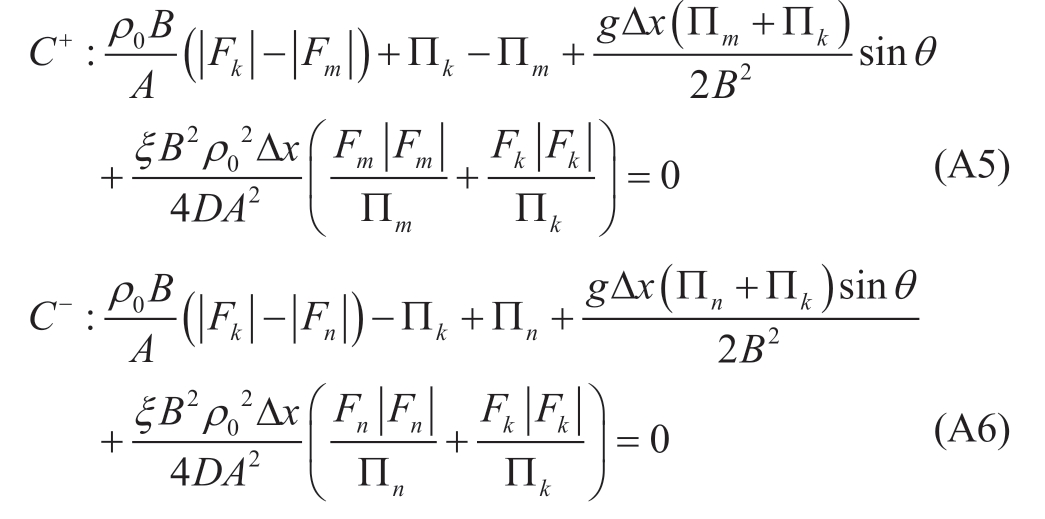
Given the pressure and flow rate of nodes m and n of gas,the pressure and flow rate at node k can be obtained by combining Equations (A5) and (A6).
Appendix B
Acknowledgements
The work was supported by the Open Research Fund of Jiangsu Collaborative Innovation Center for Smart Distribution Network (XTCX202001) and National Natural Science Foundation of China (52077061).
Declaration of Competing Interest
We declare that we have no conflict of interest.
References
[1] Liu Y B,Su Y C,Xiang Y,et al.(2019) Operational reliability assessment for gas-electric integrated distribution feeders.IEEE Transactions on Smart Grid,10(1): 1091-1100
[2] Huang W J,Du E S,Capuder T,et al.(2021) Available transfer capability calculation of integrated electricity and natural-gas systems considering delay characteristics of gas transmission system.IEEE Transactions on Power Systems,36(5): 3948-3959
[3] Xie H P,Sun X T,Chen C,et al.(2022) Resilience metrics for integrated power and natural gas systems.IEEE Transactions on Smart Grid,13(3): 2483-2486
[4] Ma R,Wang D S (2019) Static security coupling analysis of multi-energy flow system considering natural gas system N-1 contingency.Proceedings of the CSEE,39(6): 1627-1636 (in Chinese)
[5] Yan C,Bie Z,Wang C,et al.(2019) Risk assessment studies for new generation energy system.Power System Technology,43(1):12-22 (in Chinese)
[6] Zhang G L,Zhong H W,Tan Z F,et al.(2021) Texas electric power crisis of 2021 warns of a new blackout mechanism.CSEE Journal of Power and Energy Systems,8(1): 1-9
[7] Shao C C,Shahidehpour M,Wang X F,et al.(2017) Integrated planning of electricity and natural gas transportation systems for enhancing the power grid resilience.IEEE Transactions on Power Systems,32(6): 4418-4429
[8] Li J X,Yin X,Wang Y,et al.(2021) Resilience-motivated distribution system restoration considering electricity-water-gas interdependency.IEEE Transactions on Smart Grid,12(6): 4799-4812
[9] Yang L J,Wang C,Zhou Y,et al (2020) Electric-gas coupled integrated energy fault recovery strategy based on bi-level optimization model.Power System Technology,44(11): 4264-4273 (in Chinese)
[10] Yun J Y,Yan Z,Zhou Y,et al.(2020) Multi-time collaborative restoration for integrated electrical-gas distribution system based on rolling optimization.CSEE Journal of Power and Energy Systems: 1-13
[11] Yu J,Ma M N,Guo L,et al.(2018) Reliability evaluation of integrated electrical and natural-gas system with power-to-gas.Proceedings of the CSEE,38(3): 708-715 (in Chinese)
[12] Li G Q,Yan K F,Zhang R F,et al.(2022) Resilience-oriented distributed load restoration method for integrated power distribution and natural gas systems.IEEE Transactions on Sustainable Energy,13(1): 341-352
[13] Chen W,Ding X,Shi Y H,et al.(2019) Sequential fault restoration method for electricity-gas integrated energy system considering two-way coupling.Electric Power Automation Equipment,39(8): 86-94 (in Chinese)
[14] Liu W J,Sun L,Lin Z Z,et al.(2016) Multi-objective restoration optimisation of power systems with battery energy storage systems.IET Generation,Transmission & Distribution,10(7):1749-1757
[15] Wang Y H,Liu W X,Yao Q,et al.(2022) Pre-layout and dynamic scheduling strategy of mobile energy storage for resilience enhancement of distribution network.Automation of Electric Power Systems,46(15): 37-45 (in Chinese)
[16] Lei S B,Chen C,Zhou H,et al.(2019) Routing and scheduling of mobile power sources for distribution system resilience enhancement.IEEE Transactions on Smart Grid,10(5): 5650-5662
[17] Xu Y,Wang Y,He J H,et al.(2020) Optimal decision-making method for multi-period load restoration in distribution network with coordination of multiple sources.Automation of Electric Power Systems,44(2): 123-131 (in Chinese)
[18] Xu N Z,Chan K W,Chung C Y,et al.(2020) Enhancing adequacy of isolated systems with electric vehicle-based emergency strategy.IEEE Transactions on Intelligent Transportation Systems,21(8): 3469-3475
[19] Li B D,Chen Y,Wei W,et al.(2022) Online coordination of LNG tube trailer dispatch and resilience restoration of integrated power-gas distribution systems.IEEE Transactions on Smart Grid,13(3): 1938-1951
[20] Habibi M,Vahidinasab V,Mohammadi-Ivatloo B,et al.(2022)Exploring potential gains of mobile sector-coupling energy systems in heavily constrained networks.IEEE Transactions on Sustainable Energy,13(4): 2092-2105
[21] Liu W J,Wen F S,Xue Y S,et al.(2016) Cost characteristics and economic analysis of power-to-gas technology.Automation of Electric Power Systems,40(24): 1-11 (in Chinese)
[22] Fotouhi H,Moryadee S,Miller-Hooks E,et al.(2017)Quantifying the resilience of an urban traffic-electric power coupled system.Reliability Engineering & System Safety,163:79-94
[23] Wang W L,Wang D,Jia H J,et al.(2017) Steady state analysis of electricity-gas regional integrated energy system with consideration of NGS network status.Proceedings of the CSEE,37(5): 1293-1304 (in Chinese)
[24] Chen M,Bai L P,Fu P (2005) Discussion on emergency gas sources instead of natural gas.Gas and Heat,25(12): 21-23 (in Chinese)
[25] Zhang R F,Li X,Jiang T,et al.(2021) Review on resilience assessment and enhancement of urban integrated energy system.Journal of Global Energy Interconnection,4(2): 122-132 (in Chinese)
[26] Chen H H,Shao J Y,Jiang T,et al.(2019) Static N-1 security analysis for integrated energy system based on decoupled multienergy flow calculation method.Automation of Electric Power Systems,43(17): 20-27,131 (in Chinese)
[27] Zhang P,Tang P,Ding Y,et al.(2018) Service restoration strategy considering the volatility of distribution generations for active distribution network.Proceedings of the CSU-EPSA,30(1): 115-120 (in Chinese)
[28] Jia B Y,Li D,Li X Y,et al.(2022) Two-stage restoration strategy for multi fault of an electric gas integrated energy system.Power System Protection and Control,50(9): 113-123 (in Chinese)
[29] Zhou T,Hao L L,Wang H H,et al.(2018) Unscheduled outage restoration strategy of active distribution network considering risk and gain.Automation of Electric Power Systems,42(13): 136-144 (in Chinese)
[30] Wang C,Hao L L,Hu W Y,et al.(2022) Collaborative optimization decision-making of energy supply and restoration in power-gas integrated energy system.Power System Technology,46(8): 3017-3027 (in Chinese)
[31] Hao L L,Xue Y S,Li Z,et al.(2022) Decision support system for adaptive restoration control of distribution system.Journal of Modern Power Systems and Clean Energy,10(5): 1256-1273
Full-length article
Received: 10 December 2022/ Accepted: 28 February 2023/ Published:25 April 2023
Wangyu Hu
hwy41498@163.com
Lili Hao
haolili@njtech.edu.cn
Chuan Wang
1275552944@qq.com
Guangzong Wang
wangguangzong@bohai-power.com
Yue Sun
1751870159@qq.com
Jun Chen
13851941724@163.com
Xueping Pan
xueping_pan@163.com
2096-5117/© 2023 Global Energy Interconnection Development and Cooperation Organization.Production and hosting by Elsevier B.V.on behalf of KeAi Communications Co.,Ltd.This is an open access article under the CC BY-NC-ND license (http: //creativecommons.org/licenses/by-nc-nd/4.0/).
Biographies
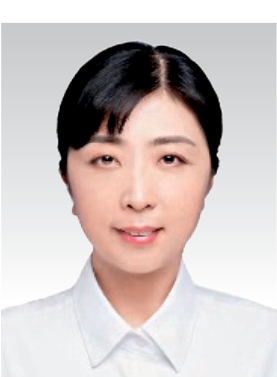
Lili Hao received the B.S.and M.S.degrees from Hohai University,Nanjing,China,in 2001 and 2004,respectively,and the Ph.D.degree from Southeast University,Nanjing,China,in 2010.She is working in College of Electrical Engineering and Control Science,Nanjing Tech University,Nanjing,China.Her research interest is power system security and stability analysis and control.
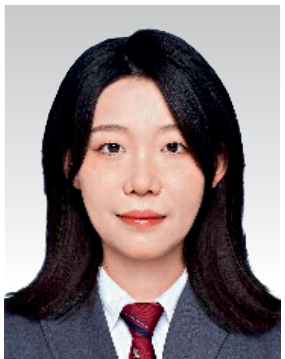
Wangyu Hu received the B.S.degree at Yanshan University,Qinhuangdao,China,in 2020.She is working towards the M.S.degree at Nanjing tech University,Nanjing,China.Her research interest is power system security and stability analysis and control.
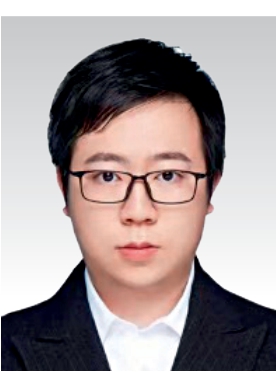
Chuan Wang received the B.S.and M.S.degrees from Nanjing tech University,Nanjing,China,in 2019 and 2022,respectively.He is working in Shennan Circuits Co.,Ltd,Wu Xi,China.His research interest is power system security and stability analysis and control.
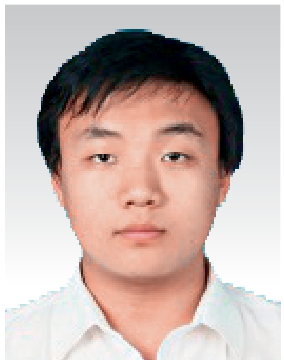
Guangzong Wang received the Master’s degree at the School of Electrical and Information Engineering,Tianjin University,2013.His research interests include Integrated Intelligent Energy,Virtual Power Plant,energy internet,etc.
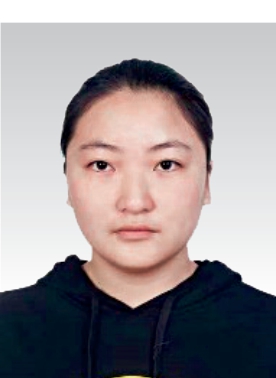
Yue Sun received the B.S.degree at Taizhou Institute of Technology,Nanjing University of Science and Technology,Taizhou,China,in 2020.She is working towards the M.S.degree at Nanjing tech University,Nanjing,China.Her research interest is power system security and stability analysis and control.
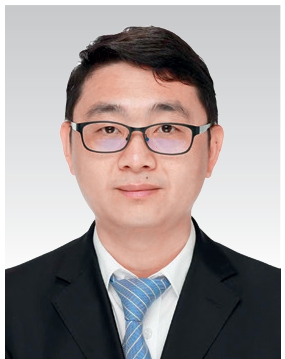
Jun Chen received the B.S.M.S.,and Ph.D.degrees in 2000,2003,and 2022,respectively.He is working in College of Electrical Engineering and Control Science,Nanjing Tech University,Nanjing,China.His research interest is power system security and stability analysis and control.
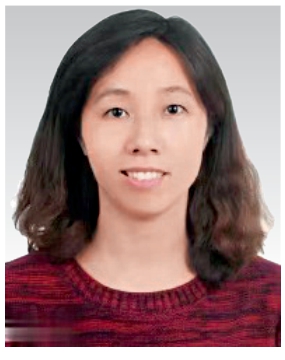
Xueping Pan received the B.S.Eng.and M.S.degrees in electrical engineering from Hohai University,China,in 1994 and 2000,respectively,and the Ph.D.degree from Zhejiang University,China,in 2008.She is working in College of Energy and Electrical Engineering,Hohai University,Nanjing,China.Her research interest is modeling and analysis of power systems with renewable power generation.
(Editor Dawei Wang)