0 Introduction
In recent years,the scale of electricity demand has been expanding in China under the stimulation of its rapid economic development.Consequently,it is vital to solve the problems of fossil energy scarcity and environmental pollution caused by the traditional thermal power industry.In this context,China announced the “30·60” goal of carbon peaking and carbon neutrality[1].The development of renewable energy is regarded as a crucial strategy for energy conservation and carbon emission reduction.However,on the one hand,the fluctuation and intermittent nature of the output of renewable energy power generation require power systems with higher flexibility[2].Additionally,the incompatibility between the output of renewable energy generation and load demand induces serious wind and solar power abandonment[3,4].On the other hand,during the early stage of renewable energy development,the Chinese government implemented policies,such as feed-in tariffs(FIT)[5],to provide subsidies.However,the subsidy gap has continued to expand with the maturing of renewable energy technology,and the government is facing substantial financial pressure[6].Therefore,there is an urgent need for China to establish an effective joint trading mechanism of green certificate,carbon emission right and electricity to realize a green certificate- carbon emission right- electricity multi-market,which would alleviate the financial pressure on the government and promote market competitiveness among renewable energy suppliers.
In the process of bidding among power suppliers,game theory becomes an effective tool to meet the strategic optimization requirements of multiple decision makers.However,the classical game theory is based on game subjects with perfect rationality,and the game process develops from the perspective of maximizing self-interest.However,in a real market,most participants cannot satisfy the requirement of “perfect rationality”.The emergence of evolutionary game theory provides a feasible solution to overcome the shortcomings of traditional game theory.The evolutionary game theory advocates that the game subjects only have bounded rationality,and each subject achieves equilibrium by constantly revising their actions and strategies throughout the game process[7-9].Cheng et al.[10]investigated the homogeneous and heterogeneous evolutionary game decision behavior of long-term bidding in the power generation market.Yin et al.[11]studied multiple evolution game strategies of four groups and more multiple groups in the open electricity market.Zhao et al.[12]developed an evolution game model of power producers to analyze the symbiotic evolution between the renewable portfolio standard(RPS)and the behavior strategies of electricity producers.Peng et al.[13]introduced an evolutionary game to solve the optimal bidding strategy in the uncertainty scenario of a smart grid containing a high proportion of new energy accesses.The above research verifies the feasibility of evolutionary game theory in solving realistic boundary rationality problems,and enriches the application scenarios of evolutionary game models in the power market.Therefore,in this paper,we construct a green certificate- carbon emission right- electricity joint market based on evolutionary game theory.
The research priorities of global renewable energy subsidy policies[14]include feed-in tariff(FIT),Green Certificates Trading(GCT)mechanism under the RPS[15,16],and carbon emissions trading(CET)mechanism[17].Since 2021,China has implemented the GCT mechanism,allowing renewable energy power suppliers to obtain extra income through GCT[18-21].By contrast,China’s carbon market was launched in 2011 in seven pioneer cities,including Beijing,Tianjin,and Shenzhen,to develop toward the official launch of the national unified market on July 16,2021[22-24].The parallel implementation of GCT and CET systems introduces an effective market-based means for reducing the new energy subsidy gap.Tan et al.[25]used a time series analysis method called the autoregressivegeneralized autoregressive conditional heteroskedasticity model(AR-GARCH),to predict the change of carbon emission rights price,based on which an optimal dispatch model of a wind-light-fire power system was constructed to consider the dynamic carbon emission cost.Luo et al.[26]proposed an optimal operation model of integrated energy systems by combining the GCT and CET mechanisms.Sun et al.[27]developed a two-layer optimization model of a generation-side price noncooperative game for considering green certificates and carbon emission costs.
However,most existing studies consider green certificates and carbon emission rights as fixed-price commodities,and ignore their dynamic trading process.Moreover,the construction of China’s GCT and CET market is still at an initial stage,which makes it necessary to continue improving the quota allocation management and market supervision systems.In doing so,the trading varieties and trading methods can be gradually developed under the premise of strengthening risk management.Therefore,a green certificate- carbon emission rightelectricity joint market is designed in this study,during which the Rubinstein game theory and ladder pricing method are used to reflect the GCT and CET bilateral transaction process.Furthermore,the impact of the revolutionary game-based optimization model on GCT and CET mechanisms is further investigated.
The main contributions of this paper are as follows:
1)A dynamic green certificate trading cost model based on Rubinstein game and carbon emissions cost model based on ladder pricing method are developed by introducing a trading process of green certificate- carbon emission rightelectricity multi-level market.The proposed market can effectively promote the participation of power suppliers in GCT and CET trading,thereby increasing extra profits from renewable power units and alleviating the pressure of government subsidies.
2)A bidding optimization model of green certificatecarbon emission right- electricity multi-level market based on evolutionary game theory is proposed.Mathematical analysis and simulation results demonstrate the dynamic process underlying the bidding strategy of a generator and verify that the proposed multi-market can give priority to the consumption of renewable energy.
The remainder of this paper is structured as follows.Section 1 introduces the joint trading process of the green certificate- carbon emission right- electricity multimarket.Section 2 establishes the dynamic cost models of GCT,CET,and electricity.Section 3 describes the bidding optimization model of the multi-market based on evolutionary game theory.Section 4 presents the case study,and Section 5 discusses the conclusions of this study.
1 Green certificate- carbon emission rightelectricity multi-market joint trading process
1.1 Green certificate trading(GCT)system
Generally,the GCT system is implemented collectively with the RPS mechanism.In other words,the government will impose mandatory requirements on the proportion of renewable energy electricity consumed by enterprises.RPS varies provincially,and is primarily based on the diversity of natural resources and level of economic development.The RPS subjects that undertake the renewable energy power consumption responsibility include electricity retailers,power users participating in the wholesale market,and enterprises with privately owned power plants.Power producers can obtain corresponding green certificates by producing renewable energy,and the additional green certificates can be sold for profit.GCT reflects the environmental value of renewable energy in addition to the value of electricity.
1.2 Carbon emissions trading(CET)system
CET system is a market in which carbon emission rights are publicly traded as valuable commodities.Based on the benchmarking method,the initial carbon emission rights are allocated free of charge.Generally,the benchmark value is determined based on the historical carbon emission intensity level,emission reduction potential of the enterprises,industrial policies,and energy consumption targets[28].Enterprises with insufficient trading rights will not be able to participate in profitable production activities,while enterprises with surplus trading rights can sell carbon emission trading rights to gain profits.
1.3 Multi-market joint trading process
In this paper,a green certificate- carbon emission right- electricity multi-market joint trading mechanism is proposed.This trading system comprises three parts,and their main functions are as follows:1)Operation and management center:Allocates initial carbon emission rights and green certificate quotas,checks qualification of power,CET,and GCT suppliers,and monitors quota completion.2)Power suppliers:three power generation resources are selected in this study,including thermal power,wind power,and photovoltaic(PV)power.3)Electricity retailer:facilitates the connection between users and power suppliers,and undertakes the renewable energy quota.The bilateral interaction can mobilize the trading enthusiasm of market players and restrain market power,thus achieving higher market efficiency than fixed-price trading.Figure 1 illustrates the trading process of the proposed joint market.
The trading steps of the joint market are as follows:
1)The operation and management center announces the load forecast data one trading day in advance.
2)Each power supplier submits their bidding price and electricity quantity one trading day in advance.Generally,the declaration electricity quantity is the highest generation capacity of the supplier to maximize profits.
3)The electricity retailer will rank all the bidding prices from lowest to highest,acquire the clearing results,and announce the marginal clearing price.
4)Based on the clearing results,the excess or shortage of GCT and CET of each subject will be calculated according to the initial quota.Subsequently,CET are either rewarded or penalized according to the ladder pricing model.Meanwhile,the transaction price in the GCT market is negotiated between renewable energy suppliers and electricity retailers.The quoted price must comply with the upper and lower limit constraints provided by the government to limit price fluctuation.
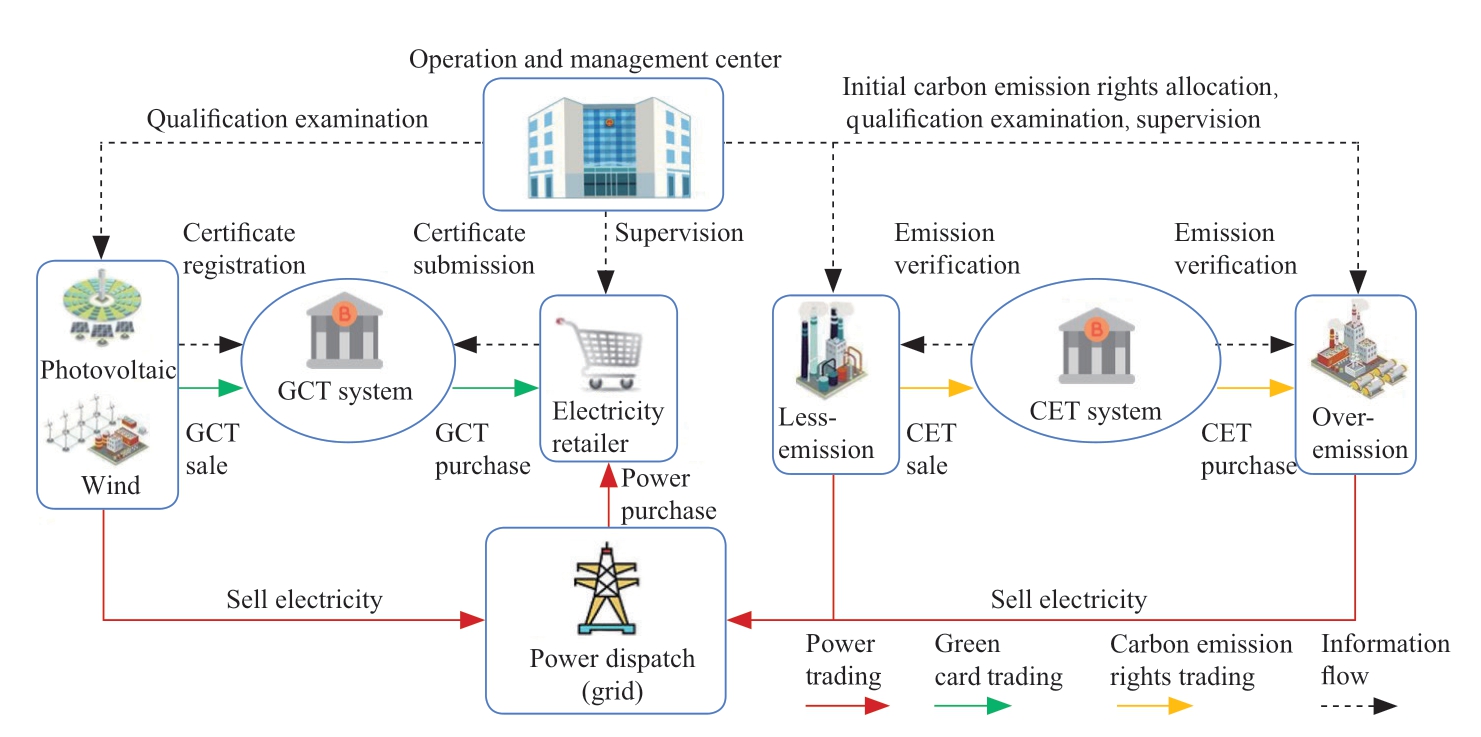
Fig.1 Green certificate- carbon emission rights- electricity multi-market trading process
5)Each supplier calculates their total profits.If a supplier needs to change the bidding strategy,return to step 2).The trading ends when all suppliers refrain from changing their bidding information.
2 Green certificate- carbon emission rightelectricity cost model
2.1 GCT cost model
In this study,the GCT market is established from a dynamic perspective,and a sequential Rubinstein game model with incomplete information[29]is constructed,which is used to analyze and describe the negotiation process between renewable energy suppliers and electricity retailers about the price of GCT.Figure 2 depicts the negotiation process.
It is assumed that the renewable energy supplier and electricity retailer are game participants A and B.To avoid an indefinite increase in the number of negotiation rounds,both sides of the game have fixed costs to spend in each round of negotiation.Both sides intend to reach an agreement as early as possible.The impatience and risk aversion of the participants are crucial factors affecting the negotiation results,which are measured using a discount factor.The discount factors of the game participants A and B are defined as δ1 and δ2,respectively,where 0 <δ1 <1<δ2 <2.The trading quantity of GCT is defined as qGCT.indicate the bid price of participants A and B at roundn,respectively.In this study,the owner of GCT is considered to be dominant in the transaction and has bidding priority.The specific negotiation process is as follows:
1)Player A bids first with a bid price of.
2)If player B accepts the bid price ,the game ends.Accordingly,the GCT profits and costs are
If the bid price is rejected,player B will offer a new bid price
.
3)Similarly,if A accepts the bid price,then the game terminates,and the benefits and costs for both sides are
If the bid price is rejected,player A will re-offer a new bid price
.Following n rounds,both sides are satisfied with a final deal price
,and the GCT transaction ends.
At the end of the negotiation,the revenue of the renewable energy supplier and the cost of the electricity retailer are:
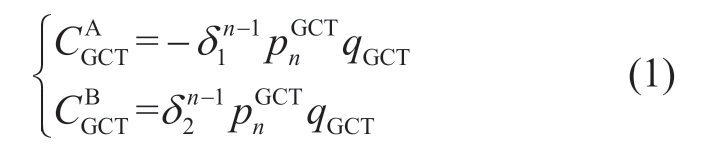
where represents the profit of renewable energy suppliers,which is obtained from selling GCT.
represents the cost of purchasing GCT for power retailers.
represents the final deal price of GCT after n rounds of negotiation.qGCT represents the final deal quantity of GCT.
2.2 CET cost model
In this paper,the baseline method is adopted to determine carbon emission allowances,and the government issues the initial allowances free of charge.Generally,the amount of CO2 emitted during the generation of thermal power units is expressed using the following equation:

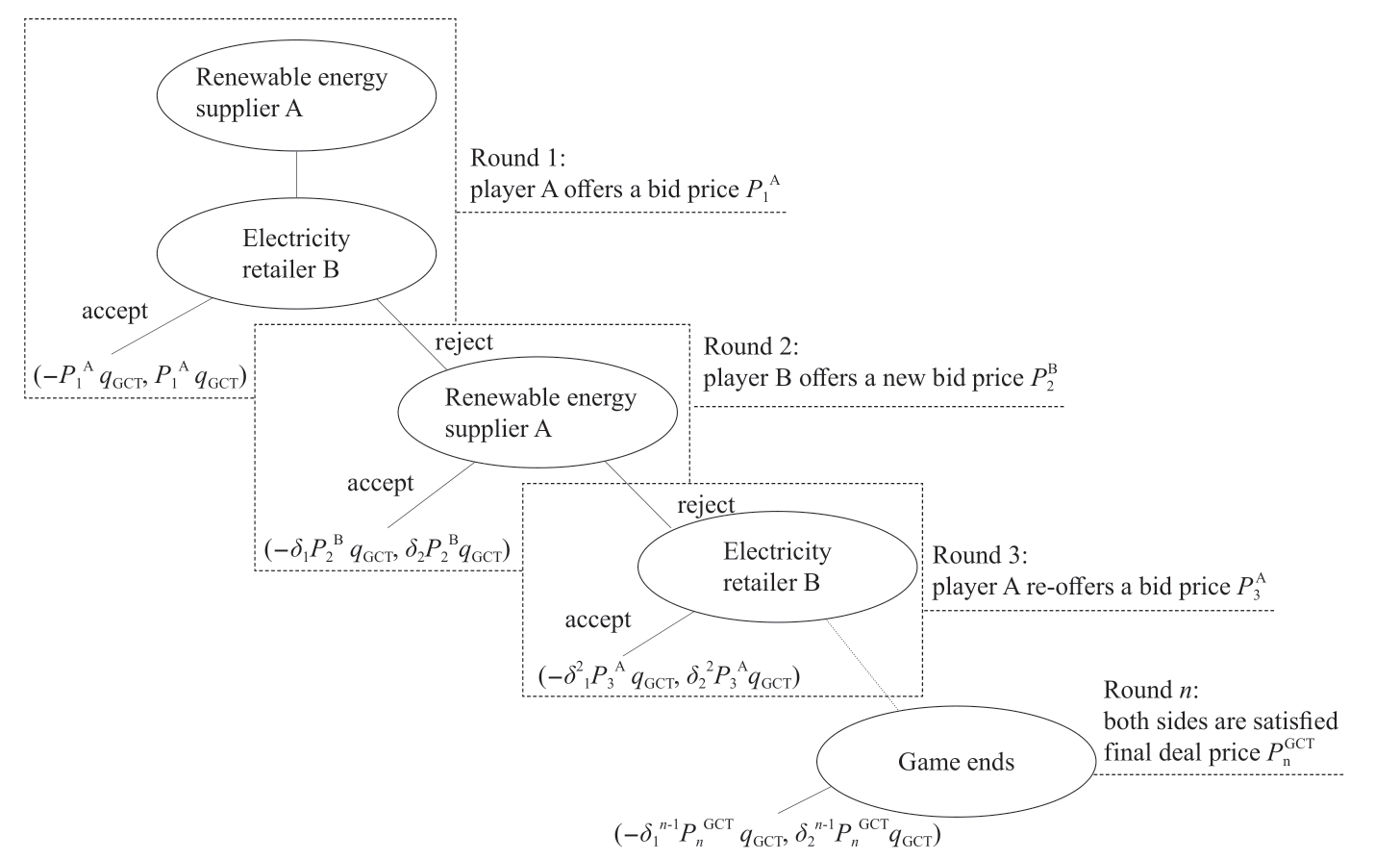
Fig.2 GCT price negotiation process
where,DTG indicates the total amount of CO2 emissions from thermal power plants,η indicates the carbon emission factor in kg/MWh.In this study,a ladder pricing model[30]is used for more stringent control of carbon emissions.
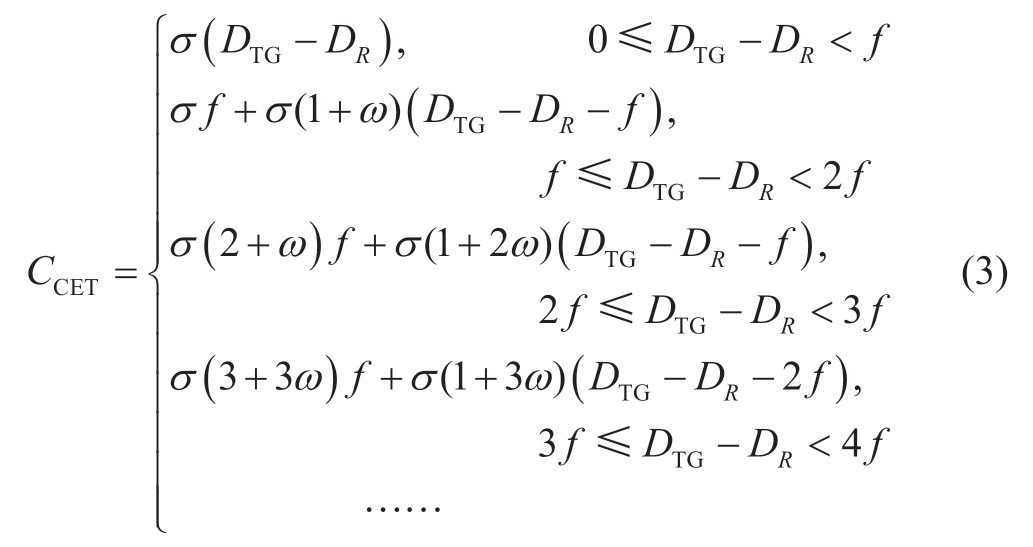
where CCET indicates the CO2 emission cost of the thermal power plant and σ symbolizes the CET price.DR indicates the initial carbon emissions allocated for free.f denotes the carbon emission interval step size of the ladder pricing model.Lastly,ω symbolizes the penalty coefficient.
2.3 Power generation cost model
A quadratic relationship can be fitted between the fuel cost of the power producer and the amount of electricity generated[31].

where Ci denotes the generation cost of power station i,qi denotes the generation capacity of power station i,ai and bi denote the variable cost coefficients of power station,and ci is a constant term,which indicates fixed cost.
3 Multi-market bidding optimization model
In this section,a multi-market bidding optimization model is constructed based on the previously proposed green certificate- carbon emission rights- electricity multi-market trading process.The model is based on the evolutionary game theory,which considers the dynamic bidding strategy behavior between green electricity suppliers and traditional thermal electricity suppliers under imperfect rationality,and considers individual profit optimization as the objective function.
3.1 Optimization objective
The focus of this study is on the impact of introducing carbon credits and green certificates trading on the electricity market in the context of a new electricity market with a high proportion of renewable energy.To this,three game parties are introduced,namely thermal,wind,and photovoltaic power generators,to compete for prices.The profit Ei of generator i is given by the following equation:

where pMCP indicates the marginal clearing tariff in the electricity market.Considering the exclusivity of the participating market game subjects,each power producer pursues their own profit maximization,and the optimization objective of the upper model is:

where qL represents the total load demand,pi(qi)represents the bidding strategy curve of generators i.
In this paper,considering the imperfectly rational bidding behavior of participants in China’s electricity market in decision making,the evolutionary game model[32]is used to simulate the market competition process among power generators,which is more in line with the actual state of the market compared with the traditional game.As listed in Table 1,the three participating generators submit two bidding strategies Hi and Li(i=1,2,3)to the power trading center.x,y,and z respectively denote the probability that PV generator(PG),wind generator(WG),and thermal power generator(TG)choose the high price strategy Hi.
Table 1 2 × 2 × 2 asymmetric evolutionary game payoff matrix
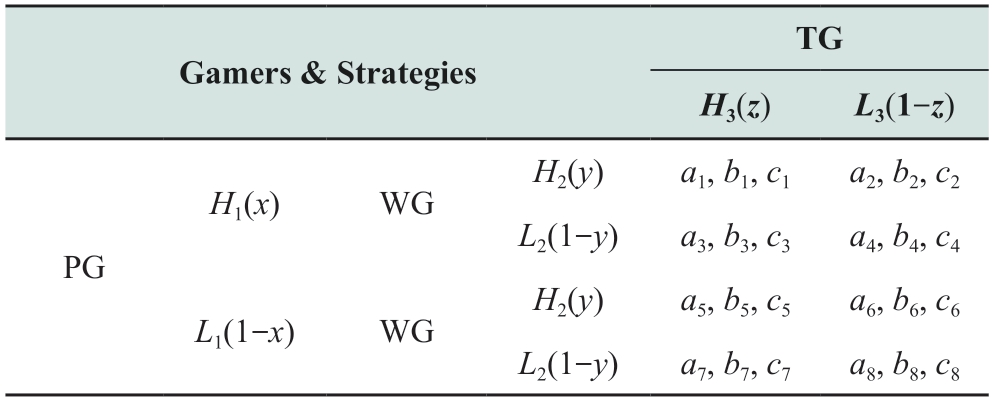
The expected returns of the game participants PG,WG,and TG are calculated as follows:
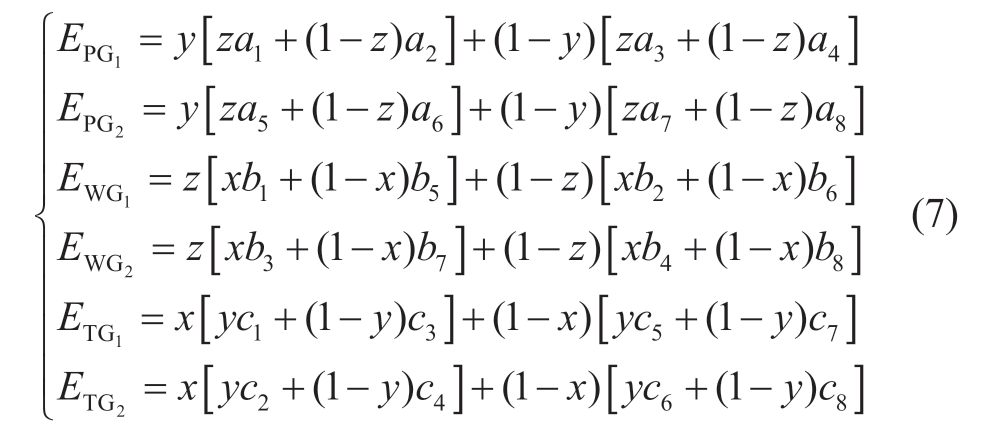
where represent the expected returns of PG,WG,and TG under the high price strategy.Meanwhile,
represent the expected returns of PG,WG,and TG under the low price strategy.Their average expected returns
are calculated as follows:
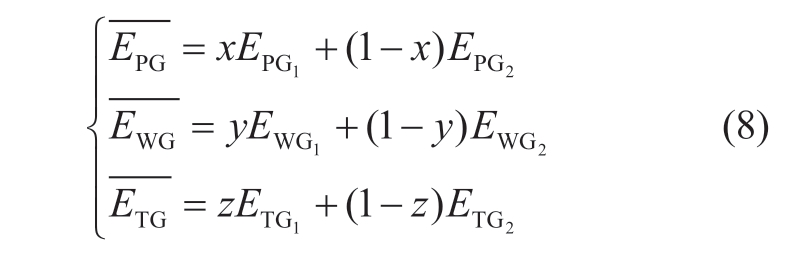
Furthermore,the asymmetric evolutionary game replication dynamic equations of the PV power generation company PG,wind power generation company WG,and thermal power generation company TG are obtained as follows:
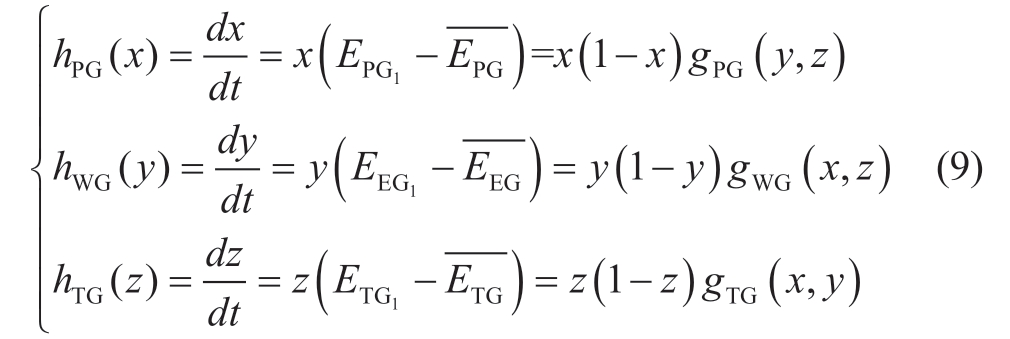
When is satisfied,the market is considered to have reached the equilibrium stabilization point,and the participating generators have made the best choice of bidding strategy.
3.2 Constraints
1)Supply and demand balance constraints

where PTG(t),PPG(t),and PWG(t)represent the output power of thermal,photovoltaic,and wind units at time t. PL(t)represents the total load and power losses of power grid at time t.
2)Unit output constraints

where PTG,max and PTG,min represent the upper and lower output limits of the thermal units.
3)Unit output power ramp rate constraint
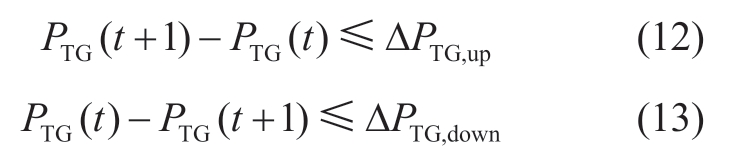
where ΔPTG,up and ΔPTG,down represent the upper and lower ramp rate limits of the thermal units,respectively.
4)New energy output constraints

where represent the predicted output of wind and photovoltaic power at time t,respectively.
5)GCT price negotiation range constraints

whererepresents the bidding price of game participant at negotiation round n.
represent the lower and upper limits of GCT bidding prices.
3.3 Model solving algorithms
As mentioned above,PG,WG,and TG entail the three independent groups,and each group has two quotation strategy curves for low price and high price.Each individual in the population can make an offer for the strategy choice,and the initial probability of choosing both low and high price strategies is set to 0.5,that is,the number of individuals choosing both strategies in the population is assumed to be the same at the beginning of the game.A composite differential evolutionary game algorithm based on multi-swarm evolution is constructed by combining the differential evolutionary algorithm[33]and evolutionary game idea.Based on this algorithm,the optimization process of power suppliers’ evolution game bidding for power generation is realized.Table 2 outlines the specific steps of solving the model.The specific evaluation steps are as follows:
Table 2 Composite differential evolutionary game algorithm
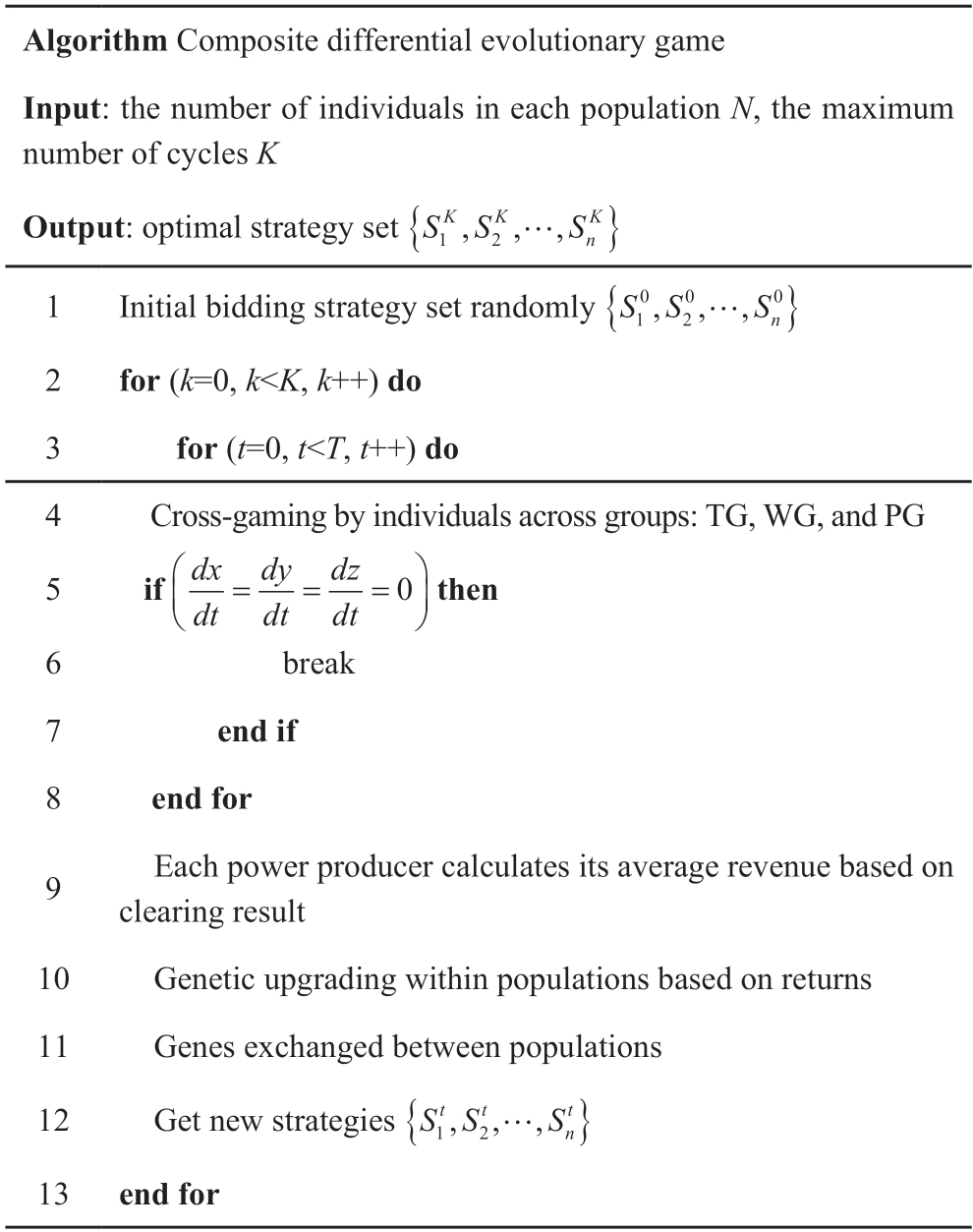
1)Initialization:Suppose there are N individuals in each population and the initial bidding strategy set is The cycle starts with k=0,and the maximum number of rounds is set to K.
2)Cross-bargaining game:Individuals are randomly selected from each group for cross-games.Each individual can play multiple games,and each game is independent of each other and does not affect each other.The electricity retailer acquires the power in the order of low to high price until the load demand is satisfied.Upon determining the power clearing results,each game participant will calculate the surplus or shortage of carbon emission rights and the number of green certificates under the supervision of the operation management organization,and complete the clearing and trading of the GCT and CET market based on the models presented in Section 2,and finally the total cost and profit values of PG,WG,and TG are obtained.
3)Evolution:When the game reaches N times,each power supplier calculates their average revenue based on the clearing results of electricity,GCT,and CET.Individuals within populations are ranked according to their average returns,and disadvantaged individuals can change their bidding strategy.The new strategy set is Upon reaching the maximum number of rounds K,the optimal solution is obtained.
4 Case study
To verify the effectiveness of the bidding optimization model based on the evolutionary game proposed in this study,three participants,PG,WG,and TG,are set in the context of high proportion of new energy grid-connected consumption.Following that,their game processes under different bidding strategies are used for simulating and testing.The renewable energy supplier can obtain one green certificate for producing one MW of electricity and the price of GCT is between 60 and 80 RMB.The initial carbon emission quota is 40% and the price of CET σis 90 RMB.The ladder pricing model step size is 10% and the penalty coefficient(ω)is 0.1.The parameters of each generator are listed in Table 3,and the high and low offer strategy curves are illustrated in Fig.3.
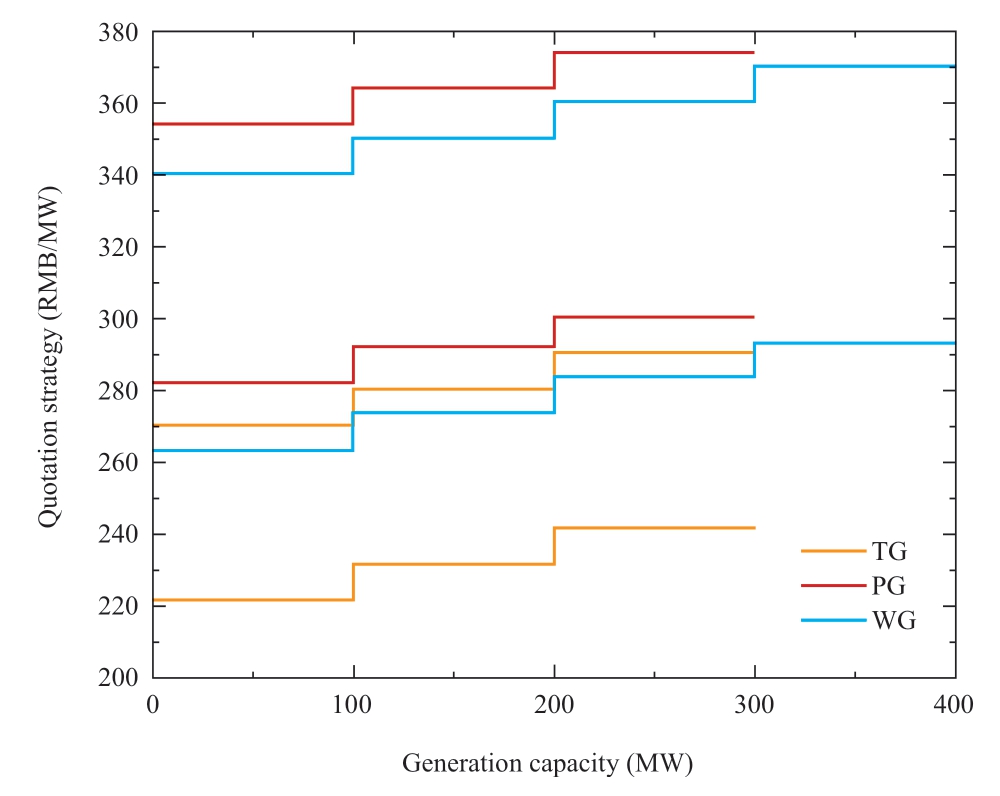
Fig.3 Generators quotation strategy curve
Table 3 Parameters of each unit
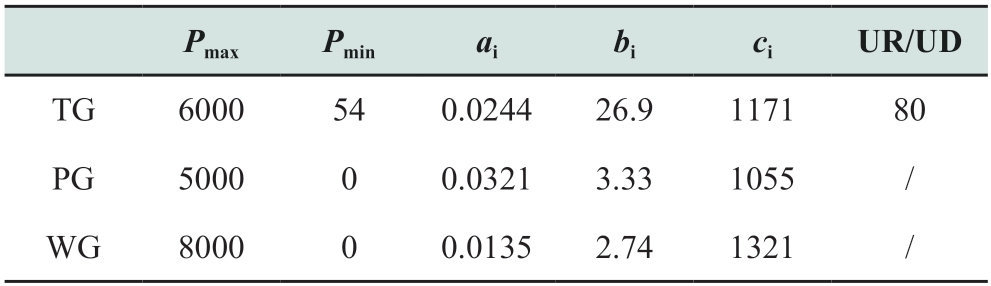
The parameters in the composite differential evolutionary game algorithm used in the study are set as follows:the maximum number of iterative rounds of the population is 160,the number of individuals of all three populations is 10,the crossover probability is 0.5,and the variance scaling factor is 0.1.The simulation period is taken as 1 d,where one day is divided into 24 periods,and each hour is utilized as a game bidding period.
Figure 4 shows the trend graph of the probability of choosing the high-bid strategy for the generators under the evolutionary game model.With the iteration of game rounds,the three parties continuously summarize the game gains and rival bidding strategies of the previous round,and adjust and find the optimal bidding strategy suitable for them through the evolution of learning.At the beginning of the market competition,most of the three types of generators choose a low-price strategy,primarily because their objective is to capture higher market share and increase the amount of electricity cleared through malicious price competition.As the market develops and grows,the generators realize that low price competition will affect the overall clearing price,which is detrimental to the interests of all parties,so the generators will tacitly choose to jointly raise prices to increase revenue.
As indicated in Fig.4,during the initial bidding stage,PG,which has the highest cost and pricing,is obviously at a price disadvantage.When PG finds that it cannot increase its market share by lowering its pricing,PG quickly switches and chooses the high price strategy to increase its profit by raising the market-clearing tariff.The probability of PG choosing the high price strategy rises the fastest among the three game subjects and reaches equilibrium at the earliest,and after fifty iterations,PG keeps choosing the high price strategy steadily.The probability of PG choosing the high price strategy is the fastest among the three players and reaches equilibrium at the earliest.By contrast,TG,which has the lowest cost and pricing,chooses both high and lowprice strategies without affecting its clearing share,so the process of evolution to stability is the slowest.
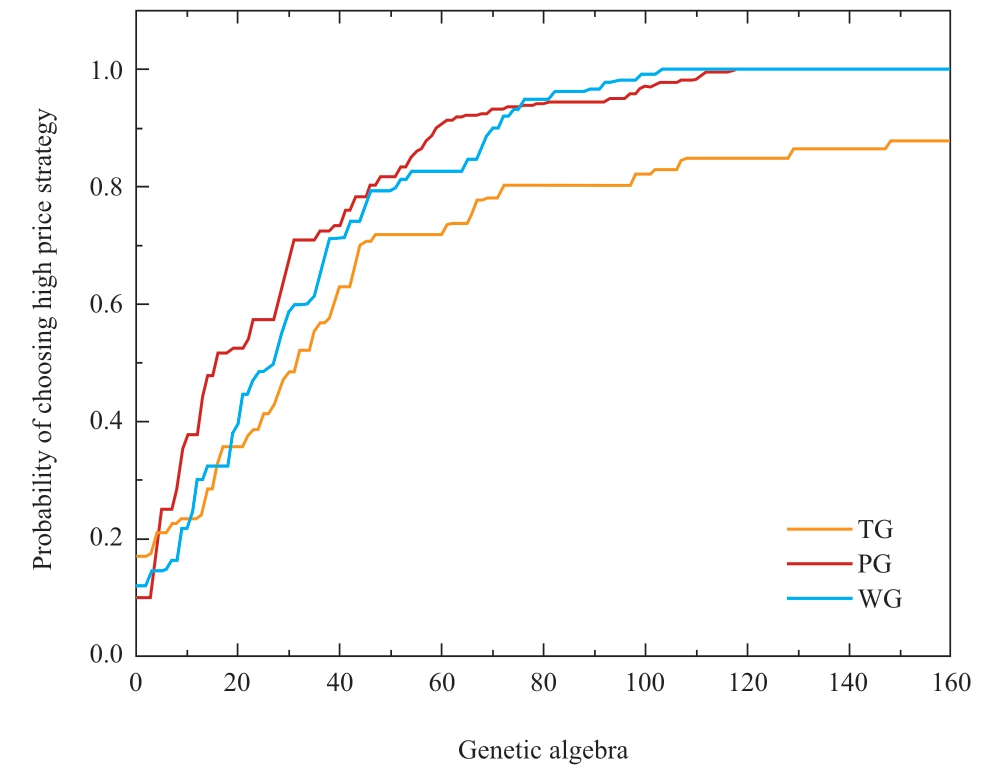
Fig.4 Changing process of individual strategy choices
Figures.5-7 illustrate the total operating profit of PG,WG,and TG under three different scenarios.In Fig.5,only the power market is considered,where each power supplier only has electricity cost.In Fig.6,the joint market is considered;however,the green certificate and carbon emission right are set as fixed-price.Moreover,in Fig.7,the joint market is considered with the dynamic cost model proposed in this paper.Table 4 presents the total profits of each power supplier under three different scenarios.In Fig.5,when only the cost of generation is considered,TG exhibits the best advantage in the clearing owing to its lower cost and lower offer.
Table 4 Total profits of each power supplier under three different market scenarios(×106 RMB)
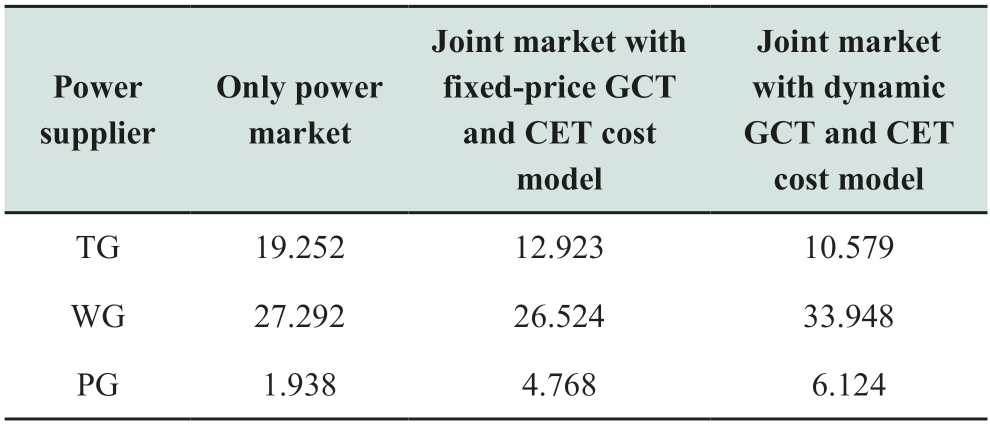
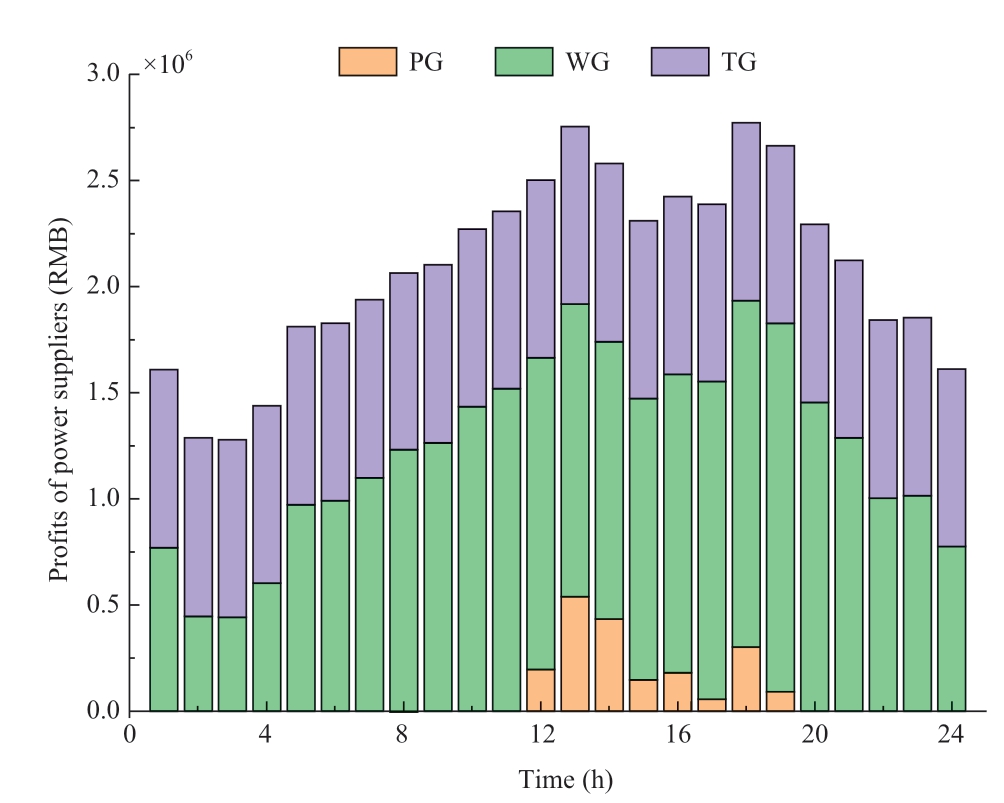
Fig.5 Profits considering only the electricity market
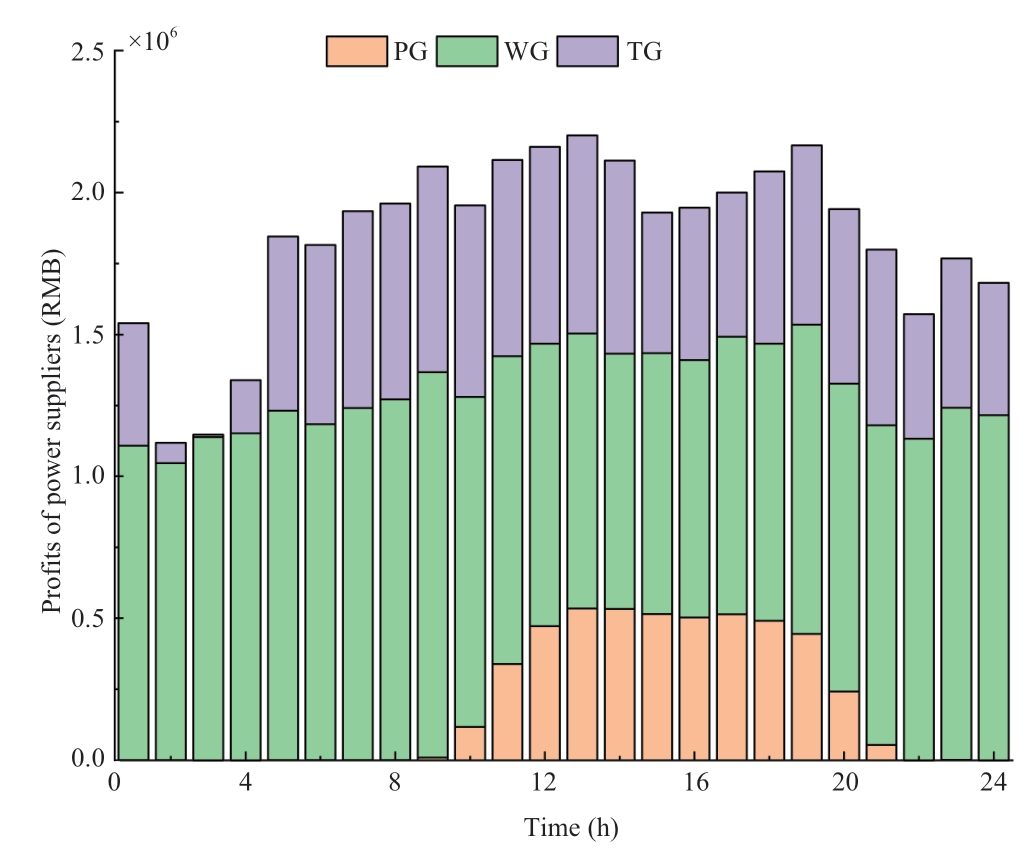
Fig.6 Profits considering the joint market with fixed-price GCT and CET cost model
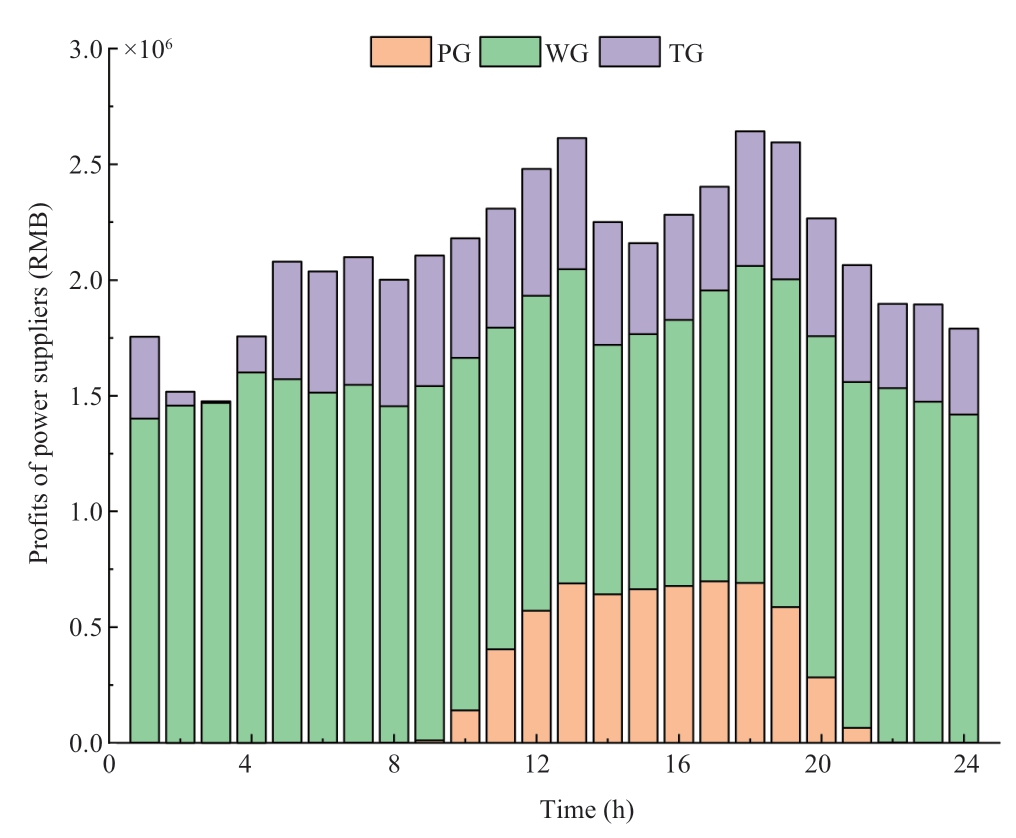
Fig.7 Profits considering the joint market with dynamic GCT and CET cost model
In Fig.6,following the implementation of the traditional fixed-price GCT and CET,the profits of PG and WG can be subsidized by green certificates.Meanwhile,the overemission thermal power plants will pay the cost of carbon emission rights,and the advantages of renewable energy are initially highlighted.
And in Fig.7,the bilateral bargaining GCT model can provide a more satisfactory price for both parties,and the green certificate quota bearers need to pay a penalty if they fail to complete the quota,and the penalty amount is much higher than the range of GCT price.Therefore,the bilateral bargaining mechanism has effectively improved the enthusiasm of trading entities,fully reflecting the fact that renewable energy generation has environmental value in addition to electricity value,which further promotes the consumption of renewable energy.The ladder pricing of CET is a further tightening of the policy based on the traditional fixed carbon price,and the control of carbon emission is stricter,creating favorable market conditions for the consumption of renewable energy.
Figure 8 presents the profits of the power suppliers for different GCT price ranges.The results reveal that as the GCT price increases,the profits of PV and wind power suppliers will gradually increase.Additionally,the total cost of electricity retailers purchasing CCT will increase.This indicates that the high cost of GCT place renewable power in a priority position in the competition of power generation rights.Moreover,when the price of GCT is higher than 80 RMB,the profits of electricity suppliers basically stop increasing.Therefore,the government needs to set an appropriate GCT price range that can promote renewable energy consumption while avoiding additional financial pressure on electricity retailers.
Figure 9 presents the average profit of thermal power suppliers under different initial carbon emission quotas.It is evident that if the initial carbon emission quota is higher than 70%,there is no significant punishment on overemission thermal power supplier and the CET cost is equal to zero.Furthermore,when the initial carbon emission quota falls below 35%,it will lead to a sharp reduction in profits of TG.Overall,the CET mechanism has a limiting effect on carbon emissions.However,in the actual market,it is still necessary to consider the benefits of traditional thermal power suppliers and choose suitable initial quotas during the energy transformation process.
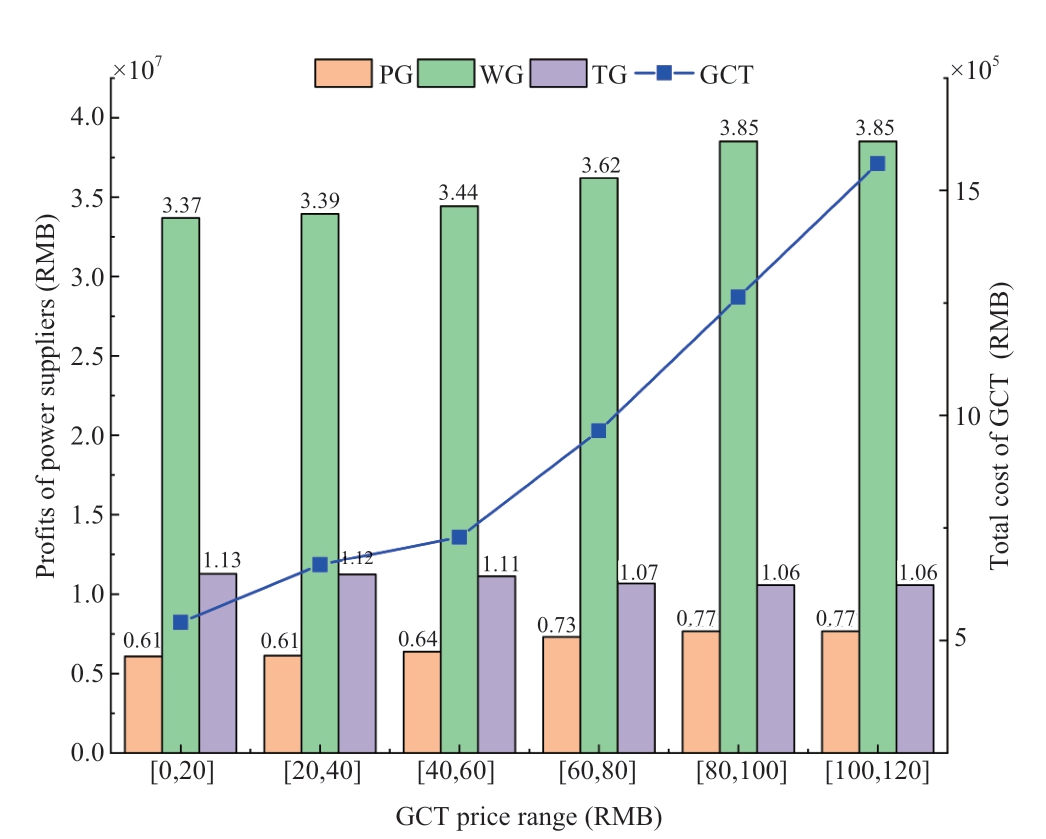
Fig.8 Profits of power suppliers for different GCT price ranges
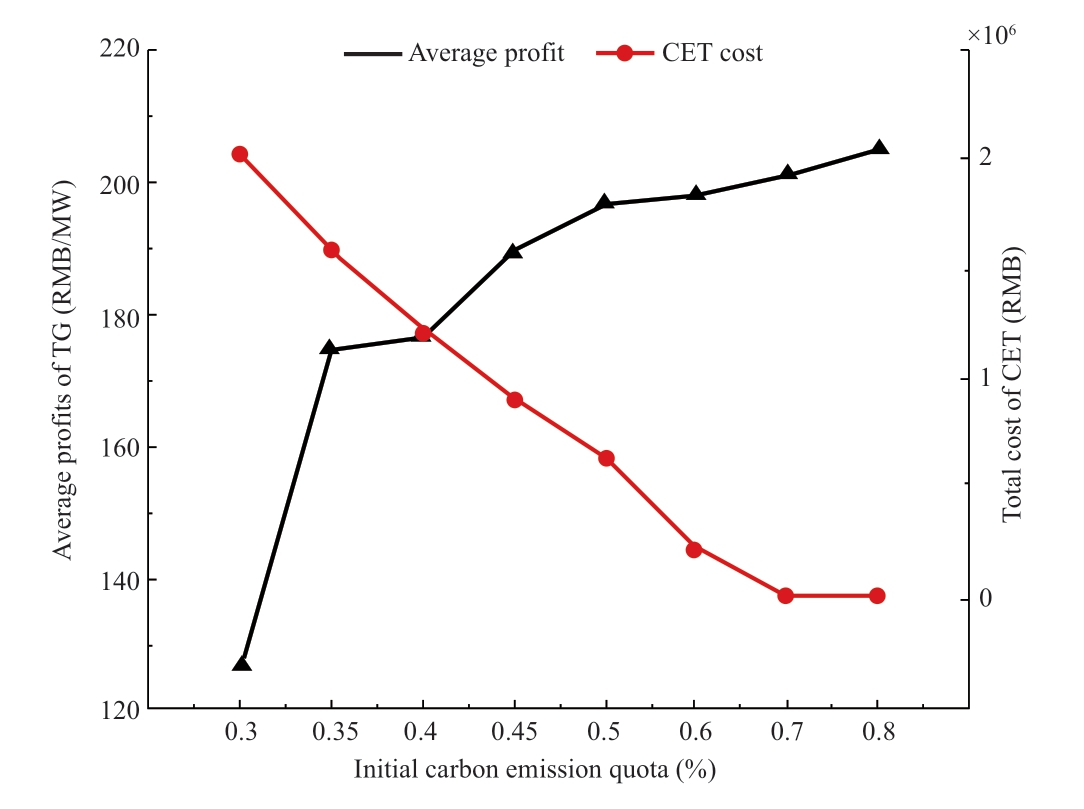
Fig.9 Profits of TG for different initial carbon emission quota
5 Conclusion
Against the background that the development of renewable energy is becoming increasingly mature and the proportion of renewable energy generation in the electricity market is gradually increasing,a joint trading mechanism of green certificate- carbon emission right- electricity was developed to alleviate the financial burden on the government.The proposed mechanism was introduced to a thermal-wind-photovoltaic power system and the evolutionary game theory was applied to analyze the bidding behaviors of the power suppliers.
The conclusions of this study are as follows:
1)The GCT bilateral bargaining model and CET ladder pricing model were introduced into the electricity and energy market trading to expand the scale of voluntary green certificate transactions through bilateral bargaining and promote energy conservation and emission reduction of fossil energy power generators by imposing appropriate penalties.Accordingly,market-oriented means were used to alleviate the financial pressure on government subsidies for renewable energy.
2)A three-party asymmetric evolutionary game bidding optimization model for thermal power,wind power,and photovoltaic power suppliers was constructed by considering the existence of non-perfect rational behavior of power generators.
3)The results of the case reveal that compared with the traditional fixed green certificate price and fixed carbon price,the GCT bilateral bargaining system and the CET ladder pricing mechanism can effectively stimulate the trading potential of the green certificate and carbon rights market.Moreover,the revenue of renewable energy generators can be significantly improved,which has a good promotional effect for renewable energy consumption,and the carbon emission penalty can effectively avoid excessive carbon emissions from thermal power plants.
Appendix A
Table A1 The nomenclature of variables
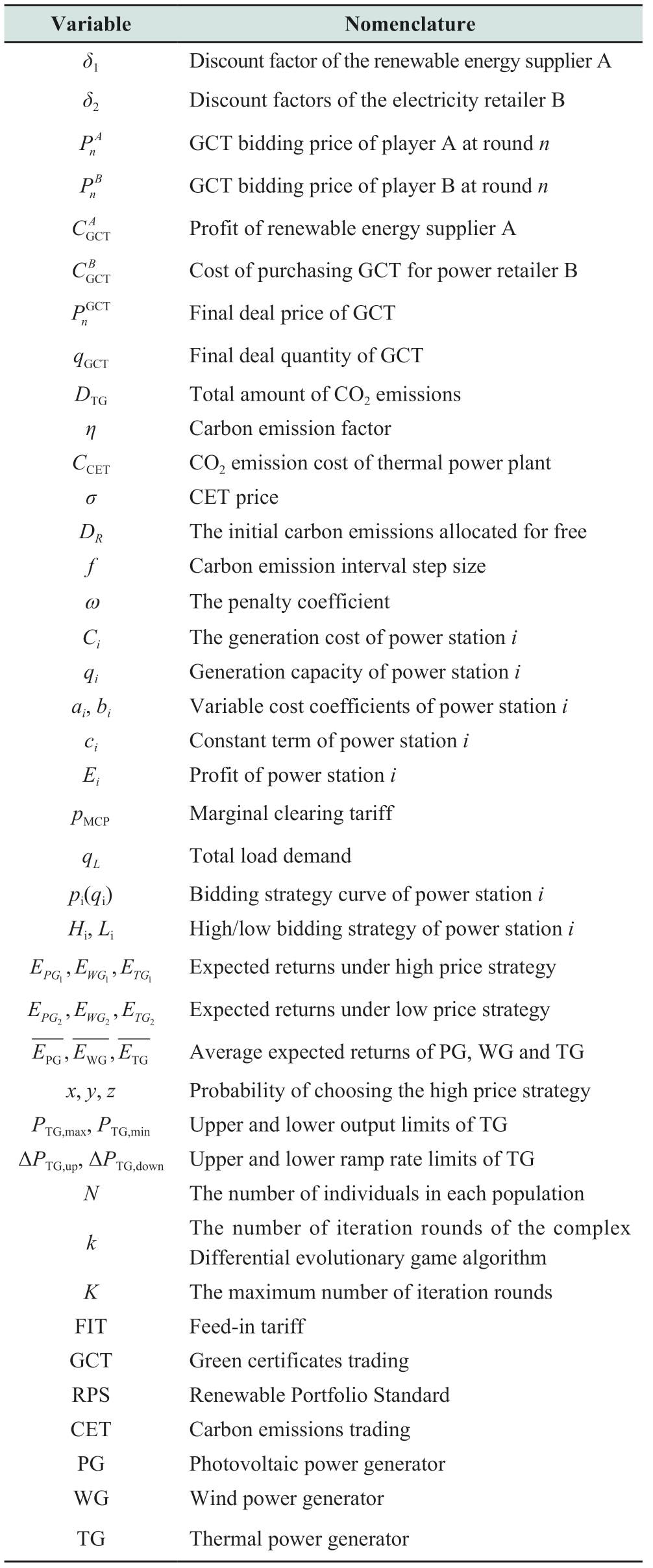
Acknowledgements
This work was supported by the National Key R&D Program of China(2017YFB0902200).
Declaration of Competing Interest
We declare that we have no conflict of interest.
References
[1]Li Y,Chen K,Zheng N,et al.(2021)Strategy research on accelerating green and low-carbon development under the guidance of carbon peak and carbon neutral targets.Proceedings of the 5th International Conference on Environmental and Energy Engineering,Yangzhou,China,Mar 2021
[2]Wang H,Zhang N,Du E,et al.(2022)A comprehensive review for wind,solar,and electrical load forecasting methods.Global Energy Interconnection,5(1):9-30
[3]Lin B,Wu W(2017)Cost of long-distance electricity transmission in China.Energy Policy,109:132-140
[4]Liu S,Zhao H,Lin J,et al.(2018)Curtailment of renewable energy in Northwest China and market-based solutions.Energy Policy,123:494-502
[5]Xie Z,Zhang Y(2016)Realization of full acquisition of renewable energy based on quota system.Proceedings of 2016 International Conference on Modeling,Simulation and Optimization Technologies and Applications,Xiamen,China,Dec 2016
[6]Zhu C,Fan R,Lin J(2020)The impact of renewable portfolio standard on retail electricity market:a system dynamics model of tripartite evolutionary game.Energy Policy,136:111072
[7]Dong J,Jiang Y,Liu D,et al.(2022)Promoting dynamic pricing implementation considering policy incentives and electricity retailers’ behaviors:An evolutionary game model based on prospect theory.Energy Policy,167:113059
[8]Cheng L,Chen Y,Liu G(2022)2PnS-EG:A general twopopulation n-strategy evolutionary game for strategic long-term bidding in a deregulated market under different market clearing mechanisms.International Journal of Electrical Power &Energy Systems,142:108182
[9]Han O,Ding T,Bai L,et al.(2021)Evolutionary game based demand response bidding strategy for end-users using Q-learning and compound differential evolution.IEEE Transactions on Cloud Computing,10(1):97-110
[10]Cheng L,Yu T(2020)Decision-making behavior investigation for general multi-strategy evolutionary games in the spontaneous formation of long-term bidding equilibria of a power generation market.Proceedings of the CSEE,40(21):6936-6956
[11]Yin L,Li S,Gao F(2020)Equilibrium stability of asymmetric evolutionary games of multi-agent systems with multiple groups in open electricity market.IEEE Access,8:28970-28978
[12]Zhao X,Ren L,Zhang Y(2018)Evolutionary game analysis on the behavior strategies of power producers in renewable portfolio standard.Energy,162:505-516
[13]Peng C,Qian K,Yan J(2019)A bidding strategy based on differential evolution game for generation side in power grid integrated with renewable energy resources.Power System Technology,43(6):2002-2010
[14]Shen N,Deng R,Liao H,et al.(2020)Mapping renewable energy subsidy policy research published from 1997 to 2018:A scient metric review.Utilities Policy,64:101055
[15]Karakosta O,Petropoulou D(2022)The EU electricity market:renewables targets,Tradable Green Certificates and electricity trade.Energy Economics,111:106034
[16]Wang H,Wang L,Shi S,et al.(2019)Research on US green certificate trading mechanism experience and domestic implementation prospects.IOP Conference Series:Earth and Environmental Science,237:052035
[17]Atici C(2022)Reconciling the flexibility mechanisms of climate policies towards the inclusiveness of developing countries:commitments and prospects.Environment,Development and Sustainability,24:9048-9067
[18]Feng T,Li R,Zhang H,et al.(2021)Induction mechanism and optimization of tradable green certificates and carbon emission trading acting on electricity market in China.Resources,Conservation &Recycling,169:105487
[19]Song X,Han J,Shan Y,et al.(2020)Efficiency of tradable green certificate markets in China.Journal of Cleaner Production,264:121518
[20]Wu Q,Xi M(2021)Equilibrium analysis of trans-provincial electricity market considering tradable green certificates.Modern Electric Power,38(4):434-441
[21]Dong F,Shi L(2019)Design and simulation of renewable portfolio standard and tradable green certificate mechanism.Automation of Electric Power Systems,43(12):113-121
[22]Zhang W,Li J,Li G,et al.(2020)Emission reduction effect and carbon market efficiency of carbon emissions trading policy in China.Energy,196:117117
[23]Gao Y,Li M,Xue J,et al.(2020)Evaluation of effectiveness of China’s carbon emissions trading scheme in carbon mitigation.Energy Economics,90:104872
[24]Wang R,Wen X,Wang X,et al.(2022)Low carbon optimal operation of integrated energy system based on carbon capture technology,LCA carbon emissions and ladder-type carbon trading.Applied Energy,311:118664
[25]Tan Q,Ding Y,Ye Q,et al.(2019)Optimization and evaluation of a dispatch model for an integrated wind-photovoltaic-thermal power system based on dynamic carbon emissions trading.Applied Energy,253:113598
[26]Luo Z,Qin J,Liang J,et al.(2021)Day-ahead optimal scheduling of integrated energy system with carbon-green certificate coordinated trading mechanism.Electric Power Automation Equipment,41(9):248-255
[27]Sun Y,Ling J,Qin Y,et al.(2018)A bidding optimization method for renewable energy cross-regional transaction under green certificate trading mechanism.Renewable Energy Resources,36(6):942-948
[28]Chang X,Li Y,Wang S(2018)Optimal decisions of carbon emission reduction and production pricing under benchmarking free allocation of carbon permits.Journal of Beijing Institute of Technology(Social Science Edition),20(6):1-8
[29]Duman P,Trockel W(2016)On non-cooperative foundation and implementation of the Nash solution in subgame perfect equilibrium via Rubinstein’s game.Journal of Mechanism and Institution Design,1(1):83-106
[30]Chen Z,Hu Z,Weng C,et al.(2021)Multi-stage planning of park-level integrated energy system based on ladder-type carbon trading mechanism.Electric Power Automation Equipment,41(9):148-155
[31]Yin G,Zhou L,Duan M,et al.(2018)Impacts of carbon pricing and renewable electricity subsidy on direct cost of electricity generation:a case study of China’s provincial power sector.Journal of Cleaner Production,205:375-387
[32]Zeng K,Cheng L,Liu J,et al.(2020)Two-population asymmetric evolutionary game dynamics-based decision-making behavior analysis for a supply-side electric power bidding market.E3S Web of Conferences,194:03009
[33]Yang Y,Liu J,Tan S,et al.(2019)A multi-objective differential evolutionary algorithm for constrained multi-objective optimization problems with low feasible ratio.Applied Soft Computing Journal,80:42-56
Received:19 April 2022/Accepted:15 September 2022/Published:25 February 2023
Bingtuan Gao
gaobingtuan@seu.edu.cn
Ran Wang
220202974 @seu.edu.cn
Yanhe Li
lyhe0416@163.com
2096-5117/© 2023 Global Energy Interconnection Development and Cooperation Organization.Production and hosting by Elsevier B.V.on behalf of KeAi Communications Co.,Ltd.This is an open access article under the CC BY-NC-ND license(http://creativecommons.org/licenses/by-nc-nd/4.0/).
Biographies
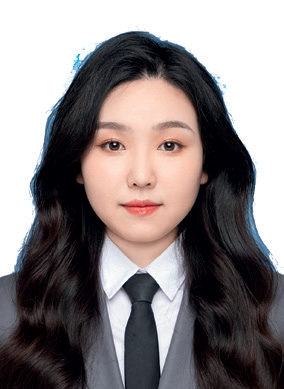
Ran Wang received a Bachelor degree at Dalian University of Technology,Dalian,China,in 2020 and is currently pursuing a Master degree at Southeast University,Nanjing,China.Her research interests include renewable energy subsidy policies and power market optimization scheduling.
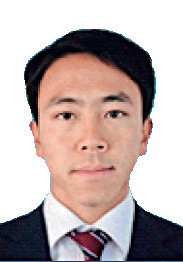
Yanhe Li received Bachelor and Master degrees in electrical engineering at Tianjin University,Tianjin,China,in 2005 and 2008,respectively.He is employed as a senior engineer in State Grid Qinghai Electric Power Company,Xining,China.He has been pursuing his Ph.D.degree in Southeast University since 2020.His research interests include the operation and control of power grids.
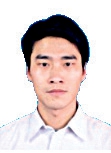
Bingtuan Gao received a Bachelor degree in electrical engineering,Master degree in control theory and control engineering,and Ph.D.degree in power electronics and electrical drives,all from Harbin Institute of Technology,Harbin,China,in 2002,2004,and 2007,respectively.From 2008 to 2010,he was a Post Doctor with the Department of Electrical and Computer Engineering,Michigan State University,East Lansing,USA.He is currently a Professor with the School of Electrical Engineering,Southeast University,Nanjing,China.His research interests include robotics,renewable energy generation,and demand-side management.
(Editor Yajun Zou)